DSTI at LLMs4OL 2024 Task A: Intrinsic versus extrinsic knowledge for type classification
Hanna Abi Akl
2024-08-28
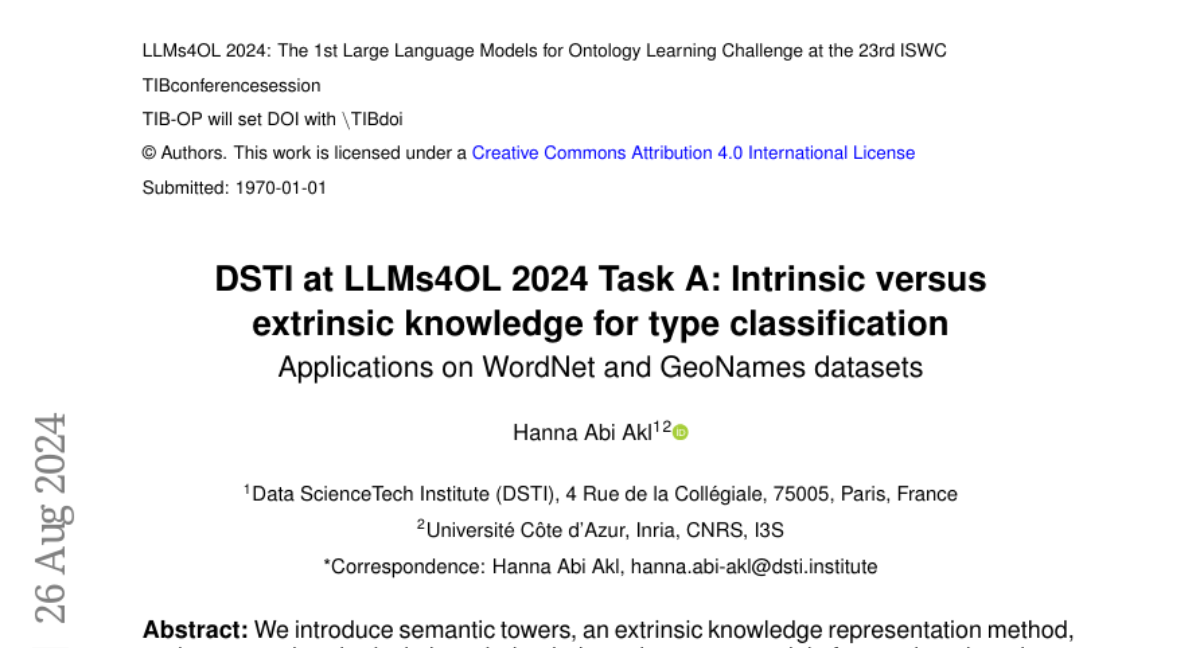
Summary
This paper discusses a project called SHADOW, which focuses on using advanced reasoning techniques to improve how computers understand and build knowledge from data.
What's the problem?
Building knowledge bases from data is challenging because existing methods often struggle to accurately connect different pieces of information. Traditional models may not be able to generalize well across various tasks, leading to incomplete or inaccurate knowledge bases.
What's the solution?
The authors introduce SHADOW, a fine-tuned language model that uses associative deductive reasoning to enhance the process of constructing knowledge bases. They compare this approach with standard methods and demonstrate that SHADOW performs significantly better, achieving a higher accuracy in completing knowledge triples, which are essential for organizing data.
Why it matters?
This research is important because it improves how machines can learn and organize information, making it easier to build accurate knowledge bases. This has applications in fields like artificial intelligence, data management, and information retrieval, ultimately helping users access better and more reliable information.
Abstract
We introduce semantic towers, an extrinsic knowledge representation method, and compare it to intrinsic knowledge in large language models for ontology learning. Our experiments show a trade-off between performance and semantic grounding for extrinsic knowledge compared to a fine-tuned model intrinsic knowledge. We report our findings on the Large Language Models for Ontology Learning (LLMs4OL) 2024 challenge.