EgoVid-5M: A Large-Scale Video-Action Dataset for Egocentric Video Generation
Xiaofeng Wang, Kang Zhao, Feng Liu, Jiayu Wang, Guosheng Zhao, Xiaoyi Bao, Zheng Zhu, Yingya Zhang, Xingang Wang
2024-11-14
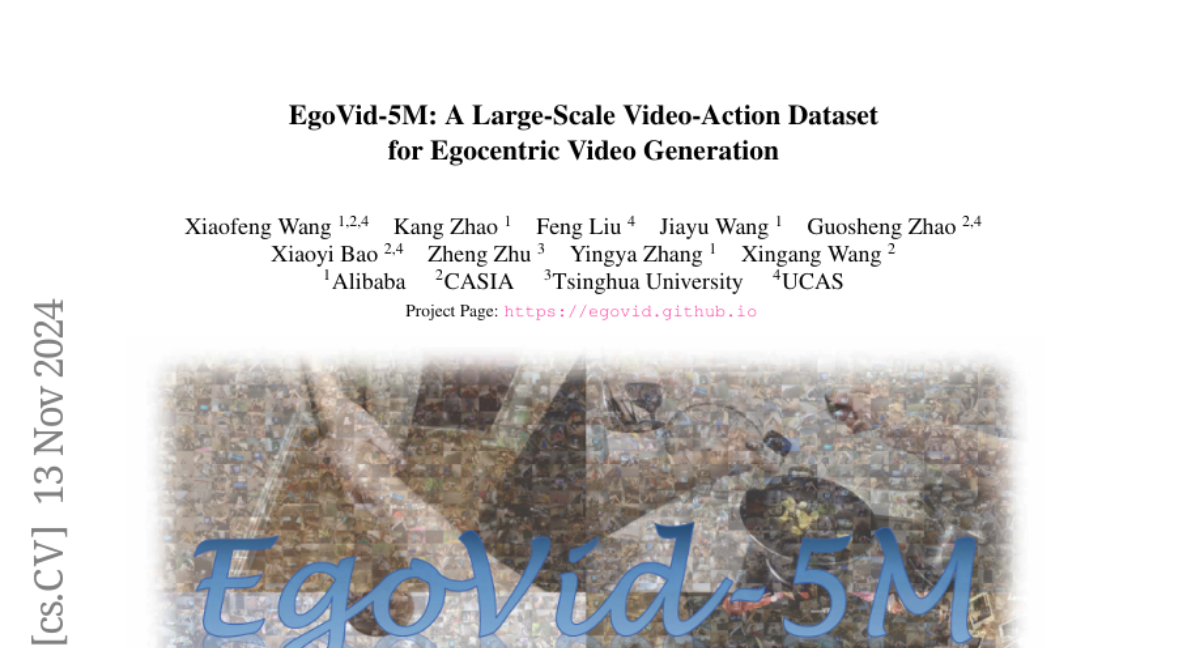
Summary
This paper introduces EgoVid-5M, a massive dataset designed to help create videos from a first-person perspective, which is important for applications like virtual reality and gaming.
What's the problem?
Generating videos that show what a person sees (egocentric videos) is challenging because it involves many different actions and scenes. Existing datasets do not provide enough variety or quality to train models effectively for this task.
What's the solution?
The authors created EgoVid-5M, which contains 5 million egocentric video clips with detailed annotations about the actions happening in each clip. They also developed a system called EgoDreamer that can generate new egocentric videos based on action descriptions and specific movement controls. To ensure the dataset's quality, they used a thorough cleaning process to maintain consistency and smoothness in the videos.
Why it matters?
This work is significant because it provides researchers with a high-quality resource for developing better egocentric video generation techniques. By improving how machines understand and create videos from a human perspective, it can enhance experiences in virtual reality, augmented reality, and gaming, making them more immersive and realistic.
Abstract
Video generation has emerged as a promising tool for world simulation, leveraging visual data to replicate real-world environments. Within this context, egocentric video generation, which centers on the human perspective, holds significant potential for enhancing applications in virtual reality, augmented reality, and gaming. However, the generation of egocentric videos presents substantial challenges due to the dynamic nature of egocentric viewpoints, the intricate diversity of actions, and the complex variety of scenes encountered. Existing datasets are inadequate for addressing these challenges effectively. To bridge this gap, we present EgoVid-5M, the first high-quality dataset specifically curated for egocentric video generation. EgoVid-5M encompasses 5 million egocentric video clips and is enriched with detailed action annotations, including fine-grained kinematic control and high-level textual descriptions. To ensure the integrity and usability of the dataset, we implement a sophisticated data cleaning pipeline designed to maintain frame consistency, action coherence, and motion smoothness under egocentric conditions. Furthermore, we introduce EgoDreamer, which is capable of generating egocentric videos driven simultaneously by action descriptions and kinematic control signals. The EgoVid-5M dataset, associated action annotations, and all data cleansing metadata will be released for the advancement of research in egocentric video generation.