Designing a Dashboard for Transparency and Control of Conversational AI
Yida Chen, Aoyu Wu, Trevor DePodesta, Catherine Yeh, Kenneth Li, Nicholas Castillo Marin, Oam Patel, Jan Riecke, Shivam Raval, Olivia Seow, Martin Wattenberg, Fernanda Viégas
2024-06-17
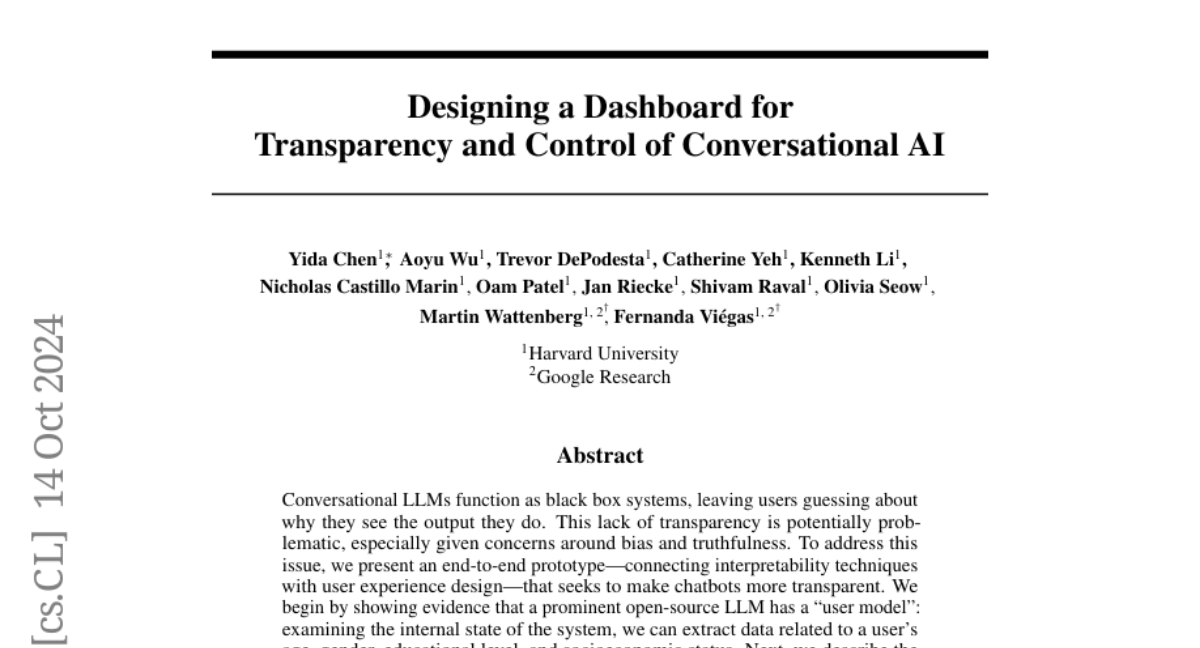
Summary
This paper presents a new system called TalkTuner, which is designed to make chatbots more transparent and give users control over how these AI systems understand them. It aims to help users understand why chatbots respond the way they do by showing them the internal workings of the AI.
What's the problem?
Many conversational AI systems, like chatbots, operate as 'black boxes,' meaning users can't see how they generate their responses. This lack of transparency can lead to confusion and concern about biases in the chatbot's answers, as users might not know why they receive certain responses or if those responses are fair and accurate.
What's the solution?
To tackle this issue, the authors developed a dashboard that displays a 'user model' for the chatbot. This model shows information about the user, such as their age, gender, education level, and socioeconomic status, which the chatbot uses to tailor its responses. The dashboard allows users to see this information in real-time and even modify it to see how it affects the chatbot's behavior. The authors conducted a study where users interacted with the system, and they found that participants appreciated being able to see and control these internal states, which helped them identify biased responses.
Why it matters?
This research is significant because it enhances user trust in AI systems by providing transparency about how chatbots operate. By allowing users to understand and control the factors influencing chatbot responses, TalkTuner can help reduce biases and improve user experience. This work also opens up new avenues for future research in both design and machine learning, highlighting the importance of user-centered approaches in AI development.
Abstract
Conversational LLMs function as black box systems, leaving users guessing about why they see the output they do. This lack of transparency is potentially problematic, especially given concerns around bias and truthfulness. To address this issue, we present an end-to-end prototype-connecting interpretability techniques with user experience design-that seeks to make chatbots more transparent. We begin by showing evidence that a prominent open-source LLM has a "user model": examining the internal state of the system, we can extract data related to a user's age, gender, educational level, and socioeconomic status. Next, we describe the design of a dashboard that accompanies the chatbot interface, displaying this user model in real time. The dashboard can also be used to control the user model and the system's behavior. Finally, we discuss a study in which users conversed with the instrumented system. Our results suggest that users appreciate seeing internal states, which helped them expose biased behavior and increased their sense of control. Participants also made valuable suggestions that point to future directions for both design and machine learning research. The project page and video demo of our TalkTuner system are available at https://bit.ly/talktuner-project-page