Diversity Empowers Intelligence: Integrating Expertise of Software Engineering Agents
Kexun Zhang, Weiran Yao, Zuxin Liu, Yihao Feng, Zhiwei Liu, Rithesh Murthy, Tian Lan, Lei Li, Renze Lou, Jiacheng Xu, Bo Pang, Yingbo Zhou, Shelby Heinecke, Silvio Savarese, Huan Wang, Caiming Xiong
2024-08-14
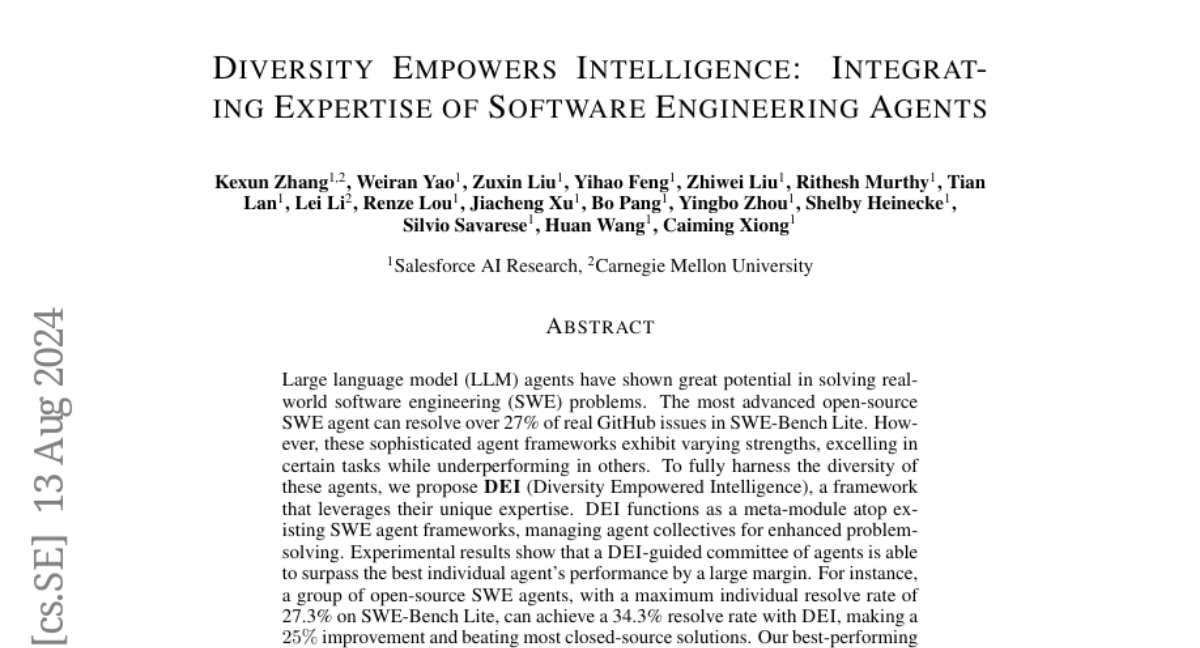
Summary
This paper discusses DEI (Diversity Empowered Intelligence), a framework that combines the strengths of various software engineering agents to solve real-world problems more effectively.
What's the problem?
Software engineering agents, which are AI systems designed to help with coding and software issues, often excel in specific tasks but struggle in others. This inconsistency limits their overall effectiveness in solving complex problems.
What's the solution?
The authors propose DEI as a meta-module that works on top of existing software engineering agents. It organizes a group of these agents to work together, allowing them to share their unique skills and knowledge. By doing this, a team of agents can perform better than any single agent alone. For example, when using DEI, a group of agents improved their problem-solving success rate from 27.3% to 34.3%, showing a significant performance boost.
Why it matters?
This research is important because it highlights the benefits of collaboration among AI systems. By leveraging the diverse capabilities of different agents, we can tackle more complex software engineering challenges, leading to better solutions and advancements in AI technology.
Abstract
Large language model (LLM) agents have shown great potential in solving real-world software engineering (SWE) problems. The most advanced open-source SWE agent can resolve over 27% of real GitHub issues in SWE-Bench Lite. However, these sophisticated agent frameworks exhibit varying strengths, excelling in certain tasks while underperforming in others. To fully harness the diversity of these agents, we propose DEI (Diversity Empowered Intelligence), a framework that leverages their unique expertise. DEI functions as a meta-module atop existing SWE agent frameworks, managing agent collectives for enhanced problem-solving. Experimental results show that a DEI-guided committee of agents is able to surpass the best individual agent's performance by a large margin. For instance, a group of open-source SWE agents, with a maximum individual resolve rate of 27.3% on SWE-Bench Lite, can achieve a 34.3% resolve rate with DEI, making a 25% improvement and beating most closed-source solutions. Our best-performing group excels with a 55% resolve rate, securing the highest ranking on SWE-Bench Lite. Our findings contribute to the growing body of research on collaborative AI systems and their potential to solve complex software engineering challenges.