EHRCon: Dataset for Checking Consistency between Unstructured Notes and Structured Tables in Electronic Health Records
Yeonsu Kwon, Jiho Kim, Gyubok Lee, Seongsu Bae, Daeun Kyung, Wonchul Cha, Tom Pollard, Alistair Johnson, Edward Choi
2024-06-27
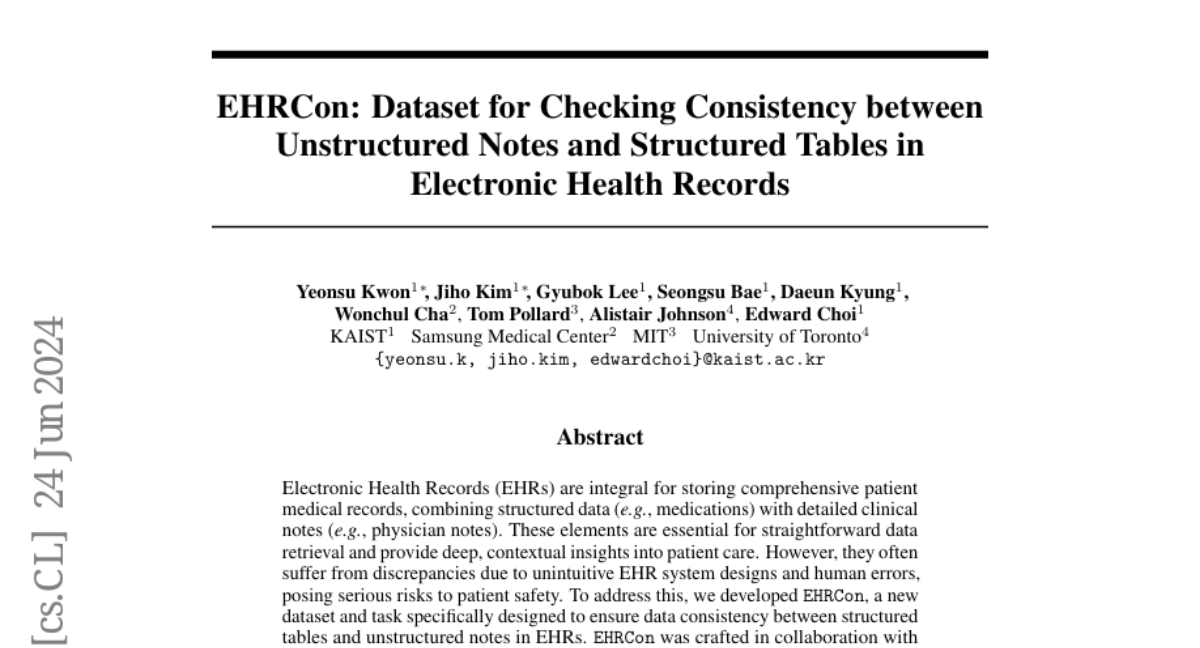
Summary
This paper talks about EHRCon, a new dataset and task created to check the consistency between unstructured clinical notes and structured data in Electronic Health Records (EHRs). It aims to improve patient safety by ensuring that all medical information is accurate and reliable.
What's the problem?
Electronic Health Records combine structured data, like medication lists, with unstructured data, such as detailed notes from doctors. However, these records often have inconsistencies due to poor design of EHR systems and human errors. This can lead to serious risks for patients, such as incorrect treatments or misdiagnoses because the information may not match up correctly.
What's the solution?
To solve this problem, the authors developed EHRCon, which includes a dataset of 3,943 entities from 105 clinical notes that have been checked against database entries for consistency. They also created a framework called CheckEHR that uses large language models to verify the accuracy of the information in these records. This framework follows an eight-step process to ensure that the clinical notes align with the structured data in the EHRs. EHRCon has two versions to make it more widely applicable and useful for different healthcare settings.
Why it matters?
This research is important because it helps ensure that patient records are accurate and consistent, which is crucial for providing safe and effective healthcare. By developing tools like EHRCon and CheckEHR, healthcare providers can reduce errors in patient records, ultimately improving patient care and safety.
Abstract
Electronic Health Records (EHRs) are integral for storing comprehensive patient medical records, combining structured data (e.g., medications) with detailed clinical notes (e.g., physician notes). These elements are essential for straightforward data retrieval and provide deep, contextual insights into patient care. However, they often suffer from discrepancies due to unintuitive EHR system designs and human errors, posing serious risks to patient safety. To address this, we developed EHRCon, a new dataset and task specifically designed to ensure data consistency between structured tables and unstructured notes in EHRs. EHRCon was crafted in collaboration with healthcare professionals using the MIMIC-III EHR dataset, and includes manual annotations of 3,943 entities across 105 clinical notes checked against database entries for consistency. EHRCon has two versions, one using the original MIMIC-III schema, and another using the OMOP CDM schema, in order to increase its applicability and generalizability. Furthermore, leveraging the capabilities of large language models, we introduce CheckEHR, a novel framework for verifying the consistency between clinical notes and database tables. CheckEHR utilizes an eight-stage process and shows promising results in both few-shot and zero-shot settings. The code is available at https://github.com/dustn1259/EHRCon.