DynaMath: A Dynamic Visual Benchmark for Evaluating Mathematical Reasoning Robustness of Vision Language Models
Chengke Zou, Xingang Guo, Rui Yang, Junyu Zhang, Bin Hu, Huan Zhang
2024-11-05
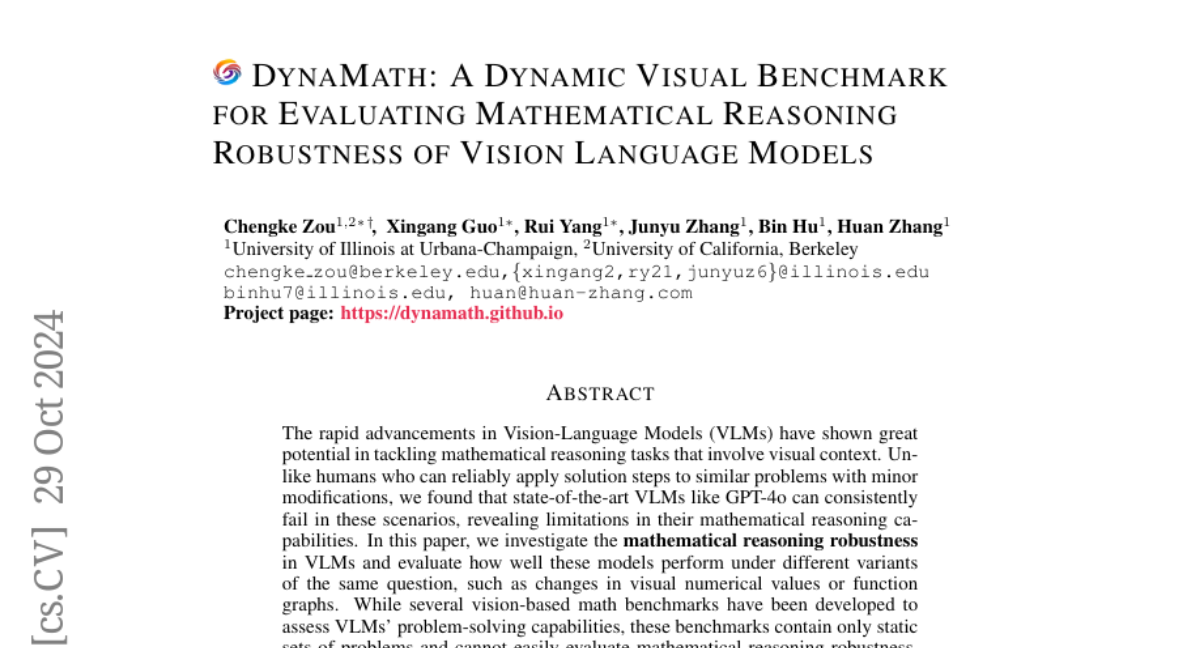
Summary
This paper presents DynaMath, a new benchmark designed to evaluate how well Vision-Language Models (VLMs) can reason mathematically when faced with different versions of the same question. It aims to test the models' ability to adapt to changes in visual information and question structure.
What's the problem?
While VLMs, like GPT-4o, have advanced in understanding language and images, they often struggle with mathematical reasoning tasks that involve slight variations in questions. Unlike humans, who can easily apply learned solutions to similar problems, these models can fail when the questions change even a little. Existing benchmarks for testing these models are limited because they only use fixed sets of problems, which do not adequately assess their reasoning abilities under different conditions.
What's the solution?
DynaMath addresses this issue by providing a dynamic benchmark that includes 501 high-quality seed questions. These questions are represented as Python programs and can automatically generate a larger variety of related questions with different visual and textual elements. This allows researchers to evaluate how well VLMs perform when faced with various versions of the same question. The authors tested 14 state-of-the-art VLMs using over 5,000 generated questions and found that the models' performance varied significantly depending on the question's format and details.
Why it matters?
This research is important because it highlights the need for better evaluation methods for AI models that perform mathematical reasoning. By using DynaMath, researchers can gain insights into how these models think and where they struggle, leading to improvements in their design. This could ultimately help create more reliable AI systems capable of solving complex problems in education, science, and technology.
Abstract
The rapid advancements in Vision-Language Models (VLMs) have shown great potential in tackling mathematical reasoning tasks that involve visual context. Unlike humans who can reliably apply solution steps to similar problems with minor modifications, we found that SOTA VLMs like GPT-4o can consistently fail in these scenarios, revealing limitations in their mathematical reasoning capabilities. In this paper, we investigate the mathematical reasoning robustness in VLMs and evaluate how well these models perform under different variants of the same question, such as changes in visual numerical values or function graphs. While several vision-based math benchmarks have been developed to assess VLMs' problem-solving capabilities, these benchmarks contain only static sets of problems and cannot easily evaluate mathematical reasoning robustness. To fill this gap, we introduce DynaMath, a dynamic visual math benchmark designed for in-depth assessment of VLMs. DynaMath includes 501 high-quality, multi-topic seed questions, each represented as a Python program. Those programs are carefully designed and annotated to enable the automatic generation of a much larger set of concrete questions, including many different types of visual and textual variations. DynaMath allows us to evaluate the generalization ability of VLMs, by assessing their performance under varying input conditions of a seed question. We evaluated 14 SOTA VLMs with 5,010 generated concrete questions. Our results show that the worst-case model accuracy, defined as the percentage of correctly answered seed questions in all 10 variants, is significantly lower than the average-case accuracy. Our analysis emphasizes the need to study the robustness of VLMs' reasoning abilities, and DynaMath provides valuable insights to guide the development of more reliable models for mathematical reasoning.