Document Parsing Unveiled: Techniques, Challenges, and Prospects for Structured Information Extraction
Qintong Zhang, Victor Shea-Jay Huang, Bin Wang, Junyuan Zhang, Zhengren Wang, Hao Liang, Shawn Wang, Matthieu Lin, Wentao Zhang, Conghui He
2024-10-29
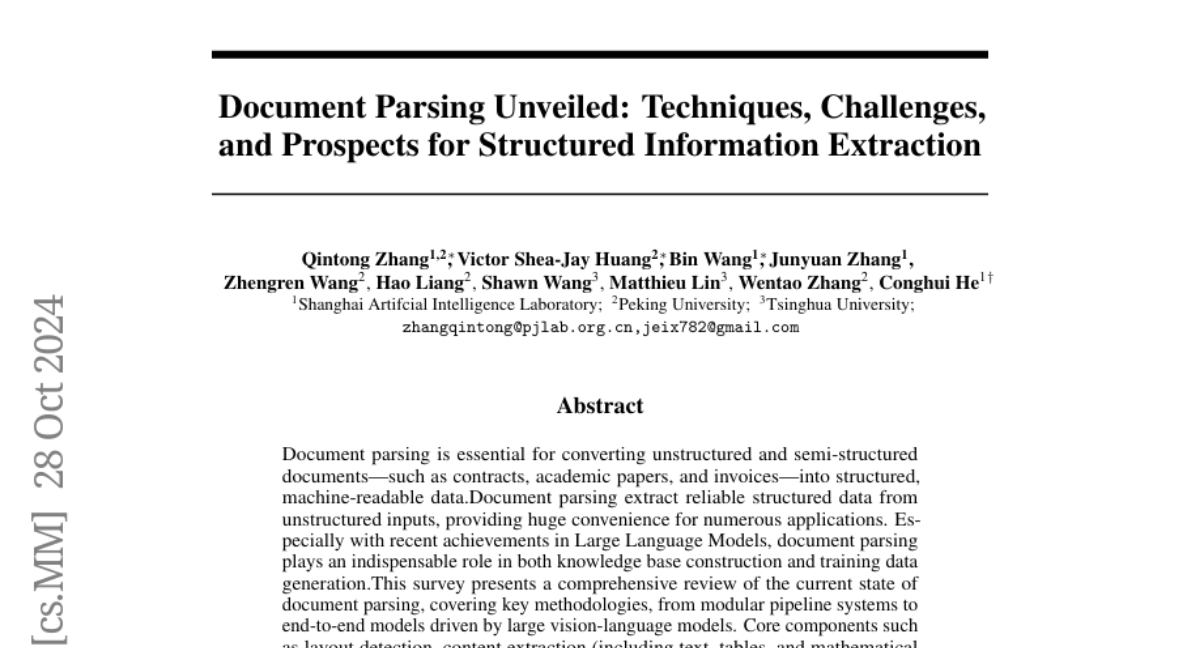
Summary
This paper provides an overview of document parsing, which is the process of converting unstructured documents like contracts and invoices into structured data that computers can easily read and understand.
What's the problem?
Many documents are unstructured or semi-structured, meaning they don't follow a clear format that computers can easily process. This makes it difficult for software to extract useful information from these documents, which is necessary for various applications like data analysis and knowledge management. Additionally, the rise of large language models has increased the need for effective document parsing methods.
What's the solution?
The authors review current techniques used in document parsing, including modular pipeline systems and end-to-end models that utilize advanced vision-language models. They discuss key components of the parsing process, such as detecting layouts, extracting content (like text and tables), and integrating different types of data. The paper also highlights challenges faced by these systems, such as dealing with complex document layouts and the need for larger datasets to improve performance.
Why it matters?
This research is important because effective document parsing can significantly enhance how we manage and utilize information from various sources. By improving the ability to extract structured data from unstructured documents, organizations can make better use of their data, leading to more informed decision-making and improved efficiency in many fields.
Abstract
Document parsing is essential for converting unstructured and semi-structured documents-such as contracts, academic papers, and invoices-into structured, machine-readable data. Document parsing extract reliable structured data from unstructured inputs, providing huge convenience for numerous applications. Especially with recent achievements in Large Language Models, document parsing plays an indispensable role in both knowledge base construction and training data generation. This survey presents a comprehensive review of the current state of document parsing, covering key methodologies, from modular pipeline systems to end-to-end models driven by large vision-language models. Core components such as layout detection, content extraction (including text, tables, and mathematical expressions), and multi-modal data integration are examined in detail. Additionally, this paper discusses the challenges faced by modular document parsing systems and vision-language models in handling complex layouts, integrating multiple modules, and recognizing high-density text. It emphasizes the importance of developing larger and more diverse datasets and outlines future research directions.