Depth Pro: Sharp Monocular Metric Depth in Less Than a Second
Aleksei Bochkovskii, Amaël Delaunoy, Hugo Germain, Marcel Santos, Yichao Zhou, Stephan R. Richter, Vladlen Koltun
2024-10-04
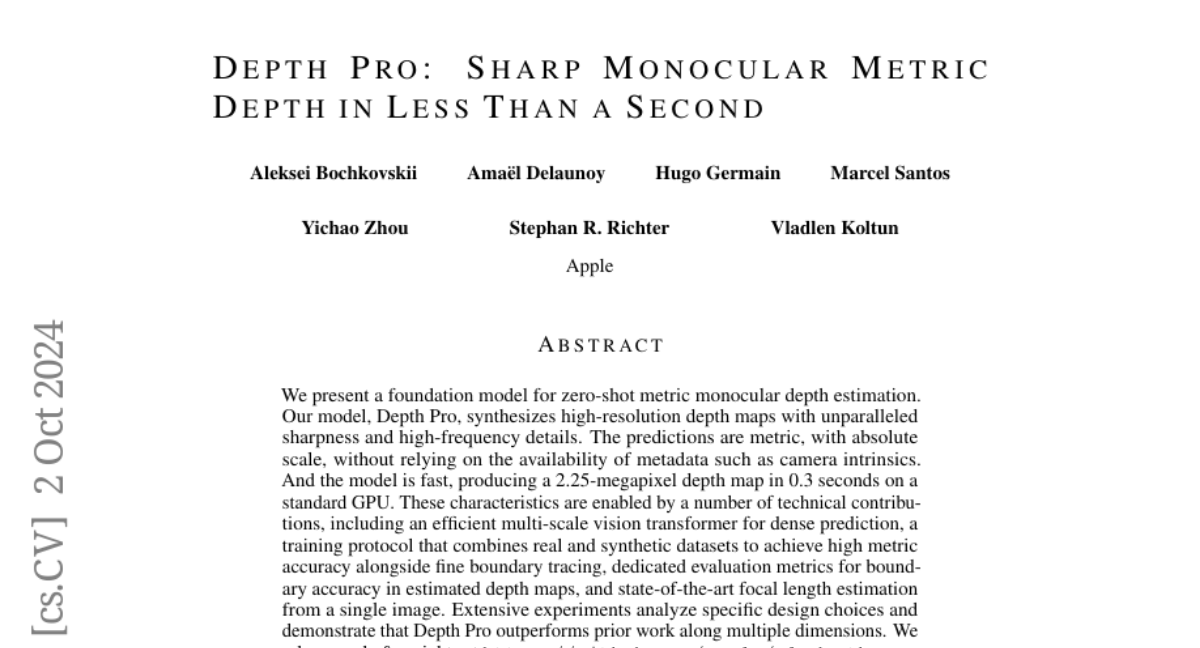
Summary
This paper introduces Depth Pro, a new model that can quickly create detailed depth maps from single images, allowing for accurate distance measurements without needing extra camera information.
What's the problem?
Creating depth maps (which show how far away objects are in an image) from a single camera view can be challenging, especially when trying to achieve high accuracy and detail. Traditional methods often require additional information about the camera settings, which isn't always available. Additionally, many existing models take too long to produce these depth maps or don't provide the sharp details needed for applications like image editing or virtual reality.
What's the solution?
Depth Pro solves these problems by using advanced techniques to generate high-resolution depth maps quickly—producing a detailed 2.25-megapixel depth map in just 0.3 seconds. It does this without relying on extra camera data, using a special training method that combines real and synthetic images to improve accuracy. The model also includes new ways to measure how well it captures fine details, such as edges and boundaries of objects, ensuring that the depth maps are precise and useful.
Why it matters?
This research is important because it makes it easier and faster to create accurate depth maps from single images, which can be used in various fields like photography, video games, and augmented reality. By improving how we estimate depth, Depth Pro can enhance the quality of visual content and make advanced imaging techniques more accessible.
Abstract
We present a foundation model for zero-shot metric monocular depth estimation. Our model, Depth Pro, synthesizes high-resolution depth maps with unparalleled sharpness and high-frequency details. The predictions are metric, with absolute scale, without relying on the availability of metadata such as camera intrinsics. And the model is fast, producing a 2.25-megapixel depth map in 0.3 seconds on a standard GPU. These characteristics are enabled by a number of technical contributions, including an efficient multi-scale vision transformer for dense prediction, a training protocol that combines real and synthetic datasets to achieve high metric accuracy alongside fine boundary tracing, dedicated evaluation metrics for boundary accuracy in estimated depth maps, and state-of-the-art focal length estimation from a single image. Extensive experiments analyze specific design choices and demonstrate that Depth Pro outperforms prior work along multiple dimensions. We release code and weights at https://github.com/apple/ml-depth-pro