Exploring Model Kinship for Merging Large Language Models
Yedi Hu, Yunzhi Yao, Ningyu Zhang, Shumin Deng, Huajun Chen
2024-10-17
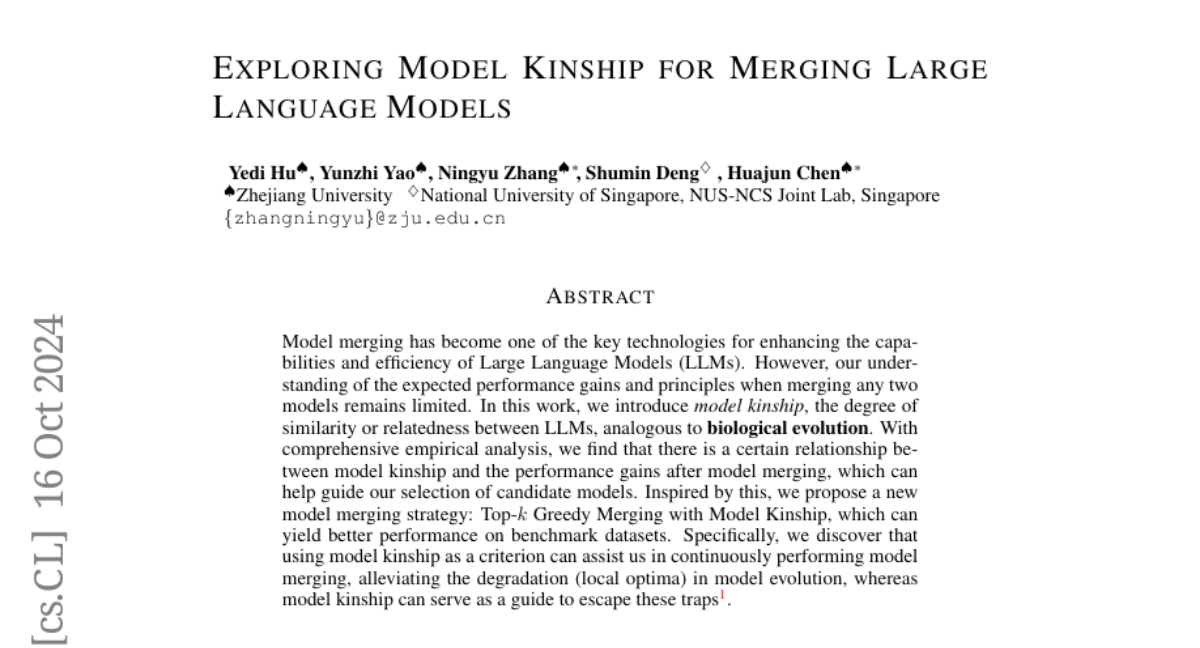
Summary
This paper discusses the concept of model kinship, which measures how similar large language models (LLMs) are to each other, and introduces a new strategy for merging these models to improve their performance.
What's the problem?
Merging LLMs is a key method for enhancing their capabilities, but we currently lack a clear understanding of how to effectively combine different models. Without knowing which models work well together, it can be difficult to achieve better performance after merging.
What's the solution?
The authors propose the idea of model kinship, which is like measuring the family resemblance between models. They found that there is a relationship between how similar two models are and the performance improvements that can be achieved by merging them. Based on this insight, they developed a new merging strategy called Top-k Greedy Merging with Model Kinship, which helps select the best models to merge for better results. This approach also helps avoid common problems that can occur during the merging process.
Why it matters?
This research matters because it provides a new way to think about and improve the merging of language models. By understanding model kinship, researchers can create more effective AI systems that perform better across various tasks, ultimately leading to advancements in artificial intelligence technology.
Abstract
Model merging has become one of the key technologies for enhancing the capabilities and efficiency of Large Language Models (LLMs). However, our understanding of the expected performance gains and principles when merging any two models remains limited. In this work, we introduce model kinship, the degree of similarity or relatedness between LLMs, analogous to biological evolution. With comprehensive empirical analysis, we find that there is a certain relationship between model kinship and the performance gains after model merging, which can help guide our selection of candidate models. Inspired by this, we propose a new model merging strategy: Top-k Greedy Merging with Model Kinship, which can yield better performance on benchmark datasets. Specifically, we discover that using model kinship as a criterion can assist us in continuously performing model merging, alleviating the degradation (local optima) in model evolution, whereas model kinship can serve as a guide to escape these traps. Code is available at https://github.com/zjunlp/ModelKinship.