E.T. Bench: Towards Open-Ended Event-Level Video-Language Understanding
Ye Liu, Zongyang Ma, Zhongang Qi, Yang Wu, Ying Shan, Chang Wen Chen
2024-10-03
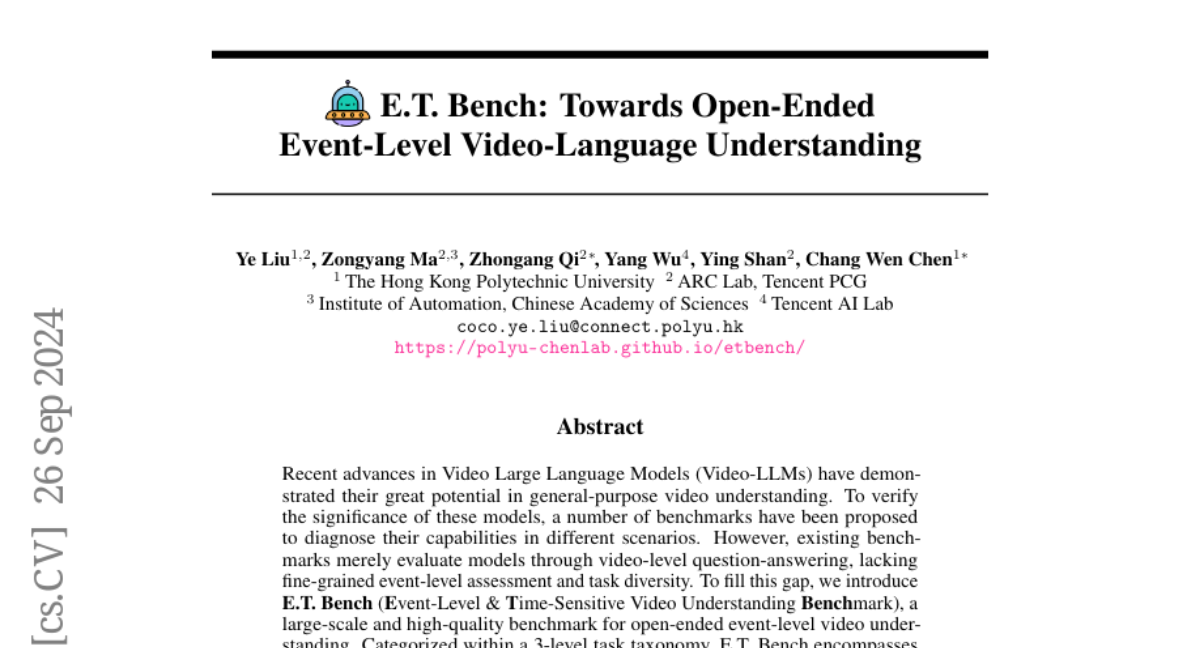
Summary
This paper introduces E.T. Bench, a new benchmark designed to evaluate how well video-language models understand events in videos, focusing on their ability to handle complex, time-sensitive situations.
What's the problem?
While existing video-language models can answer questions about entire videos, they often fail to analyze specific events within those videos. This lack of detailed evaluation means we don’t know how well these models can understand and respond to complex scenarios that involve multiple events happening over time.
What's the solution?
To address this issue, the authors created E.T. Bench, which includes a large dataset with 7,300 samples across 12 different tasks and 7,000 videos. This benchmark allows for a more detailed assessment of how well models can understand events in videos. They also developed a new model called E.T. Chat, which is designed to improve performance on these event-level tasks by focusing on specific details and relationships in the video content.
Why it matters?
This research is important because it provides a way to better evaluate and improve video-language models, ensuring they can accurately understand and respond to complex situations in videos. As video content becomes more prevalent in our digital world, having models that can effectively interpret and analyze this information is crucial for applications in education, entertainment, and information retrieval.
Abstract
Recent advances in Video Large Language Models (Video-LLMs) have demonstrated their great potential in general-purpose video understanding. To verify the significance of these models, a number of benchmarks have been proposed to diagnose their capabilities in different scenarios. However, existing benchmarks merely evaluate models through video-level question-answering, lacking fine-grained event-level assessment and task diversity. To fill this gap, we introduce E.T. Bench (Event-Level & Time-Sensitive Video Understanding Benchmark), a large-scale and high-quality benchmark for open-ended event-level video understanding. Categorized within a 3-level task taxonomy, E.T. Bench encompasses 7.3K samples under 12 tasks with 7K videos (251.4h total length) under 8 domains, providing comprehensive evaluations. We extensively evaluated 8 Image-LLMs and 12 Video-LLMs on our benchmark, and the results reveal that state-of-the-art models for coarse-level (video-level) understanding struggle to solve our fine-grained tasks, e.g., grounding event-of-interests within videos, largely due to the short video context length, improper time representations, and lack of multi-event training data. Focusing on these issues, we further propose a strong baseline model, E.T. Chat, together with an instruction-tuning dataset E.T. Instruct 164K tailored for fine-grained event-level understanding. Our simple but effective solution demonstrates superior performance in multiple scenarios.