ExoViP: Step-by-step Verification and Exploration with Exoskeleton Modules for Compositional Visual Reasoning
Yuxuan Wang, Alan Yuille, Zhuowan Li, Zilong Zheng
2024-08-06
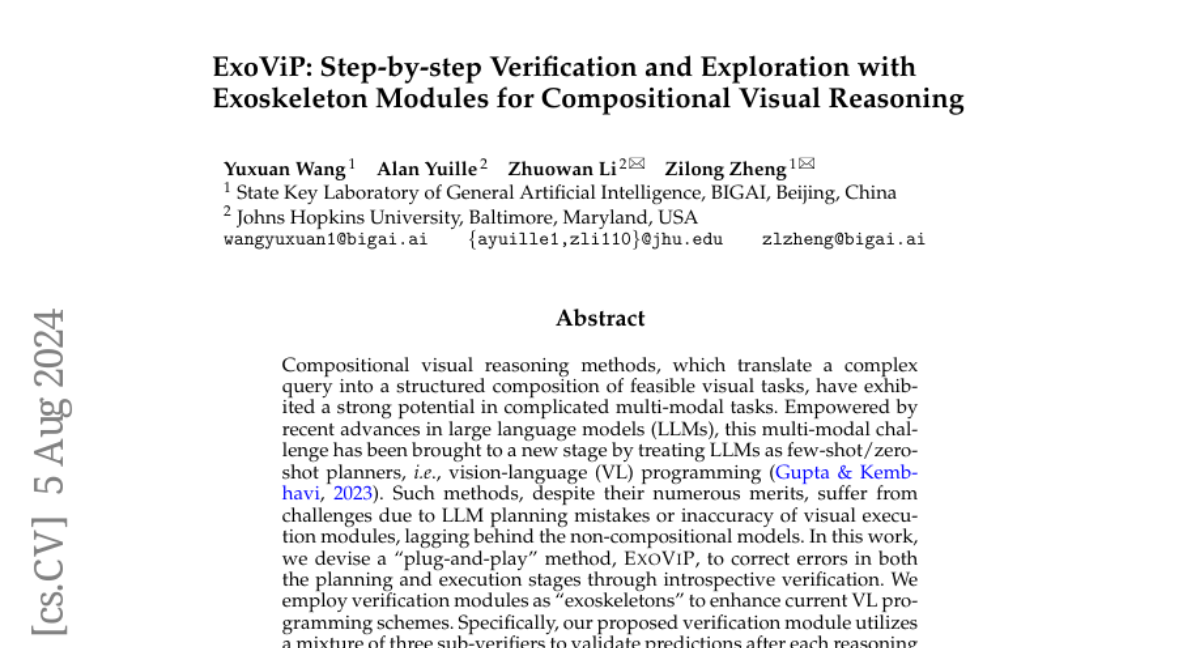
Summary
This paper introduces ExoViP, a new method that improves the accuracy of visual reasoning tasks by using verification modules to check and correct errors during the planning and execution stages.
What's the problem?
Compositional visual reasoning methods, which break down complex queries into manageable visual tasks, often make mistakes due to errors in planning or inaccuracies in executing visual tasks. These issues can lead to poor performance compared to simpler models that do not use this compositional approach.
What's the solution?
The authors developed ExoViP, a 'plug-and-play' method that employs verification modules, likened to 'exoskeletons,' to enhance the reasoning process. These modules validate the predictions made at each step and help refine the overall reasoning path planned by large language models (LLMs). By using a combination of three different verification methods, ExoViP effectively corrects both planning and execution errors, leading to better outcomes in visual reasoning tasks.
Why it matters?
ExoViP is significant because it enhances the performance of AI systems that rely on visual reasoning, making them more reliable and capable of handling complex multi-modal challenges. This improvement is crucial for applications like visual question answering and language-guided image editing, where accurate interpretation of visual information is essential.
Abstract
Compositional visual reasoning methods, which translate a complex query into a structured composition of feasible visual tasks, have exhibited a strong potential in complicated multi-modal tasks. Empowered by recent advances in large language models (LLMs), this multi-modal challenge has been brought to a new stage by treating LLMs as few-shot/zero-shot planners, i.e., vision-language (VL) programming. Such methods, despite their numerous merits, suffer from challenges due to LLM planning mistakes or inaccuracy of visual execution modules, lagging behind the non-compositional models. In this work, we devise a "plug-and-play" method, ExoViP, to correct errors in both the planning and execution stages through introspective verification. We employ verification modules as "exoskeletons" to enhance current VL programming schemes. Specifically, our proposed verification module utilizes a mixture of three sub-verifiers to validate predictions after each reasoning step, subsequently calibrating the visual module predictions and refining the reasoning trace planned by LLMs. Experimental results on two representative VL programming methods showcase consistent improvements on five compositional reasoning tasks on standard benchmarks. In light of this, we believe that ExoViP can foster better performance and generalization on open-domain multi-modal challenges.