Dolphin: Long Context as a New Modality for Energy-Efficient On-Device Language Models
Wei Chen, Zhiyuan Li, Shuo Xin, Yihao Wang
2024-08-29
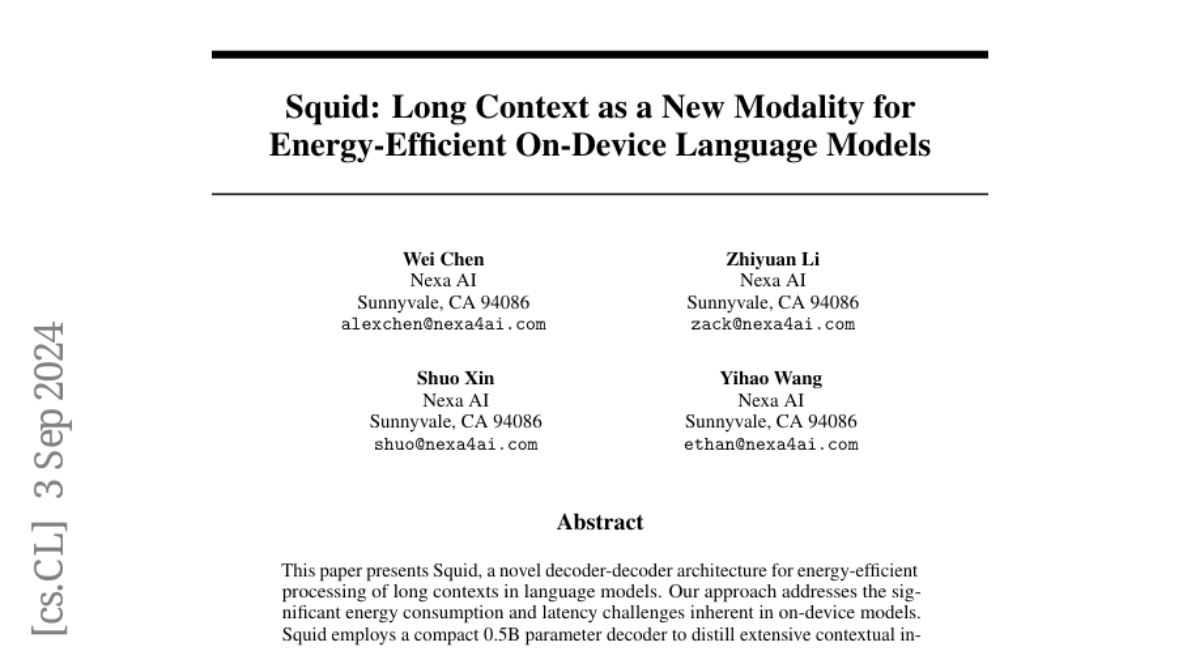
Summary
This paper introduces Dolphin, a new model designed to efficiently handle long text inputs for language processing on devices with limited energy and resources.
What's the problem?
Processing long contexts in language models can be very energy-intensive and slow, especially when these models are run on devices like smartphones or tablets. Traditional methods struggle with this because they require a lot of computational power, which can drain battery life and reduce performance.
What's the solution?
Dolphin uses a unique architecture that includes a smaller decoder to summarize long text inputs into a more manageable format before passing it to a larger decoder. This allows the model to process longer texts without using as much energy or time. The model treats long text as a different type of input, similar to how images are processed in vision-language models. Tests show that Dolphin is ten times more energy-efficient and five times faster than traditional methods while maintaining high-quality responses.
Why it matters?
This research is important because it makes advanced language processing more accessible on everyday devices. By improving efficiency, Dolphin enables more sophisticated AI applications in areas like mobile apps, virtual assistants, and other technologies where battery life and processing power are critical.
Abstract
This paper presents Dolphin, a novel decoder-decoder architecture for energy-efficient processing of long contexts in language models. Our approach addresses the significant energy consumption and latency challenges inherent in on-device models. Dolphin employs a compact 0.5B parameter decoder to distill extensive contextual information into a memory embedding, substantially reducing the input length for the primary 7B parameter decoder model. Inspired by vision-language models, we repurpose the image embedding projector to encode long textual contexts, effectively treating extended context as a distinct modality. This innovative method enables processing of substantially longer contexts without the typical computational overhead associated with extended input sequences. Empirical evaluations demonstrate a 10-fold improvement in energy efficiency and a 5-fold reduction in latency compared to conventional full-length context processing methods without losing quality of the response. Our work contributes to the development of more sustainable and scalable language models for on-device applications, addressing the critical need for energy-efficient and responsive AI technologies in resource-constrained environments while maintaining the accuracy to understand long contexts. This research has implications for the broader field of natural language processing, particularly in the domain of efficient model design for resource-limited settings. By enabling more sophisticated AI capabilities on edge devices, Dolphin paves the way for advanced language processing in a wide range of applications where computational resources are at a premium. The Dolphin model is publicly available at https://huggingface.co/NexaAIDev/Dolphin.