DiffusionDrive: Truncated Diffusion Model for End-to-End Autonomous Driving
Bencheng Liao, Shaoyu Chen, Haoran Yin, Bo Jiang, Cheng Wang, Sixu Yan, Xinbang Zhang, Xiangyu Li, Ying Zhang, Qian Zhang, Xinggang Wang
2024-11-28
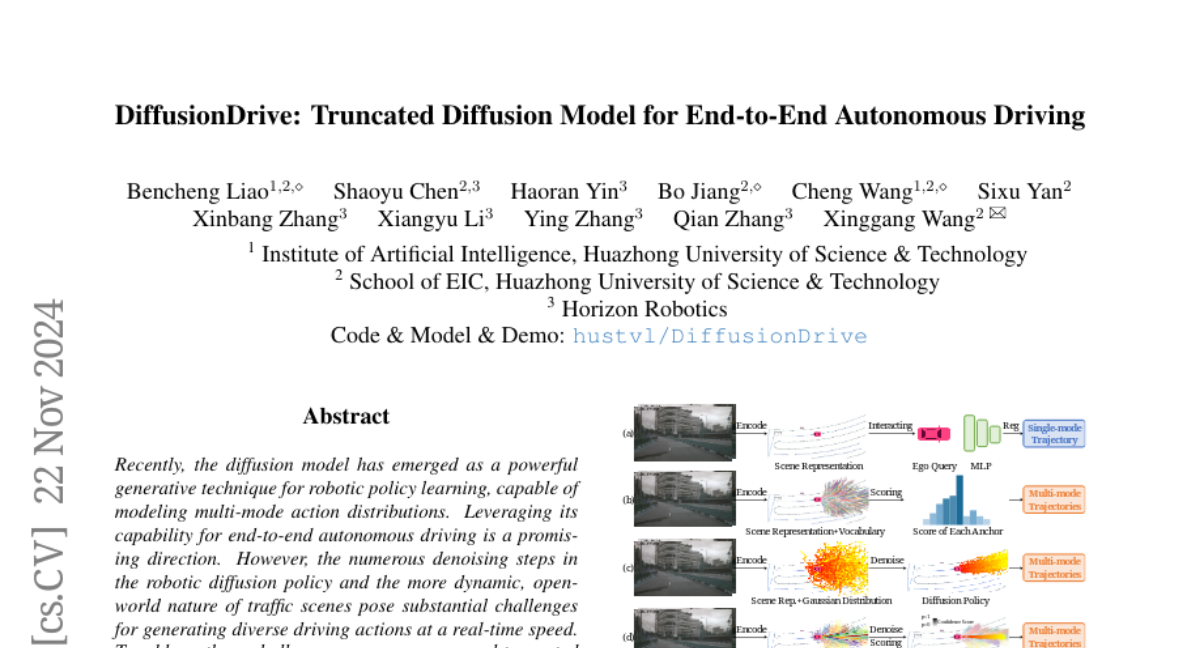
Summary
This paper introduces DiffusionDrive, a new method for autonomous driving that uses a truncated diffusion model to make real-time driving decisions more efficiently.
What's the problem?
Traditional methods for autonomous driving often require many complex steps to decide how to drive, which can slow down the process and make it harder to react quickly in dynamic traffic situations. This can lead to challenges in generating diverse and effective driving actions in real-time.
What's the solution?
The authors developed DiffusionDrive, which simplifies the decision-making process by using a truncated diffusion model. This model reduces the number of steps needed to generate driving actions while still maintaining high quality. It incorporates prior knowledge of driving behaviors and uses a special decoder that interacts with the environment, allowing it to make better decisions more quickly. The results show that DiffusionDrive is significantly faster and more effective than previous methods.
Why it matters?
This research is important because it enhances the capabilities of self-driving cars, making them safer and more efficient. By improving how these vehicles can make decisions in real-time, DiffusionDrive could lead to better performance in various driving scenarios, ultimately contributing to the development of reliable autonomous vehicles.
Abstract
Recently, the diffusion model has emerged as a powerful generative technique for robotic policy learning, capable of modeling multi-mode action distributions. Leveraging its capability for end-to-end autonomous driving is a promising direction. However, the numerous denoising steps in the robotic diffusion policy and the more dynamic, open-world nature of traffic scenes pose substantial challenges for generating diverse driving actions at a real-time speed. To address these challenges, we propose a novel truncated diffusion policy that incorporates prior multi-mode anchors and truncates the diffusion schedule, enabling the model to learn denoising from anchored Gaussian distribution to the multi-mode driving action distribution. Additionally, we design an efficient cascade diffusion decoder for enhanced interaction with conditional scene context. The proposed model, DiffusionDrive, demonstrates 10times reduction in denoising steps compared to vanilla diffusion policy, delivering superior diversity and quality in just 2 steps. On the planning-oriented NAVSIM dataset, with the aligned ResNet-34 backbone, DiffusionDrive achieves 88.1 PDMS without bells and whistles, setting a new record, while running at a real-time speed of 45 FPS on an NVIDIA 4090. Qualitative results on challenging scenarios further confirm that DiffusionDrive can robustly generate diverse plausible driving actions. Code and model will be available at https://github.com/hustvl/DiffusionDrive.