DELTA: Dense Efficient Long-range 3D Tracking for any video
Tuan Duc Ngo, Peiye Zhuang, Chuang Gan, Evangelos Kalogerakis, Sergey Tulyakov, Hsin-Ying Lee, Chaoyang Wang
2024-11-01
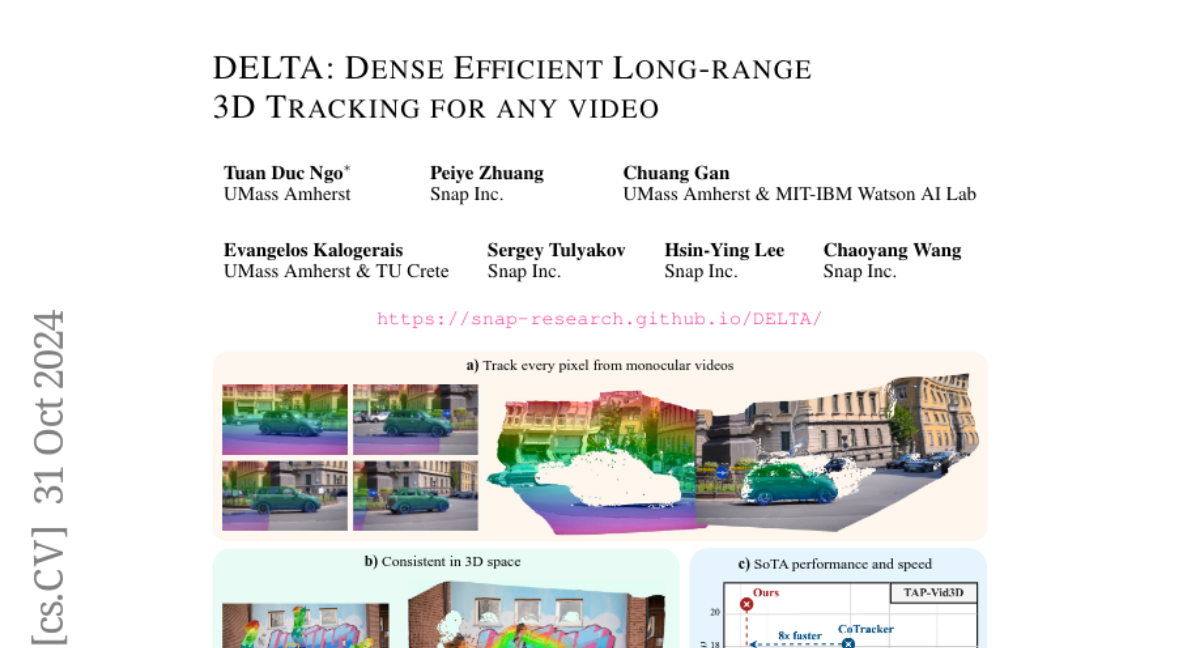
Summary
This paper presents DELTA, a new method for tracking dense 3D motion in videos. It focuses on accurately following every pixel's movement in 3D space over long video sequences, addressing challenges in precision and efficiency.
What's the problem?
Tracking the movement of objects in videos is difficult, especially when trying to achieve high accuracy over many frames. Existing methods either run too slowly or only track a few points instead of every pixel, making it hard to capture detailed motion.
What's the solution?
DELTA uses a unique approach that combines global and local attention mechanisms for tracking at a lower resolution before refining the results to high resolution with a transformer-based upsampler. This method allows it to track every pixel efficiently and accurately, achieving speeds over eight times faster than previous methods while maintaining high accuracy.
Why it matters?
This research is significant because it improves the ability to track fine movements in videos, which can be crucial for applications like robotics, animation, and surveillance. By providing a faster and more accurate tracking solution, DELTA opens up new possibilities for analyzing motion in various fields.
Abstract
Tracking dense 3D motion from monocular videos remains challenging, particularly when aiming for pixel-level precision over long sequences. We introduce \Approach, a novel method that efficiently tracks every pixel in 3D space, enabling accurate motion estimation across entire videos. Our approach leverages a joint global-local attention mechanism for reduced-resolution tracking, followed by a transformer-based upsampler to achieve high-resolution predictions. Unlike existing methods, which are limited by computational inefficiency or sparse tracking, \Approach delivers dense 3D tracking at scale, running over 8x faster than previous methods while achieving state-of-the-art accuracy. Furthermore, we explore the impact of depth representation on tracking performance and identify log-depth as the optimal choice. Extensive experiments demonstrate the superiority of \Approach on multiple benchmarks, achieving new state-of-the-art results in both 2D and 3D dense tracking tasks. Our method provides a robust solution for applications requiring fine-grained, long-term motion tracking in 3D space.