DreamVideo-2: Zero-Shot Subject-Driven Video Customization with Precise Motion Control
Yujie Wei, Shiwei Zhang, Hangjie Yuan, Xiang Wang, Haonan Qiu, Rui Zhao, Yutong Feng, Feng Liu, Zhizhong Huang, Jiaxin Ye, Yingya Zhang, Hongming Shan
2024-10-18
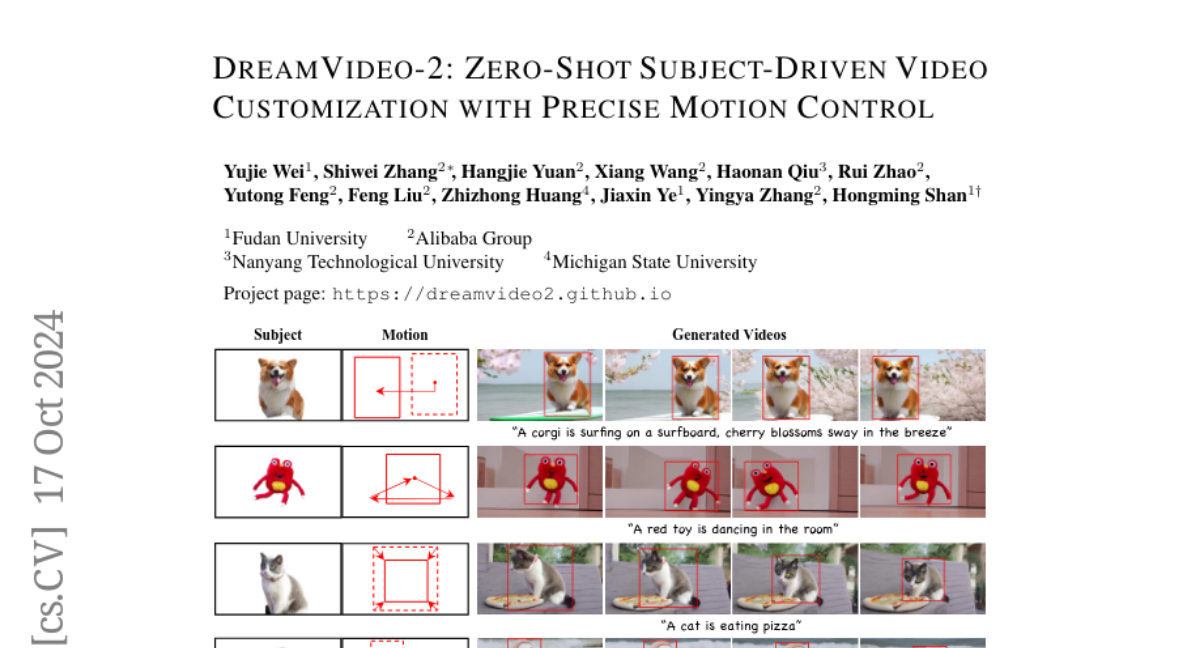
Summary
This paper introduces DreamVideo-2, a new system that allows users to customize videos by changing the main subject and controlling its movements without needing additional training.
What's the problem?
Current methods for customizing videos often require complex adjustments and fine-tuning, making it hard for users to easily change subjects or control motion. This limits how effectively these systems can be used in real-world applications, as they struggle to balance between learning about the subject and managing its movement.
What's the solution?
To solve this problem, the authors developed DreamVideo-2, which can generate customized videos based on just one image of the new subject and a sequence of bounding boxes that define the motion. The system uses two innovative components: reference attention to help it learn about the subject from the image, and a mask-guided motion module that accurately controls how the subject moves based on the bounding boxes. They also introduced techniques to ensure that both subject learning and motion control are balanced, leading to better overall performance.
Why it matters?
This research is important because it simplifies the process of creating customized videos, making it more accessible for users who want to create unique content without needing extensive technical knowledge. DreamVideo-2's ability to work 'zero-shot' means it can adapt to new subjects without prior examples, which opens up exciting possibilities for creative projects in areas like film, gaming, and social media.
Abstract
Recent advances in customized video generation have enabled users to create videos tailored to both specific subjects and motion trajectories. However, existing methods often require complicated test-time fine-tuning and struggle with balancing subject learning and motion control, limiting their real-world applications. In this paper, we present DreamVideo-2, a zero-shot video customization framework capable of generating videos with a specific subject and motion trajectory, guided by a single image and a bounding box sequence, respectively, and without the need for test-time fine-tuning. Specifically, we introduce reference attention, which leverages the model's inherent capabilities for subject learning, and devise a mask-guided motion module to achieve precise motion control by fully utilizing the robust motion signal of box masks derived from bounding boxes. While these two components achieve their intended functions, we empirically observe that motion control tends to dominate over subject learning. To address this, we propose two key designs: 1) the masked reference attention, which integrates a blended latent mask modeling scheme into reference attention to enhance subject representations at the desired positions, and 2) a reweighted diffusion loss, which differentiates the contributions of regions inside and outside the bounding boxes to ensure a balance between subject and motion control. Extensive experimental results on a newly curated dataset demonstrate that DreamVideo-2 outperforms state-of-the-art methods in both subject customization and motion control. The dataset, code, and models will be made publicly available.