Discovering Preference Optimization Algorithms with and for Large Language Models
Chris Lu, Samuel Holt, Claudio Fanconi, Alex J. Chan, Jakob Foerster, Mihaela van der Schaar, Robert Tjarko Lange
2024-06-13
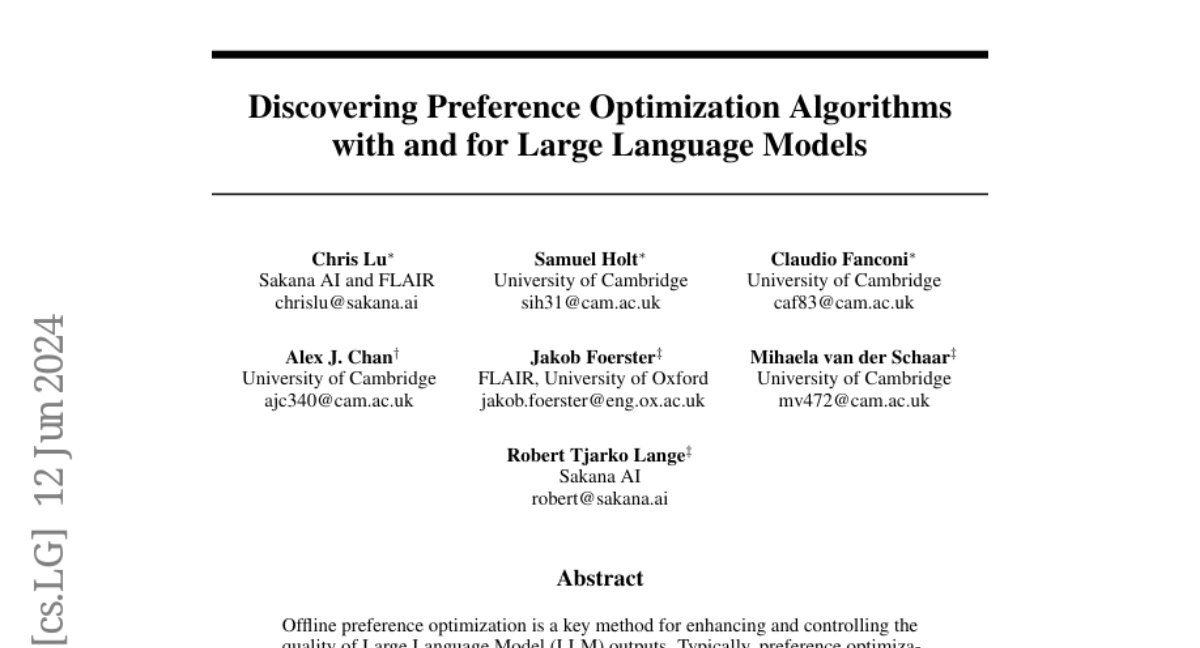
Summary
This paper discusses a new method for improving how large language models (LLMs) learn from and respond to human preferences. It introduces a technique called DiscoPOP, which automatically discovers better algorithms for optimizing LLM outputs without needing human input.
What's the problem?
Traditional methods for optimizing LLMs rely on human-designed loss functions, which are mathematical formulas used to measure how well the model is performing. These functions can be limited by human creativity, meaning that many potential options remain unexplored. This can hinder the model's ability to improve and adapt effectively to different tasks.
What's the solution?
To solve this problem, the authors developed a process where an LLM itself generates new loss functions based on previous performance data. By prompting the model iteratively, they were able to discover new algorithms that perform better than existing ones. The most successful of these is called Discovered Preference Optimization (DiscoPOP), which combines different types of loss functions to enhance performance. Experiments showed that DiscoPOP outperforms traditional methods in various tasks.
Why it matters?
This research is important because it shows that LLMs can not only be optimized but can also help create better optimization strategies on their own. This could lead to more efficient and effective AI systems that adapt more easily to human needs and preferences, ultimately improving the quality of AI-generated content.
Abstract
Offline preference optimization is a key method for enhancing and controlling the quality of Large Language Model (LLM) outputs. Typically, preference optimization is approached as an offline supervised learning task using manually-crafted convex loss functions. While these methods are based on theoretical insights, they are inherently constrained by human creativity, so the large search space of possible loss functions remains under explored. We address this by performing LLM-driven objective discovery to automatically discover new state-of-the-art preference optimization algorithms without (expert) human intervention. Specifically, we iteratively prompt an LLM to propose and implement new preference optimization loss functions based on previously-evaluated performance metrics. This process leads to the discovery of previously-unknown and performant preference optimization algorithms. The best performing of these we call Discovered Preference Optimization (DiscoPOP), a novel algorithm that adaptively blends logistic and exponential losses. Experiments demonstrate the state-of-the-art performance of DiscoPOP and its successful transfer to held-out tasks.