Emergence of Abstractions: Concept Encoding and Decoding Mechanism for In-Context Learning in Transformers
Seungwook Han, Jinyeop Song, Jeff Gore, Pulkit Agrawal
2024-12-18
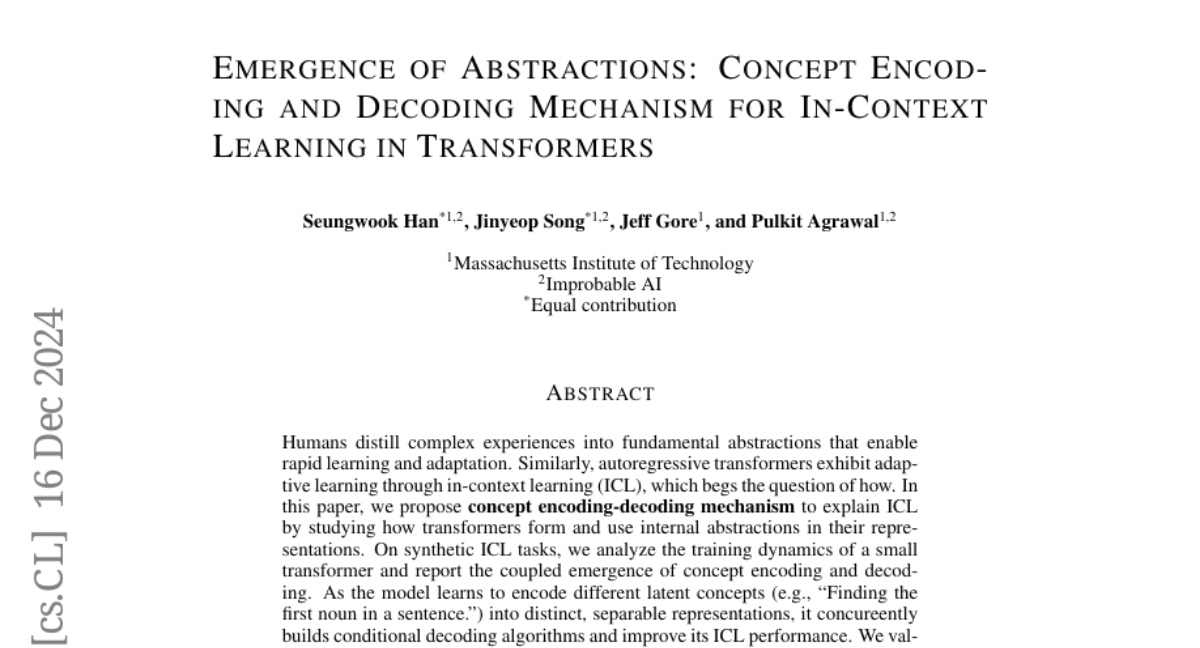
Summary
This paper talks about the emergence of abstractions in transformers, explaining how these models learn to understand and use complex concepts through a process called in-context learning (ICL).
What's the problem?
Transformers, which are advanced AI models, can learn from examples given to them in real-time without needing to be retrained. However, it's unclear how they form and use internal concepts or abstractions to improve their reasoning and learning capabilities. Understanding this process is important for enhancing their performance in various tasks.
What's the solution?
The authors propose a mechanism called concept encoding-decoding that helps explain how transformers develop and utilize these internal abstractions during ICL. They conducted experiments with a small transformer model to observe how it learns to encode different concepts into separate representations while simultaneously developing ways to decode them for reasoning. Their findings show that as the model improves its ability to encode concepts, its performance in ICL also enhances. They validated this mechanism across different pretrained transformer models of various sizes.
Why it matters?
This research is significant because it provides insights into how transformers learn and reason, which can help improve their effectiveness in real-world applications. By understanding the underlying processes of ICL, developers can create better AI models that can adapt more quickly and accurately to new tasks, making them more useful in fields like education, healthcare, and technology.
Abstract
Humans distill complex experiences into fundamental abstractions that enable rapid learning and adaptation. Similarly, autoregressive transformers exhibit adaptive learning through in-context learning (ICL), which begs the question of how. In this paper, we propose concept encoding-decoding mechanism to explain ICL by studying how transformers form and use internal abstractions in their representations. On synthetic ICL tasks, we analyze the training dynamics of a small transformer and report the coupled emergence of concept encoding and decoding. As the model learns to encode different latent concepts (e.g., ``Finding the first noun in a sentence.") into distinct, separable representations, it concureently builds conditional decoding algorithms and improve its ICL performance. We validate the existence of this mechanism across pretrained models of varying scales (Gemma-2 2B/9B/27B, Llama-3.1 8B/70B). Further, through mechanistic interventions and controlled finetuning, we demonstrate that the quality of concept encoding is causally related and predictive of ICL performance. Our empirical insights shed light into better understanding the success and failure modes of large language models via their representations.