Distilled Decoding 1: One-step Sampling of Image Auto-regressive Models with Flow Matching
Enshu Liu, Xuefei Ning, Yu Wang, Zinan Lin
2024-12-24
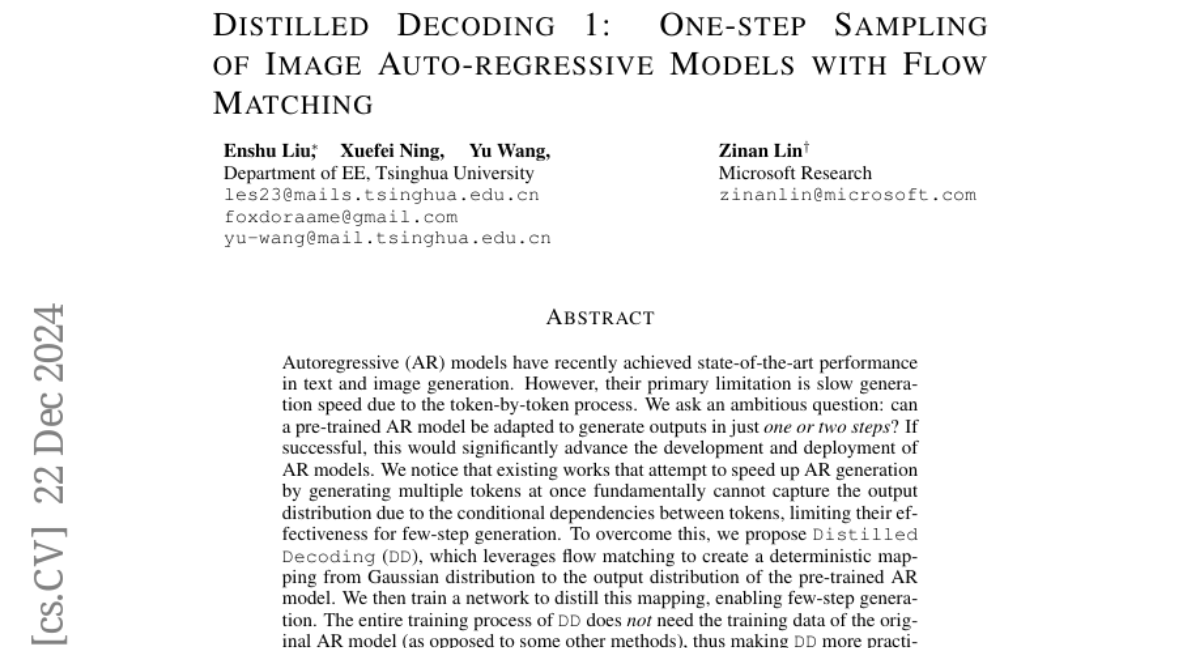
Summary
This paper talks about Distilled Decoding (DD), a new method that speeds up the process of generating images using autoregressive models, allowing them to create outputs in just one or two steps instead of many.
What's the problem?
Autoregressive models are great at generating images, but they usually do it slowly by predicting one part of the image at a time. This token-by-token approach can take a long time, especially for high-resolution images, making it inefficient for practical use. Existing methods that try to speed this up often fail to accurately capture the details needed for good image generation.
What's the solution?
To solve this problem, the authors propose Distilled Decoding, which uses a technique called flow matching to create a direct mapping from a simpler Gaussian distribution to the more complex output distribution of the autoregressive model. This allows the model to generate images in just one or two steps instead of many. The process doesn't require retraining on the original data, making it easier to implement. The results show that DD significantly speeds up image generation while maintaining quality.
Why it matters?
This research is important because it challenges the belief that autoregressive models are inherently slow. By demonstrating that these models can generate high-quality images quickly, DD opens up new possibilities for using autoregressive models in real-time applications, such as video games and interactive media, where speed is crucial.
Abstract
Autoregressive (AR) models have achieved state-of-the-art performance in text and image generation but suffer from slow generation due to the token-by-token process. We ask an ambitious question: can a pre-trained AR model be adapted to generate outputs in just one or two steps? If successful, this would significantly advance the development and deployment of AR models. We notice that existing works that try to speed up AR generation by generating multiple tokens at once fundamentally cannot capture the output distribution due to the conditional dependencies between tokens, limiting their effectiveness for few-step generation. To address this, we propose Distilled Decoding (DD), which uses flow matching to create a deterministic mapping from Gaussian distribution to the output distribution of the pre-trained AR model. We then train a network to distill this mapping, enabling few-step generation. DD doesn't need the training data of the original AR model, making it more practical.We evaluate DD on state-of-the-art image AR models and present promising results on ImageNet-256. For VAR, which requires 10-step generation, DD enables one-step generation (6.3times speed-up), with an acceptable increase in FID from 4.19 to 9.96. For LlamaGen, DD reduces generation from 256 steps to 1, achieving an 217.8times speed-up with a comparable FID increase from 4.11 to 11.35. In both cases, baseline methods completely fail with FID>100. DD also excels on text-to-image generation, reducing the generation from 256 steps to 2 for LlamaGen with minimal FID increase from 25.70 to 28.95. As the first work to demonstrate the possibility of one-step generation for image AR models, DD challenges the prevailing notion that AR models are inherently slow, and opens up new opportunities for efficient AR generation. The project website is at https://imagination-research.github.io/distilled-decoding.