EMOVA: Empowering Language Models to See, Hear and Speak with Vivid Emotions
Kai Chen, Yunhao Gou, Runhui Huang, Zhili Liu, Daxin Tan, Jing Xu, Chunwei Wang, Yi Zhu, Yihan Zeng, Kuo Yang, Dingdong Wang, Kun Xiang, Haoyuan Li, Haoli Bai, Jianhua Han, Xiaohui Li, Weike Jin, Nian Xie, Yu Zhang, James T. Kwok, Hengshuang Zhao, Xiaodan Liang
2024-09-27
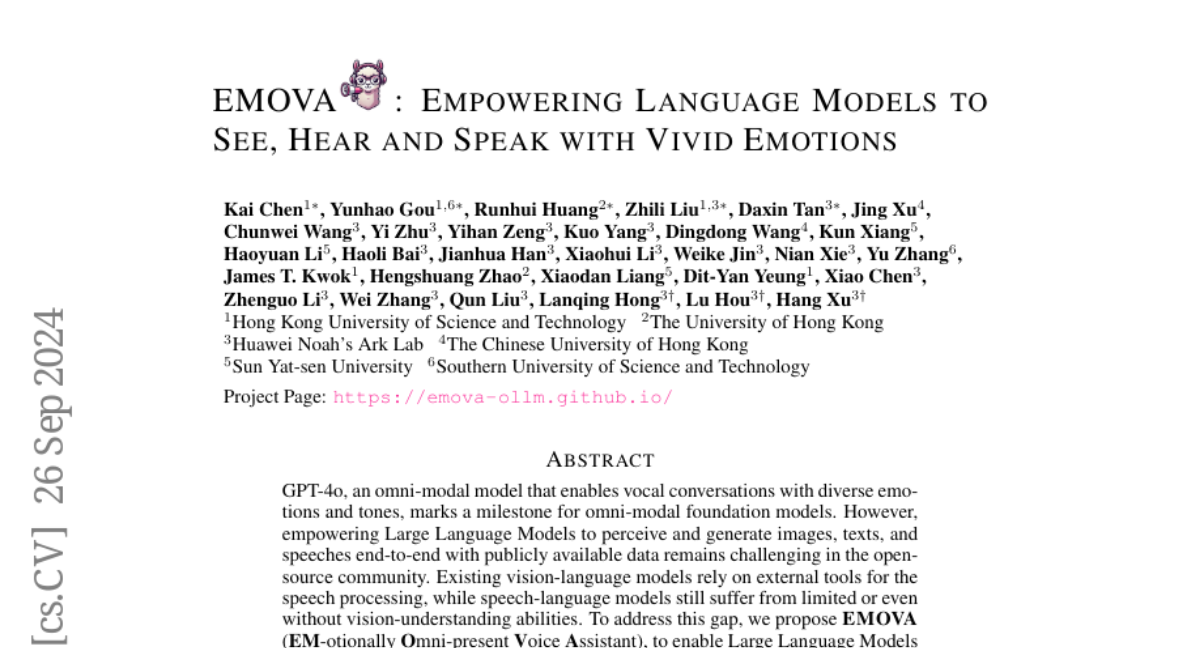
Summary
This paper talks about EMOVA, a new system that allows large language models (LLMs) to understand and express emotions through speech, text, and images. It aims to create a more interactive and emotionally aware AI assistant.
What's the problem?
While some advanced models like GPT-4o can have vocal conversations with different emotions, many existing models struggle to combine understanding of visual and auditory information with language. This limits their ability to engage in natural conversations that reflect human emotions and context. Current models either rely on external tools for speech processing or lack proper vision capabilities, making it hard for them to fully understand or generate emotional responses.
What's the solution?
To solve this issue, the researchers developed EMOVA (EMotionally Omni-present Voice Assistant), which integrates speech, vision, and text processing into one system. It uses a special tokenizer that separates semantic meaning from acoustic features, allowing the model to learn how to recognize emotions from both visual cues (like facial expressions) and auditory signals (like tone of voice). EMOVA can then generate responses that are not only textually accurate but also emotionally resonant. This system has been shown to perform exceptionally well on benchmarks for both vision-language and speech tasks.
Why it matters?
This research is important because it enhances the capabilities of AI systems to interact more naturally with humans. By enabling LLMs to perceive and express emotions effectively, EMOVA can improve user experiences in applications like virtual assistants, customer service bots, and educational tools, making them feel more relatable and human-like.
Abstract
GPT-4o, an omni-modal model that enables vocal conversations with diverse emotions and tones, marks a milestone for omni-modal foundation models. However, empowering Large Language Models to perceive and generate images, texts, and speeches end-to-end with publicly available data remains challenging in the open-source community. Existing vision-language models rely on external tools for the speech processing, while speech-language models still suffer from limited or even without vision-understanding abilities. To address this gap, we propose EMOVA (EMotionally Omni-present Voice Assistant), to enable Large Language Models with end-to-end speech capabilities while maintaining the leading vision-language performance. With a semantic-acoustic disentangled speech tokenizer, we notice surprisingly that omni-modal alignment can further enhance vision-language and speech abilities compared with the corresponding bi-modal aligned counterparts. Moreover, a lightweight style module is proposed for flexible speech style controls (e.g., emotions and pitches). For the first time, EMOVA achieves state-of-the-art performance on both the vision-language and speech benchmarks, and meanwhile, supporting omni-modal spoken dialogue with vivid emotions.