DPLM-2: A Multimodal Diffusion Protein Language Model
Xinyou Wang, Zaixiang Zheng, Fei Ye, Dongyu Xue, Shujian Huang, Quanquan Gu
2024-10-21
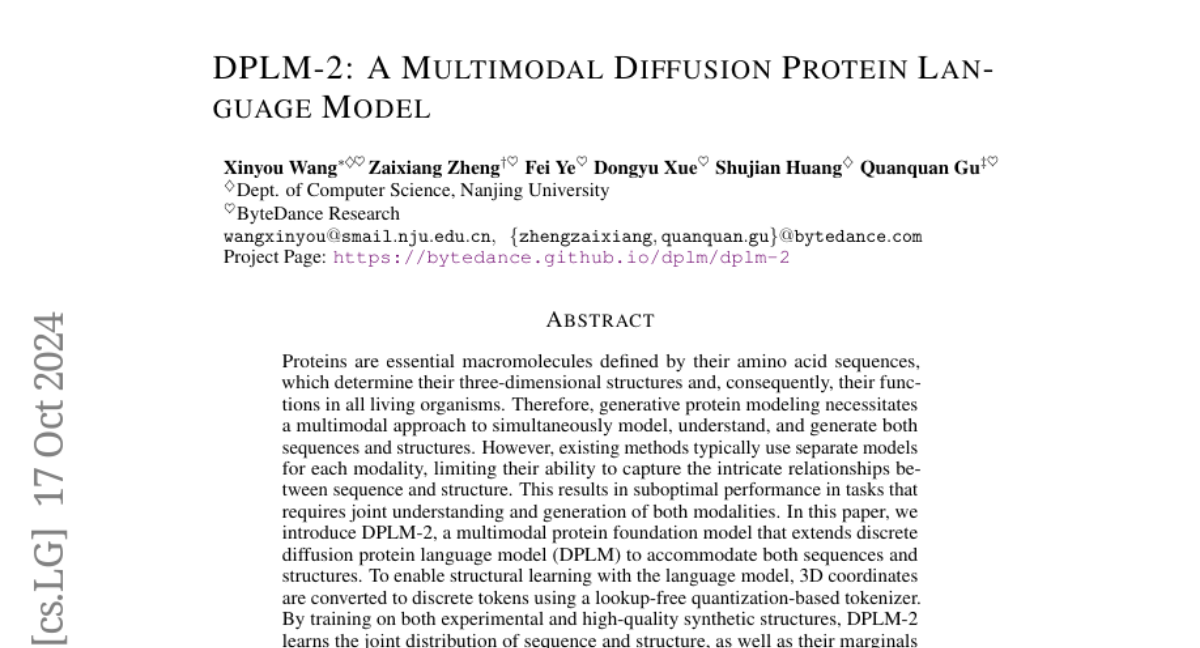
Summary
This paper introduces DPLM-2, a new multimodal model that can understand and generate both the sequences and structures of proteins, improving how we study and design these essential biological molecules.
What's the problem?
Proteins are crucial for life, and their functions depend on their specific sequences of amino acids and their three-dimensional shapes. Current methods for modeling proteins often use separate models for sequences (the order of amino acids) and structures (the 3D shape), which makes it hard to capture the complex relationships between the two. This separation can lead to less effective predictions and designs when trying to understand or create proteins.
What's the solution?
To address this issue, the authors developed DPLM-2, which combines the understanding of both protein sequences and structures into a single model. They created a system that converts 3D coordinates of protein structures into discrete tokens, allowing the model to learn how sequences relate to their shapes. By training on both real experimental data and high-quality synthetic data, DPLM-2 learns to generate compatible amino acid sequences along with their corresponding 3D structures all at once, rather than in separate stages. This approach improves efficiency and accuracy in protein modeling.
Why it matters?
This research is important because it enhances our ability to predict and design proteins, which are vital for many biological processes and applications in medicine, biotechnology, and research. By improving how we model proteins, DPLM-2 could lead to better drug designs, understanding of diseases, and advancements in synthetic biology.
Abstract
Proteins are essential macromolecules defined by their amino acid sequences, which determine their three-dimensional structures and, consequently, their functions in all living organisms. Therefore, generative protein modeling necessitates a multimodal approach to simultaneously model, understand, and generate both sequences and structures. However, existing methods typically use separate models for each modality, limiting their ability to capture the intricate relationships between sequence and structure. This results in suboptimal performance in tasks that requires joint understanding and generation of both modalities. In this paper, we introduce DPLM-2, a multimodal protein foundation model that extends discrete diffusion protein language model (DPLM) to accommodate both sequences and structures. To enable structural learning with the language model, 3D coordinates are converted to discrete tokens using a lookup-free quantization-based tokenizer. By training on both experimental and high-quality synthetic structures, DPLM-2 learns the joint distribution of sequence and structure, as well as their marginals and conditionals. We also implement an efficient warm-up strategy to exploit the connection between large-scale evolutionary data and structural inductive biases from pre-trained sequence-based protein language models. Empirical evaluation shows that DPLM-2 can simultaneously generate highly compatible amino acid sequences and their corresponding 3D structures eliminating the need for a two-stage generation approach. Moreover, DPLM-2 demonstrates competitive performance in various conditional generation tasks, including folding, inverse folding, and scaffolding with multimodal motif inputs, as well as providing structure-aware representations for predictive tasks.