ExVideo: Extending Video Diffusion Models via Parameter-Efficient Post-Tuning
Zhongjie Duan, Wenmeng Zhou, Cen Chen, Yaliang Li, Weining Qian
2024-06-21
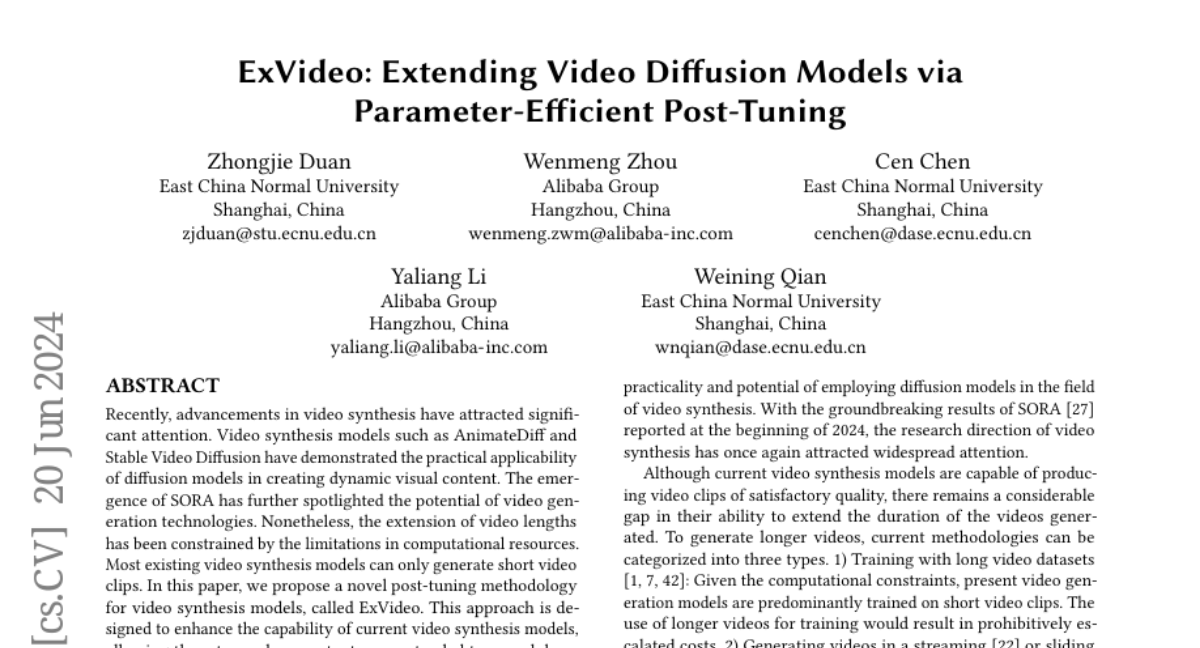
Summary
This paper introduces ExVideo, a new method that helps video synthesis models create longer videos while using fewer resources and maintaining high quality.
What's the problem?
Many existing video synthesis models, like AnimateDiff and Stable Video Diffusion, can only generate short clips due to limitations in computational power. This means they can't produce longer videos, which restricts their usefulness for applications that require more extended content. Additionally, creating longer videos usually requires a lot of time and resources for training.
What's the solution?
The researchers developed ExVideo, a post-tuning technique that enhances the ability of current video synthesis models to generate longer videos without needing extensive additional training. They designed specific strategies to improve how these models handle different types of data, such as using 3D convolution and temporal attention. By applying ExVideo to the Stable Video Diffusion model, they were able to increase its capacity to produce up to five times more frames than before, requiring only 1.5k GPU hours of training on a dataset of 40,000 videos. Importantly, this increase in video length did not reduce the model's ability to generalize or create diverse styles and resolutions.
Why it matters?
This research is important because it allows for the creation of longer and more complex videos with existing models, making them more versatile and effective for various applications like filmmaking, gaming, and virtual reality. By improving how these models work with limited resources, it opens up new possibilities for generating high-quality video content more efficiently.
Abstract
Recently, advancements in video synthesis have attracted significant attention. Video synthesis models such as AnimateDiff and Stable Video Diffusion have demonstrated the practical applicability of diffusion models in creating dynamic visual content. The emergence of SORA has further spotlighted the potential of video generation technologies. Nonetheless, the extension of video lengths has been constrained by the limitations in computational resources. Most existing video synthesis models can only generate short video clips. In this paper, we propose a novel post-tuning methodology for video synthesis models, called ExVideo. This approach is designed to enhance the capability of current video synthesis models, allowing them to produce content over extended temporal durations while incurring lower training expenditures. In particular, we design extension strategies across common temporal model architectures respectively, including 3D convolution, temporal attention, and positional embedding. To evaluate the efficacy of our proposed post-tuning approach, we conduct extension training on the Stable Video Diffusion model. Our approach augments the model's capacity to generate up to 5times its original number of frames, requiring only 1.5k GPU hours of training on a dataset comprising 40k videos. Importantly, the substantial increase in video length doesn't compromise the model's innate generalization capabilities, and the model showcases its advantages in generating videos of diverse styles and resolutions. We will release the source code and the enhanced model publicly.