Factorized-Dreamer: Training A High-Quality Video Generator with Limited and Low-Quality Data
Tao Yang, Yangming Shi, Yunwen Huang, Feng Chen, Yin Zheng, Lei Zhang
2024-08-20
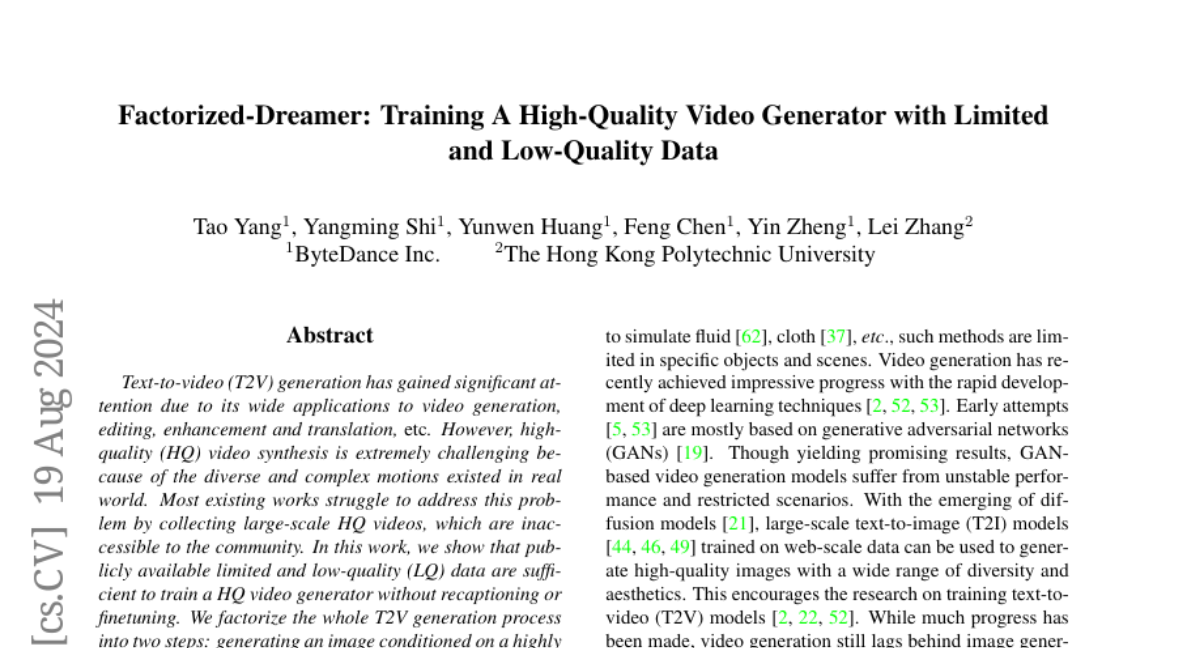
Summary
This paper introduces Factorized-Dreamer, a new method for generating high-quality videos from limited and low-quality data without needing extensive recaptioning or fine-tuning.
What's the problem?
Creating high-quality videos from text descriptions is very challenging because it usually requires a lot of high-quality video data, which is hard to obtain. Most existing methods rely on large datasets of clear and detailed videos, making it difficult for researchers who don't have access to such resources.
What's the solution?
Factorized-Dreamer breaks down the video generation process into two main steps: first, it generates an image based on a detailed text description, and then it creates a video using that image along with a shorter description of the motion. The model uses several advanced techniques, including combining different types of data inputs and employing a noise schedule to improve video quality. This allows it to work effectively with limited and low-quality datasets.
Why it matters?
This research is important because it makes it easier and cheaper to generate high-quality videos from text descriptions. By reducing the need for extensive high-quality data, Factorized-Dreamer opens up new possibilities for video generation in various fields, including entertainment, education, and content creation.
Abstract
Text-to-video (T2V) generation has gained significant attention due to its wide applications to video generation, editing, enhancement and translation, \etc. However, high-quality (HQ) video synthesis is extremely challenging because of the diverse and complex motions existed in real world. Most existing works struggle to address this problem by collecting large-scale HQ videos, which are inaccessible to the community. In this work, we show that publicly available limited and low-quality (LQ) data are sufficient to train a HQ video generator without recaptioning or finetuning. We factorize the whole T2V generation process into two steps: generating an image conditioned on a highly descriptive caption, and synthesizing the video conditioned on the generated image and a concise caption of motion details. Specifically, we present Factorized-Dreamer, a factorized spatiotemporal framework with several critical designs for T2V generation, including an adapter to combine text and image embeddings, a pixel-aware cross attention module to capture pixel-level image information, a T5 text encoder to better understand motion description, and a PredictNet to supervise optical flows. We further present a noise schedule, which plays a key role in ensuring the quality and stability of video generation. Our model lowers the requirements in detailed captions and HQ videos, and can be directly trained on limited LQ datasets with noisy and brief captions such as WebVid-10M, largely alleviating the cost to collect large-scale HQ video-text pairs. Extensive experiments in a variety of T2V and image-to-video generation tasks demonstrate the effectiveness of our proposed Factorized-Dreamer. Our source codes are available at https://github.com/yangxy/Factorized-Dreamer/.