Efficiently Democratizing Medical LLMs for 50 Languages via a Mixture of Language Family Experts
Guorui Zheng, Xidong Wang, Juhao Liang, Nuo Chen, Yuping Zheng, Benyou Wang
2024-10-16
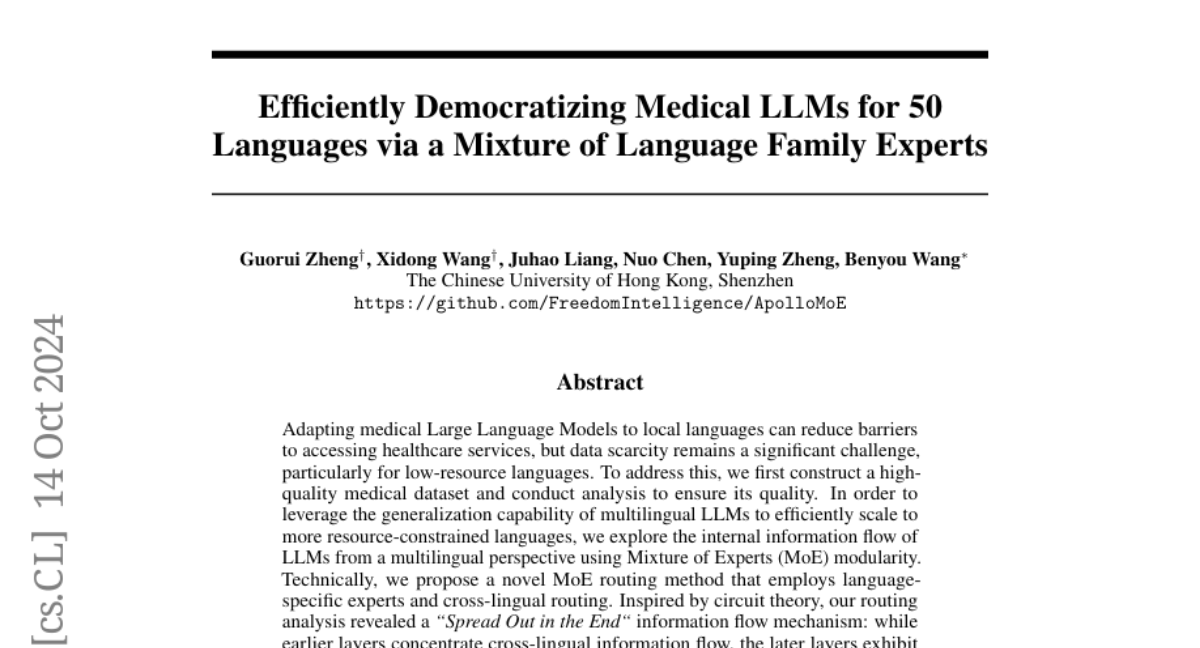
Summary
This paper discusses a new approach called Mixture of Language Family Experts (MoE) to adapt medical language models for 50 different languages, making healthcare information more accessible.
What's the problem?
Many medical language models struggle to work in local languages, especially those that are less commonly spoken, because there isn't enough data available in those languages. This makes it hard for people to access important healthcare information in their native tongue.
What's the solution?
To solve this problem, the authors created a high-quality medical dataset and used a method called MoE, which allows the model to use specialized experts for different language families. They designed a routing system that helps the model efficiently process information from various languages without needing a lot of extra data. This approach helps the model learn from existing data while being able to generalize its knowledge to new languages.
Why it matters?
This research is important because it can help democratize access to medical information across many languages, especially for communities that are often underserved. By improving how medical language models work in different languages, this work can lead to better healthcare outcomes and more inclusive access to vital information.
Abstract
Adapting medical Large Language Models to local languages can reduce barriers to accessing healthcare services, but data scarcity remains a significant challenge, particularly for low-resource languages. To address this, we first construct a high-quality medical dataset and conduct analysis to ensure its quality. In order to leverage the generalization capability of multilingual LLMs to efficiently scale to more resource-constrained languages, we explore the internal information flow of LLMs from a multilingual perspective using Mixture of Experts (MoE) modularity. Technically, we propose a novel MoE routing method that employs language-specific experts and cross-lingual routing. Inspired by circuit theory, our routing analysis revealed a Spread Out in the End information flow mechanism: while earlier layers concentrate cross-lingual information flow, the later layers exhibit language-specific divergence. This insight directly led to the development of the Post-MoE architecture, which applies sparse routing only in the later layers while maintaining dense others. Experimental results demonstrate that this approach enhances the generalization of multilingual models to other languages while preserving interpretability. Finally, to efficiently scale the model to 50 languages, we introduce the concept of language family experts, drawing on linguistic priors, which enables scaling the number of languages without adding additional parameters.