Depth Anything V2
Lihe Yang, Bingyi Kang, Zilong Huang, Zhen Zhao, Xiaogang Xu, Jiashi Feng, Hengshuang Zhao
2024-06-14
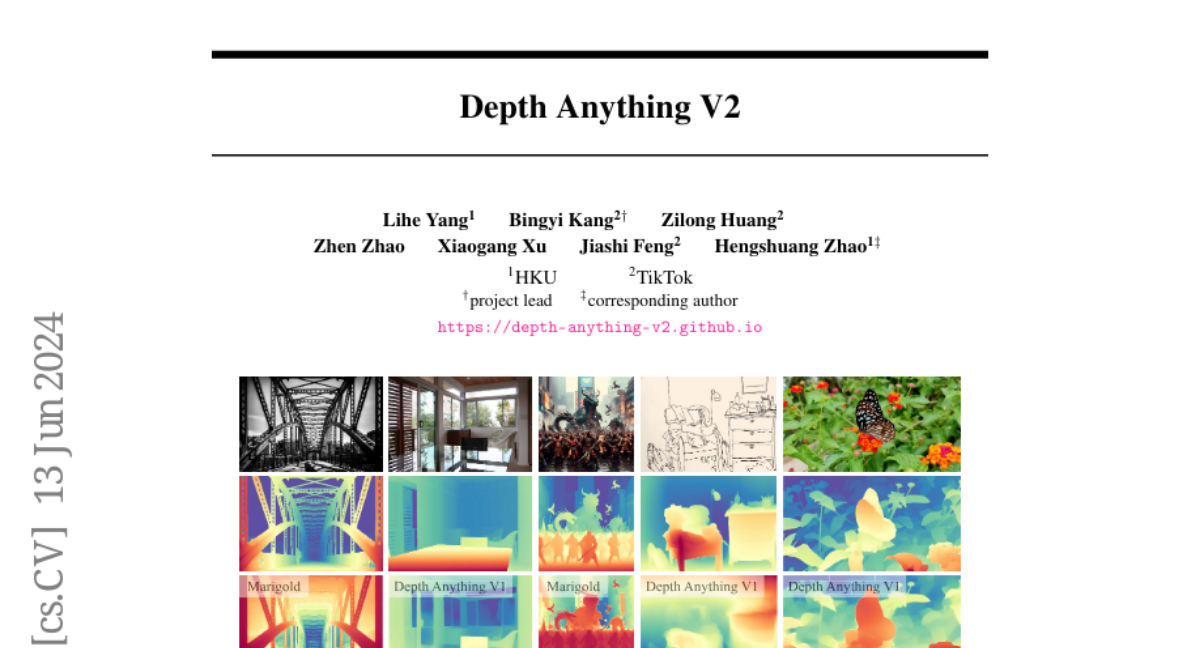
Summary
This paper introduces Depth Anything V2, an advanced model designed to estimate depth from images taken with a single camera. The goal is to improve how accurately and efficiently the model can determine how far away objects are in a picture.
What's the problem?
Estimating depth from a single image is challenging because it involves figuring out how far away different objects are based on visual clues. Traditional models often rely on labeled real images, which can be limited and noisy. This makes it hard for the models to learn effectively and can lead to inaccuracies in depth estimation.
What's the solution?
Depth Anything V2 improves upon the previous version by implementing three main strategies: first, it replaces real labeled images with synthetic ones that are easier to use; second, it increases the size and capability of the teacher model that guides the learning process; and third, it uses a large number of pseudo-labeled images to help train student models. These changes allow Depth Anything V2 to produce more accurate depth predictions while being significantly faster than other models, achieving over 10 times the efficiency of similar models.
Why it matters?
This research is important because it enhances the ability of AI systems to understand depth from single images, which is crucial in many applications like self-driving cars, robotics, and augmented reality. By improving the accuracy and speed of depth estimation, Depth Anything V2 can lead to better performance in real-world scenarios where understanding distances is essential.
Abstract
This work presents Depth Anything V2. Without pursuing fancy techniques, we aim to reveal crucial findings to pave the way towards building a powerful monocular depth estimation model. Notably, compared with V1, this version produces much finer and more robust depth predictions through three key practices: 1) replacing all labeled real images with synthetic images, 2) scaling up the capacity of our teacher model, and 3) teaching student models via the bridge of large-scale pseudo-labeled real images. Compared with the latest models built on Stable Diffusion, our models are significantly more efficient (more than 10x faster) and more accurate. We offer models of different scales (ranging from 25M to 1.3B params) to support extensive scenarios. Benefiting from their strong generalization capability, we fine-tune them with metric depth labels to obtain our metric depth models. In addition to our models, considering the limited diversity and frequent noise in current test sets, we construct a versatile evaluation benchmark with precise annotations and diverse scenes to facilitate future research.