Evaluating Multiview Object Consistency in Humans and Image Models
Tyler Bonnen, Stephanie Fu, Yutong Bai, Thomas O'Connell, Yoni Friedman, Nancy Kanwisher, Joshua B. Tenenbaum, Alexei A. Efros
2024-09-10
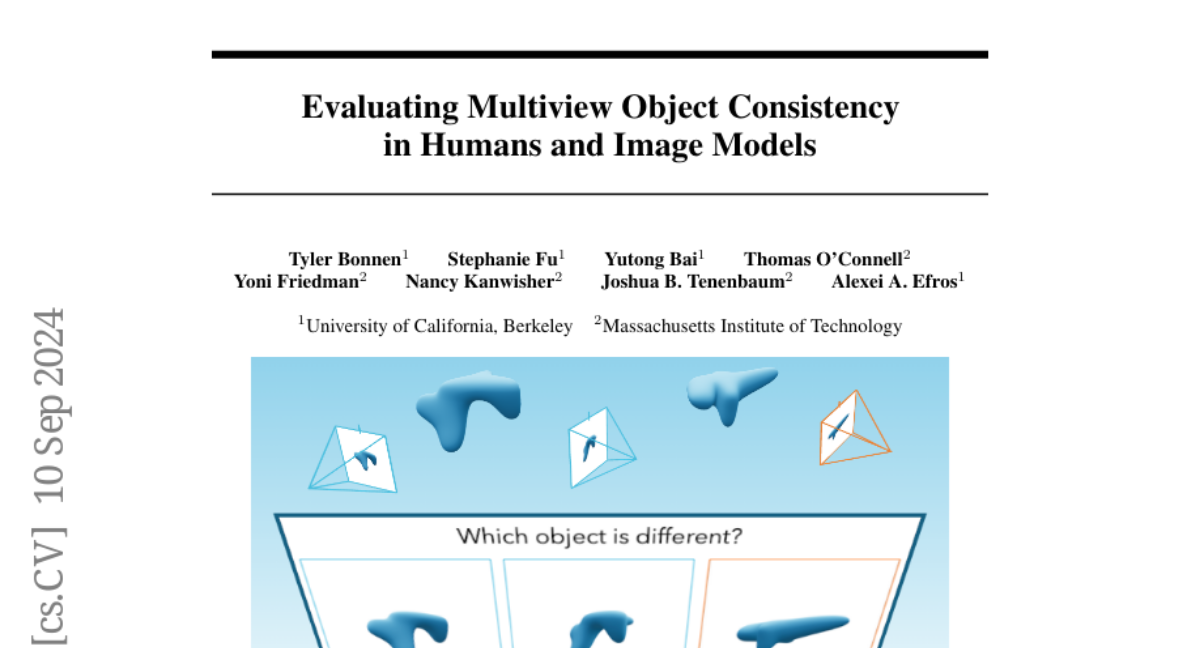
Summary
This paper talks about a benchmark designed to evaluate how well humans and vision models can identify and understand 3D shapes from images.
What's the problem?
While vision models have improved a lot in recognizing objects, they still struggle to match human abilities, especially when it comes to understanding shapes from different viewpoints. This is important because many real-world applications require accurate shape recognition, and existing tests do not effectively measure this capability.
What's the solution?
The authors created a benchmark that uses a set of images to see how well both humans and vision models can identify whether the images show the same or different objects, despite changes in perspective. They collected over 2,000 unique image sets and gathered data from more than 500 participants. They also tested various vision models to compare their performance against human observers.
Why it matters?
This research is important because it helps identify the strengths and weaknesses of both humans and AI in understanding complex visual information. By highlighting where AI falls short, it can guide future improvements in vision models, making them more effective for tasks that require detailed shape recognition.
Abstract
We introduce a benchmark to directly evaluate the alignment between human observers and vision models on a 3D shape inference task. We leverage an experimental design from the cognitive sciences which requires zero-shot visual inferences about object shape: given a set of images, participants identify which contain the same/different objects, despite considerable viewpoint variation. We draw from a diverse range of images that include common objects (e.g., chairs) as well as abstract shapes (i.e., procedurally generated `nonsense' objects). After constructing over 2000 unique image sets, we administer these tasks to human participants, collecting 35K trials of behavioral data from over 500 participants. This includes explicit choice behaviors as well as intermediate measures, such as reaction time and gaze data. We then evaluate the performance of common vision models (e.g., DINOv2, MAE, CLIP). We find that humans outperform all models by a wide margin. Using a multi-scale evaluation approach, we identify underlying similarities and differences between models and humans: while human-model performance is correlated, humans allocate more time/processing on challenging trials. All images, data, and code can be accessed via our project page.