DreamHOI: Subject-Driven Generation of 3D Human-Object Interactions with Diffusion Priors
Thomas Hanwen Zhu, Ruining Li, Tomas Jakab
2024-09-13
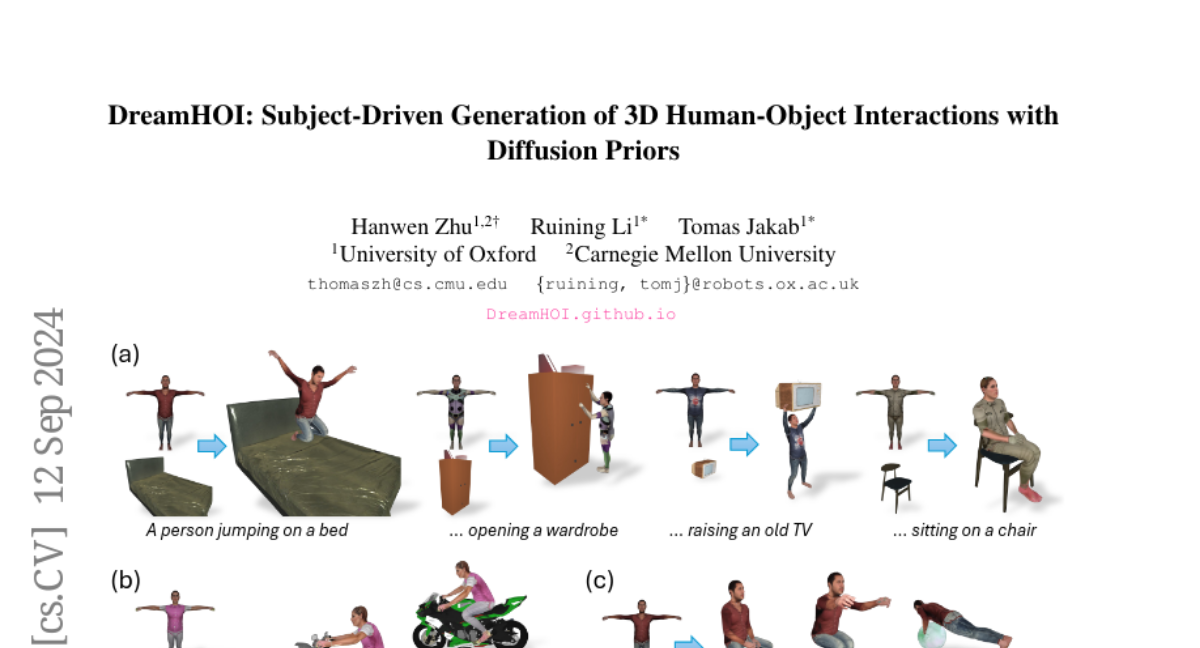
Summary
This paper introduces DreamHOI, a new method that allows AI to create realistic 3D scenes of humans interacting with objects based on simple text descriptions.
What's the problem?
Creating 3D models of humans interacting with various objects is difficult because there aren't enough examples of these interactions in existing datasets. Additionally, the shapes and types of objects can vary widely, making it hard for AI to generate accurate representations.
What's the solution?
DreamHOI uses advanced techniques to generate these interactions without needing a large dataset. It employs a method called Score Distillation Sampling (SDS) to improve how a human model moves and interacts with objects. The authors also developed a unique way to represent the human model that combines two approaches: implicit neural radiance fields (NeRFs) for smooth shapes and explicit skeleton-based articulation for detailed movements. This allows the AI to create realistic interactions by refining how the human model moves in relation to the object.
Why it matters?
This research is significant because it enables the creation of diverse and realistic 3D scenes for applications like video games, virtual reality, and animation. By allowing AI to generate these scenes based on text descriptions, it opens up new possibilities for creative industries and enhances how we can visualize human-object interactions.
Abstract
We present DreamHOI, a novel method for zero-shot synthesis of human-object interactions (HOIs), enabling a 3D human model to realistically interact with any given object based on a textual description. This task is complicated by the varying categories and geometries of real-world objects and the scarcity of datasets encompassing diverse HOIs. To circumvent the need for extensive data, we leverage text-to-image diffusion models trained on billions of image-caption pairs. We optimize the articulation of a skinned human mesh using Score Distillation Sampling (SDS) gradients obtained from these models, which predict image-space edits. However, directly backpropagating image-space gradients into complex articulation parameters is ineffective due to the local nature of such gradients. To overcome this, we introduce a dual implicit-explicit representation of a skinned mesh, combining (implicit) neural radiance fields (NeRFs) with (explicit) skeleton-driven mesh articulation. During optimization, we transition between implicit and explicit forms, grounding the NeRF generation while refining the mesh articulation. We validate our approach through extensive experiments, demonstrating its effectiveness in generating realistic HOIs.