DM-Codec: Distilling Multimodal Representations for Speech Tokenization
Md Mubtasim Ahasan, Md Fahim, Tasnim Mohiuddin, A K M Mahbubur Rahman, Aman Chadha, Tariq Iqbal, M Ashraful Amin, Md Mofijul Islam, Amin Ahsan Ali
2024-10-22
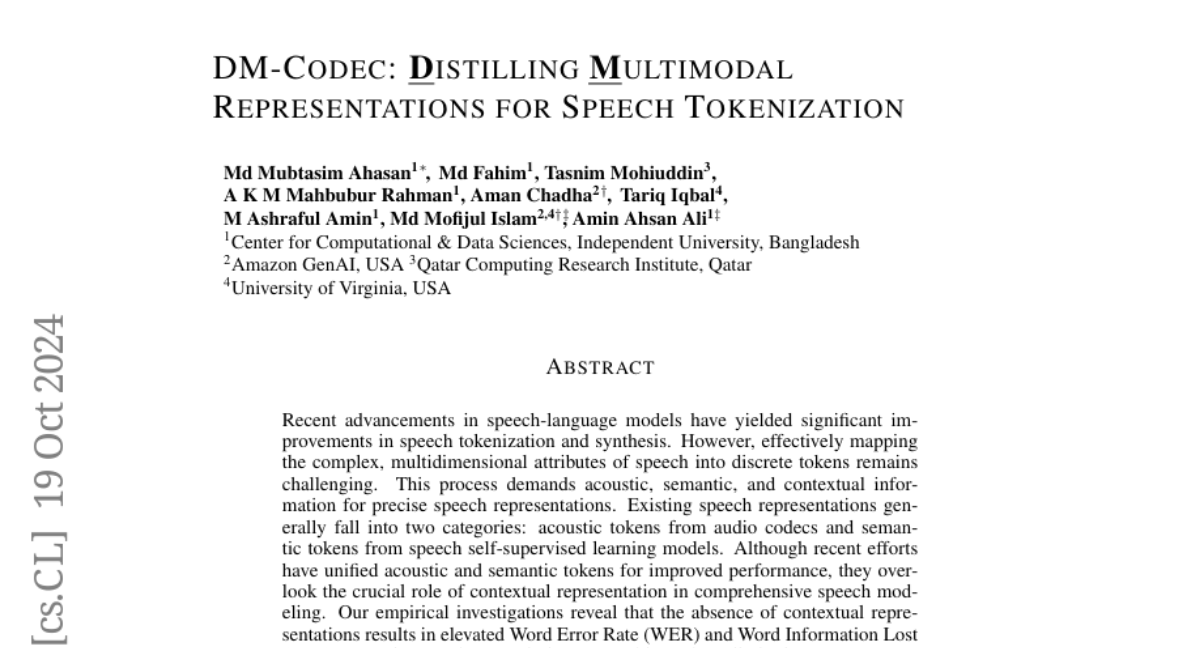
Summary
This paper introduces DM-Codec, a new method for converting speech into understandable tokens by improving how speech is represented using different types of information.
What's the problem?
Mapping the complex features of speech into simple, discrete tokens (like words) is difficult. Existing methods often ignore important context, which can lead to mistakes in understanding and transcribing speech.
What's the solution?
The researchers developed DM-Codec, which combines three types of information—acoustic (sound), semantic (meaning), and contextual (situation)—to create better speech tokens. They used two new techniques to improve training: one that focuses on context and another that combines multiple sources of information to create a more effective tokenizer.
Why it matters?
This work is important because it can lead to more accurate speech recognition systems, which are essential for applications like voice assistants and transcription services. By reducing errors in understanding speech, it helps make technology more reliable and user-friendly.
Abstract
Recent advancements in speech-language models have yielded significant improvements in speech tokenization and synthesis. However, effectively mapping the complex, multidimensional attributes of speech into discrete tokens remains challenging. This process demands acoustic, semantic, and contextual information for precise speech representations. Existing speech representations generally fall into two categories: acoustic tokens from audio codecs and semantic tokens from speech self-supervised learning models. Although recent efforts have unified acoustic and semantic tokens for improved performance, they overlook the crucial role of contextual representation in comprehensive speech modeling. Our empirical investigations reveal that the absence of contextual representations results in elevated Word Error Rate (WER) and Word Information Lost (WIL) scores in speech transcriptions. To address these limitations, we propose two novel distillation approaches: (1) a language model (LM)-guided distillation method that incorporates contextual information, and (2) a combined LM and self-supervised speech model (SM)-guided distillation technique that effectively distills multimodal representations (acoustic, semantic, and contextual) into a comprehensive speech tokenizer, termed DM-Codec. The DM-Codec architecture adopts a streamlined encoder-decoder framework with a Residual Vector Quantizer (RVQ) and incorporates the LM and SM during the training process. Experiments show DM-Codec significantly outperforms state-of-the-art speech tokenization models, reducing WER by up to 13.46%, WIL by 9.82%, and improving speech quality by 5.84% and intelligibility by 1.85% on the LibriSpeech benchmark dataset. The code, samples, and model checkpoints are available at https://github.com/mubtasimahasan/DM-Codec.