DocLayout-YOLO: Enhancing Document Layout Analysis through Diverse Synthetic Data and Global-to-Local Adaptive Perception
Zhiyuan Zhao, Hengrui Kang, Bin Wang, Conghui He
2024-10-17
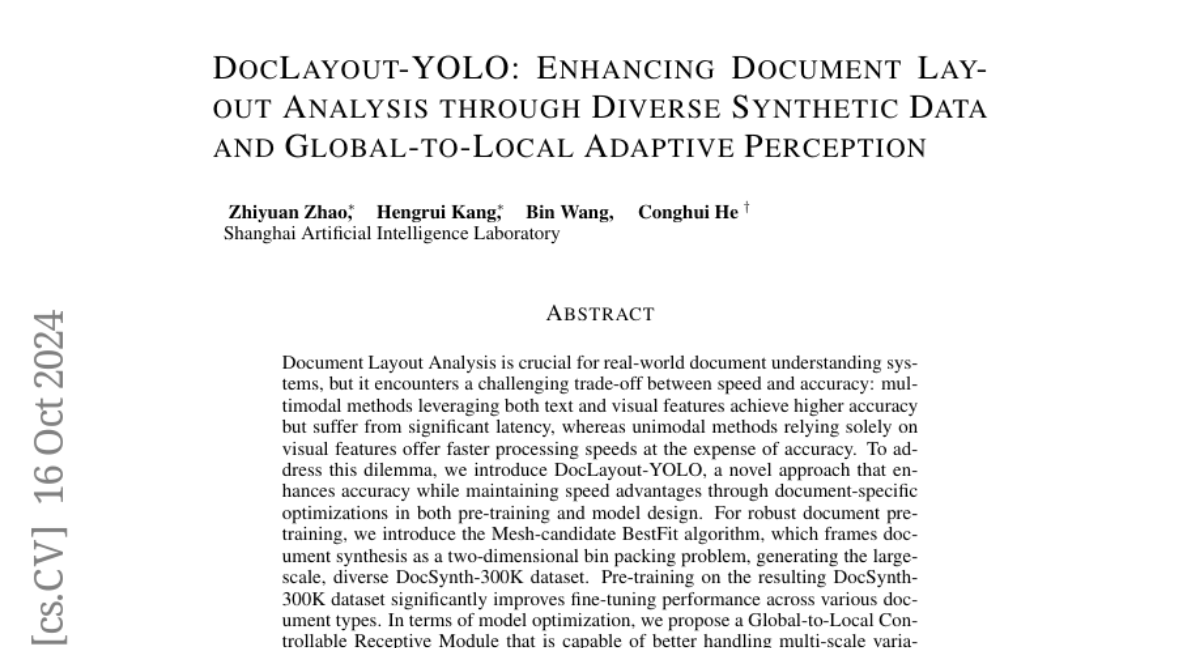
Summary
This paper introduces DocLayout-YOLO, a new method for analyzing document layouts that improves both accuracy and speed by using advanced techniques and a large synthetic dataset.
What's the problem?
Document layout analysis is important for understanding documents, but there is a trade-off between speed and accuracy. Methods that use both text and images (multimodal) are more accurate but slower, while methods that only use images (unimodal) are faster but less accurate. This makes it challenging to create systems that can analyze documents effectively in real-time.
What's the solution?
To solve this problem, the authors developed DocLayout-YOLO, which enhances accuracy without sacrificing speed. They created a large dataset called DocSynth-300K using a special algorithm that treats document layout as a packing problem, ensuring the dataset is diverse and comprehensive. Additionally, they designed a new model component called the Global-to-Local Controllable Receptive Module to better handle different sizes of document elements. This combination allows the system to analyze documents quickly and accurately.
Why it matters?
This research is significant because it provides a more effective way to analyze document layouts, which is crucial for applications like scanning documents, extracting information, and automating data entry. By improving both speed and accuracy, DocLayout-YOLO can enhance various real-world applications in business, education, and beyond.
Abstract
Document Layout Analysis is crucial for real-world document understanding systems, but it encounters a challenging trade-off between speed and accuracy: multimodal methods leveraging both text and visual features achieve higher accuracy but suffer from significant latency, whereas unimodal methods relying solely on visual features offer faster processing speeds at the expense of accuracy. To address this dilemma, we introduce DocLayout-YOLO, a novel approach that enhances accuracy while maintaining speed advantages through document-specific optimizations in both pre-training and model design. For robust document pre-training, we introduce the Mesh-candidate BestFit algorithm, which frames document synthesis as a two-dimensional bin packing problem, generating the large-scale, diverse DocSynth-300K dataset. Pre-training on the resulting DocSynth-300K dataset significantly improves fine-tuning performance across various document types. In terms of model optimization, we propose a Global-to-Local Controllable Receptive Module that is capable of better handling multi-scale variations of document elements. Furthermore, to validate performance across different document types, we introduce a complex and challenging benchmark named DocStructBench. Extensive experiments on downstream datasets demonstrate that DocLayout-YOLO excels in both speed and accuracy. Code, data, and models are available at https://github.com/opendatalab/DocLayout-YOLO.