Enhancing Automated Interpretability with Output-Centric Feature Descriptions
Yoav Gur-Arieh, Roy Mayan, Chen Agassy, Atticus Geiger, Mor Geva
2025-01-15
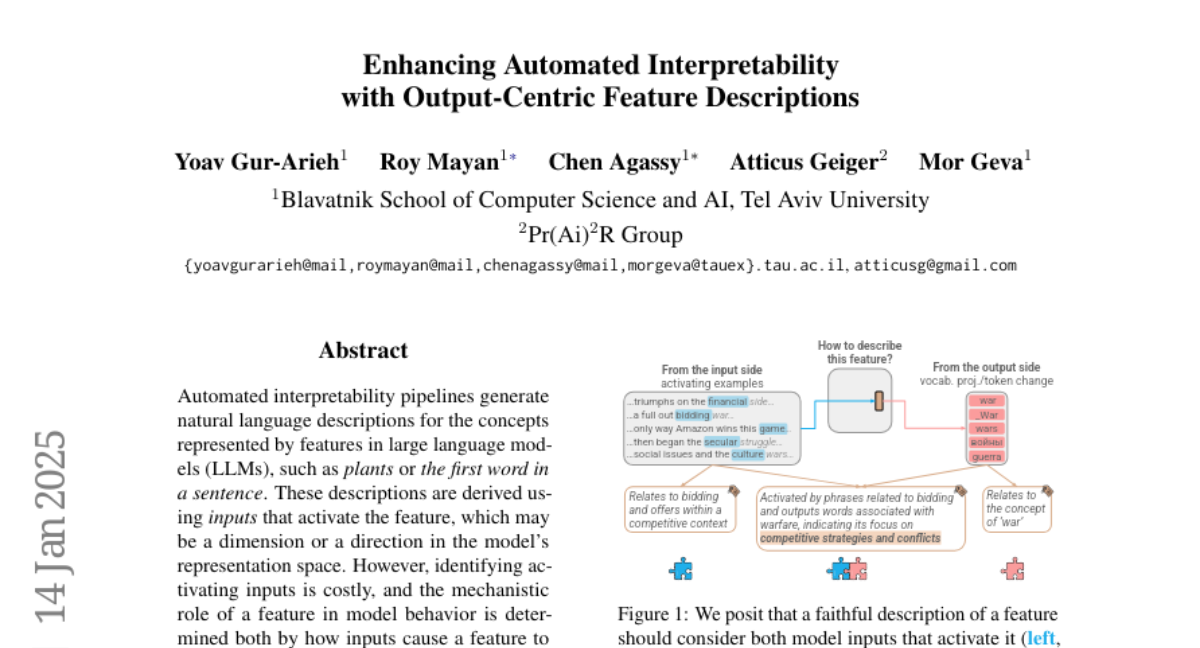
Summary
This paper talks about a new way to understand how AI language models work by looking at what they produce rather than just what goes into them. The researchers developed methods to describe the inner workings of these AI models more accurately and efficiently.
What's the problem?
Current ways of explaining how AI language models work focus on what kind of information makes certain parts of the AI 'light up' or activate. But this approach is slow and doesn't always show how these activations actually affect what the AI says or writes. It's like trying to understand how a car works by only looking at the gas pedal, without considering how pressing it makes the car move.
What's the solution?
The researchers came up with new methods that look at what the AI produces, not just what goes in. They focused on the words the AI is more likely to use after certain parts of it are activated. They also looked at how the AI's vocabulary changes when these parts are stimulated. By combining these new 'output-centric' methods with the old 'input-centric' ones, they got a much better picture of how the AI actually works.
Why it matters?
This matters because as AI becomes more common in our lives, it's crucial to understand how it makes decisions. Better understanding can lead to more trustworthy and reliable AI systems. It's like being able to explain not just why a car starts moving when you press the gas, but also how it decides which way to turn. This research could help make AI more transparent and easier to improve, which is important as we rely on it for more complex tasks in the future.
Abstract
Automated interpretability pipelines generate natural language descriptions for the concepts represented by features in large language models (LLMs), such as plants or the first word in a sentence. These descriptions are derived using inputs that activate the feature, which may be a dimension or a direction in the model's representation space. However, identifying activating inputs is costly, and the mechanistic role of a feature in model behavior is determined both by how inputs cause a feature to activate and by how feature activation affects outputs. Using steering evaluations, we reveal that current pipelines provide descriptions that fail to capture the causal effect of the feature on outputs. To fix this, we propose efficient, output-centric methods for automatically generating feature descriptions. These methods use the tokens weighted higher after feature stimulation or the highest weight tokens after applying the vocabulary "unembedding" head directly to the feature. Our output-centric descriptions better capture the causal effect of a feature on model outputs than input-centric descriptions, but combining the two leads to the best performance on both input and output evaluations. Lastly, we show that output-centric descriptions can be used to find inputs that activate features previously thought to be "dead".