Denoising Reuse: Exploiting Inter-frame Motion Consistency for Efficient Video Latent Generation
Chenyu Wang, Shuo Yan, Yixuan Chen, Yujiang Wang, Mingzhi Dong, Xiaochen Yang, Dongsheng Li, Robert P. Dick, Qin Lv, Fan Yang, Tun Lu, Ning Gu, Li Shang
2024-09-20
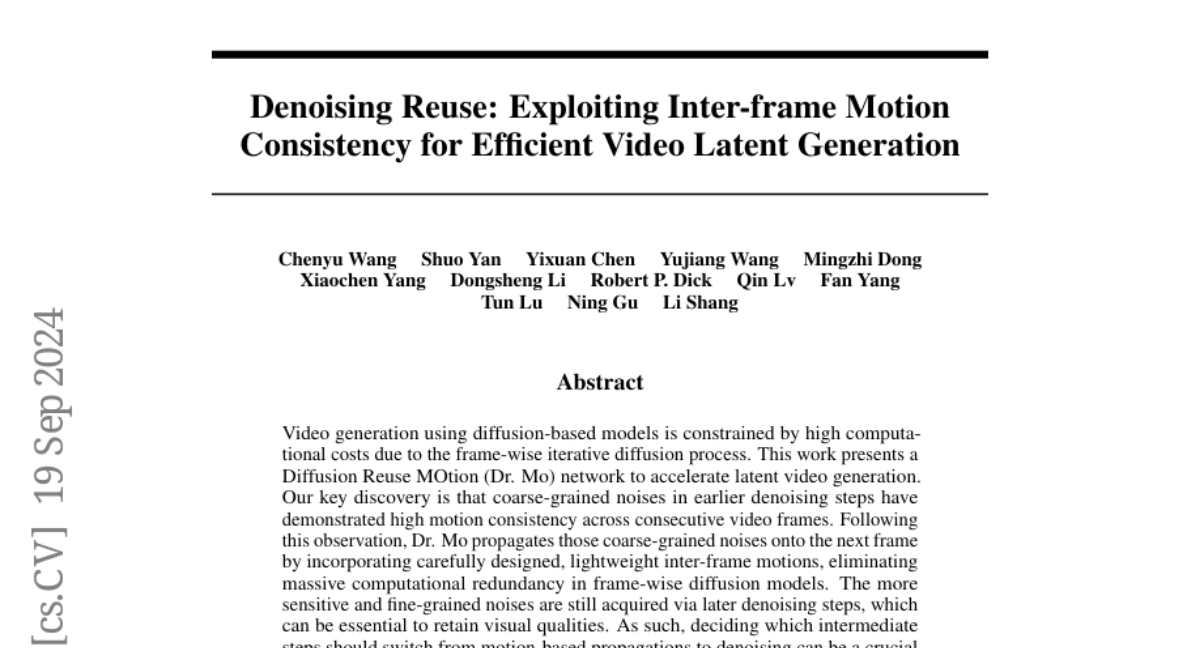
Summary
This paper introduces a new method called Denoising Reuse, which improves the efficiency of generating videos using a technique called diffusion. The method focuses on how to effectively use noise patterns from previous video frames to speed up the video creation process.
What's the problem?
Generating videos with diffusion models can be very slow and expensive in terms of computing power because it requires processing each frame one at a time. This iterative process can take a long time, making it hard to create videos quickly, especially for complex scenes.
What's the solution?
The researchers developed a network called Dr. Mo that takes advantage of the consistent noise patterns found in earlier frames. Instead of starting from scratch for each frame, Dr. Mo reuses these noise patterns and applies them to the next frame using lightweight techniques that reduce unnecessary calculations. They also created a smart system called the Denoising Step Selector (DSS) that decides when to switch from using these motion-based methods to more detailed denoising steps, balancing speed and quality.
Why it matters?
This research is important because it makes video generation faster and more efficient, which is crucial for applications like video games, movies, and virtual reality. By improving how videos are created, it allows for better quality content to be produced in less time, ultimately enhancing the user experience.
Abstract
Video generation using diffusion-based models is constrained by high computational costs due to the frame-wise iterative diffusion process. This work presents a Diffusion Reuse MOtion (Dr. Mo) network to accelerate latent video generation. Our key discovery is that coarse-grained noises in earlier denoising steps have demonstrated high motion consistency across consecutive video frames. Following this observation, Dr. Mo propagates those coarse-grained noises onto the next frame by incorporating carefully designed, lightweight inter-frame motions, eliminating massive computational redundancy in frame-wise diffusion models. The more sensitive and fine-grained noises are still acquired via later denoising steps, which can be essential to retain visual qualities. As such, deciding which intermediate steps should switch from motion-based propagations to denoising can be a crucial problem and a key tradeoff between efficiency and quality. Dr. Mo employs a meta-network named Denoising Step Selector (DSS) to dynamically determine desirable intermediate steps across video frames. Extensive evaluations on video generation and editing tasks have shown that Dr. Mo can substantially accelerate diffusion models in video tasks with improved visual qualities.