Distribution Backtracking Builds A Faster Convergence Trajectory for One-step Diffusion Distillation
Shengyuan Zhang, Ling Yang, Zejian Li, An Zhao, Chenye Meng, Changyuan Yang, Guang Yang, Zhiyuan Yang, Lingyun Sun
2024-08-29
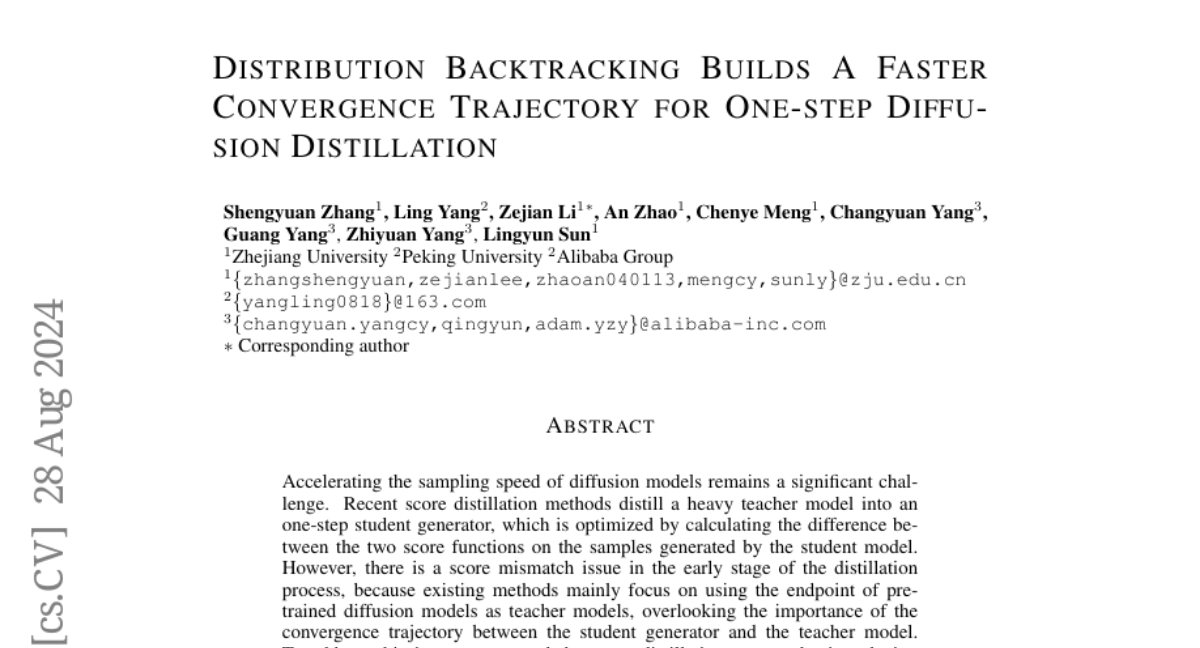
Summary
This paper discusses a new method called Distribution Backtracking Distillation (DisBack) that improves the speed and efficiency of training smaller models based on larger diffusion models.
What's the problem?
One of the main challenges in using diffusion models is that they can be slow to sample, which means generating outputs takes a long time. Existing methods for training smaller models from larger ones often focus only on the final results, ignoring how the model learns during the training process. This can lead to mismatches in performance early on, making it hard for smaller models to learn effectively.
What's the solution?
The authors introduce DisBack, which enhances the training process by considering the entire learning journey of the larger model, not just the end result. They use a two-step process: first, they record how the larger model's performance degrades as it trains, capturing its learning path. Then, they train the smaller model to follow this path, allowing it to learn more effectively from the larger model's experience. This approach leads to faster and better training outcomes compared to previous methods.
Why it matters?
This research is important because it helps create smaller models that can generate high-quality outputs quickly and efficiently. By improving how we train these models, DisBack can make advanced AI technologies more accessible and practical for various applications, such as image generation and natural language processing.
Abstract
Accelerating the sampling speed of diffusion models remains a significant challenge. Recent score distillation methods distill a heavy teacher model into an one-step student generator, which is optimized by calculating the difference between the two score functions on the samples generated by the student model. However, there is a score mismatch issue in the early stage of the distillation process, because existing methods mainly focus on using the endpoint of pre-trained diffusion models as teacher models, overlooking the importance of the convergence trajectory between the student generator and the teacher model. To address this issue, we extend the score distillation process by introducing the entire convergence trajectory of teacher models and propose Distribution Backtracking Distillation (DisBack) for distilling student generators. DisBask is composed of two stages: Degradation Recording and Distribution Backtracking. Degradation Recording is designed to obtain the convergence trajectory of teacher models, which records the degradation path from the trained teacher model to the untrained initial student generator. The degradation path implicitly represents the intermediate distributions of teacher models. Then Distribution Backtracking trains a student generator to backtrack the intermediate distributions for approximating the convergence trajectory of teacher models. Extensive experiments show that DisBack achieves faster and better convergence than the existing distillation method and accomplishes comparable generation performance. Notably, DisBack is easy to implement and can be generalized to existing distillation methods to boost performance. Our code is publicly available on https://github.com/SYZhang0805/DisBack.