Falcon Mamba: The First Competitive Attention-free 7B Language Model
Jingwei Zuo, Maksim Velikanov, Dhia Eddine Rhaiem, Ilyas Chahed, Younes Belkada, Guillaume Kunsch, Hakim Hacid
2024-10-10
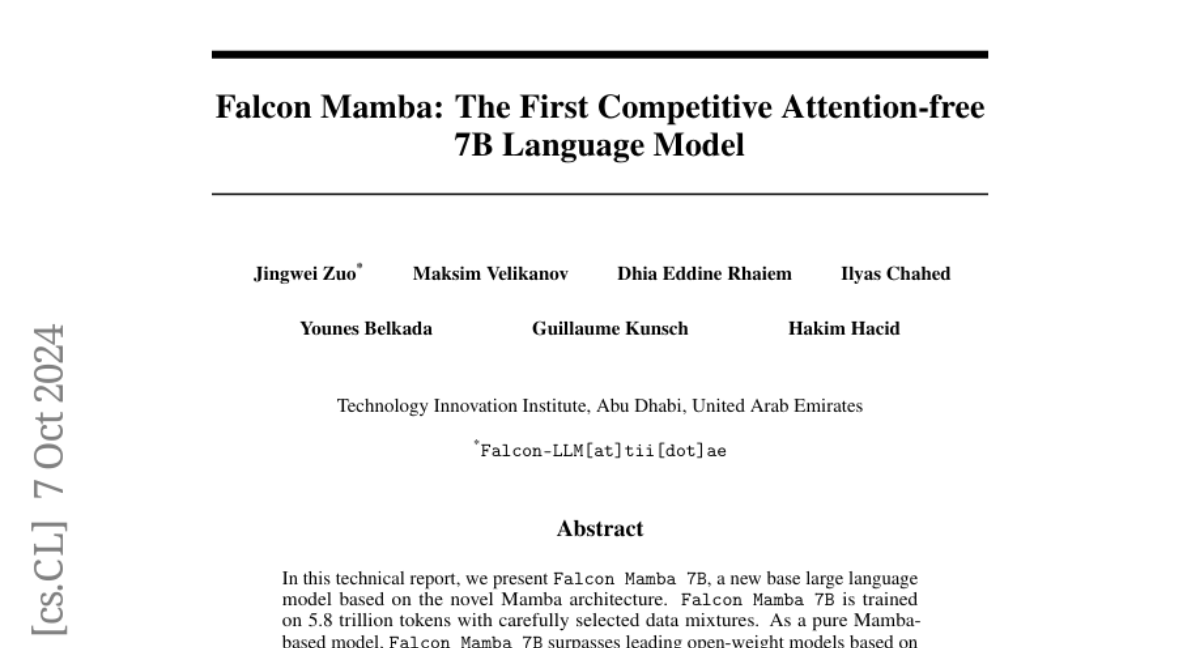
Summary
This paper introduces Falcon Mamba 7B, a new language model that uses a unique architecture called Mamba, which is designed to generate text efficiently without relying on traditional attention mechanisms.
What's the problem?
Most large language models today use a complex attention system that can slow down processing and require a lot of memory, especially when handling long sequences of text. This can make them less efficient and harder to use in real-world applications.
What's the solution?
Falcon Mamba 7B is built on the Mamba architecture, which simplifies the way the model processes information by using a method called Selective State Spaces instead of attention. This allows it to handle longer sequences more efficiently and with faster inference times. The model has been trained on a massive dataset of 5.8 trillion tokens, which helps it perform better than other models, even those that are larger. It also requires less memory, making it suitable for various applications.
Why it matters?
This research is significant because it challenges the traditional reliance on attention mechanisms in language models. By demonstrating that a simpler architecture can achieve competitive performance, Falcon Mamba 7B opens up new possibilities for developing faster and more efficient AI systems that can generate text and understand language better, benefiting fields like natural language processing, content creation, and more.
Abstract
In this technical report, we present Falcon Mamba 7B, a new base large language model based on the novel Mamba architecture. Falcon Mamba 7B is trained on 5.8 trillion tokens with carefully selected data mixtures. As a pure Mamba-based model, Falcon Mamba 7B surpasses leading open-weight models based on Transformers, such as Mistral 7B, Llama3.1 8B, and Falcon2 11B. It is on par with Gemma 7B and outperforms models with different architecture designs, such as RecurrentGemma 9B and RWKV-v6 Finch 7B/14B. Currently, Falcon Mamba 7B is the best-performing Mamba model in the literature at this scale, surpassing both existing Mamba and hybrid Mamba-Transformer models, according to the Open LLM Leaderboard. Due to its architecture, Falcon Mamba 7B is significantly faster at inference and requires substantially less memory for long sequence generation. Despite recent studies suggesting that hybrid Mamba-Transformer models outperform pure architecture designs, we demonstrate that even the pure Mamba design can achieve similar, or even superior results compared to the Transformer and hybrid designs. We make the weights of our implementation of Falcon Mamba 7B publicly available on https://huggingface.co/tiiuae/falcon-mamba-7b, under a permissive license.