Evaluating Open Language Models Across Task Types, Application Domains, and Reasoning Types: An In-Depth Experimental Analysis
Neelabh Sinha, Vinija Jain, Aman Chadha
2024-06-18
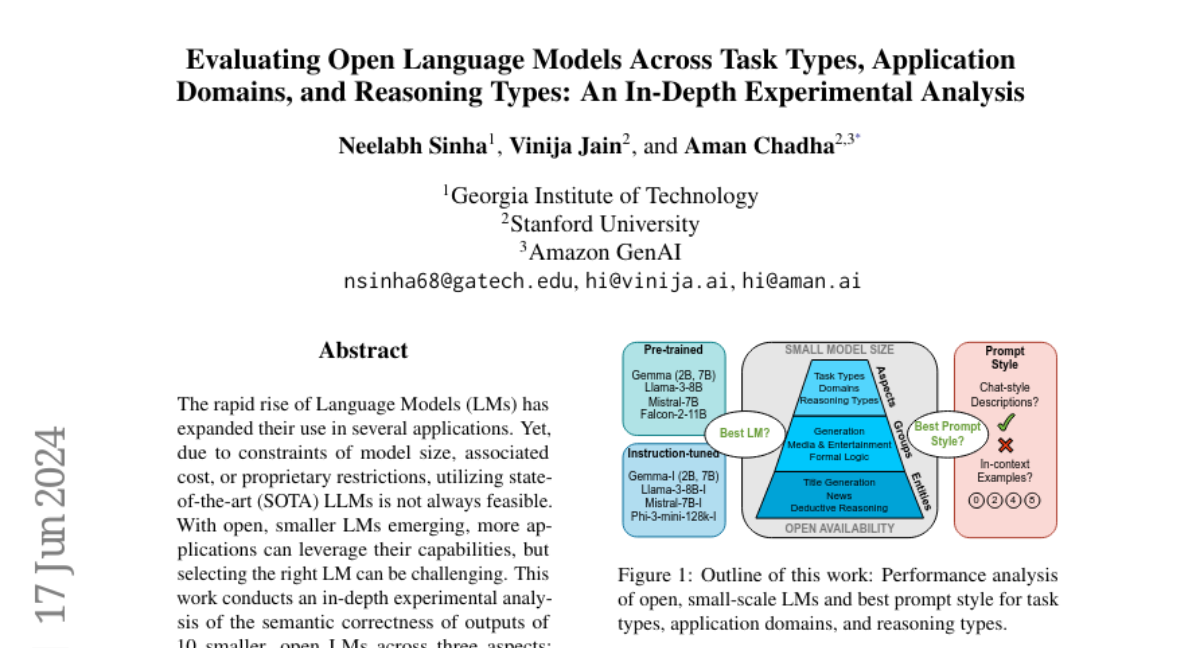
Summary
This paper analyzes how well smaller, open language models (LMs) perform across different tasks, application areas, and reasoning types. It aims to help users choose the right model for their specific needs by examining the outputs of these models under various conditions.
What's the problem?
As language models have become more popular, many advanced models are not accessible to everyone due to their size, cost, or proprietary restrictions. This makes it difficult for developers to find suitable alternatives that can still perform well. With the emergence of smaller, open-source LMs, users need guidance on which model to choose based on their specific tasks and requirements.
What's the solution?
To address this issue, the authors conducted a detailed analysis of ten smaller, open LMs by testing their outputs across different task types, application domains, and reasoning styles. They used various prompt styles to see how each model responded. The study created a three-tier framework that helps users strategically select models based on their specific use cases and constraints. The results showed that when used correctly, these smaller LMs can compete with or even outperform some of the best-known models like GPT-3.5-Turbo and GPT-4o.
Why it matters?
This research is important because it provides valuable insights into how smaller language models can be effectively used in real-world applications. By helping users understand which models work best for their needs, it encourages broader access to powerful AI tools without the high costs associated with larger models. This can lead to more innovation and creativity in fields like education, business, and technology.
Abstract
The rapid rise of Language Models (LMs) has expanded their use in several applications. Yet, due to constraints of model size, associated cost, or proprietary restrictions, utilizing state-of-the-art (SOTA) LLMs is not always feasible. With open, smaller LMs emerging, more applications can leverage their capabilities, but selecting the right LM can be challenging. This work conducts an in-depth experimental analysis of the semantic correctness of outputs of 10 smaller, open LMs across three aspects: task types, application domains and reasoning types, using diverse prompt styles. We demonstrate that most effective models and prompt styles vary depending on the specific requirements. Our analysis provides a comparative assessment of LMs and prompt styles using a proposed three-tier schema of aspects for their strategic selection based on use-case and other constraints. We also show that if utilized appropriately, these LMs can compete with, and sometimes outperform, SOTA LLMs like DeepSeek-v2, GPT-3.5-Turbo, and GPT-4o.