FancyVideo: Towards Dynamic and Consistent Video Generation via Cross-frame Textual Guidance
Jiasong Feng, Ao Ma, Jing Wang, Bo Cheng, Xiaodan Liang, Dawei Leng, Yuhui Yin
2024-08-16
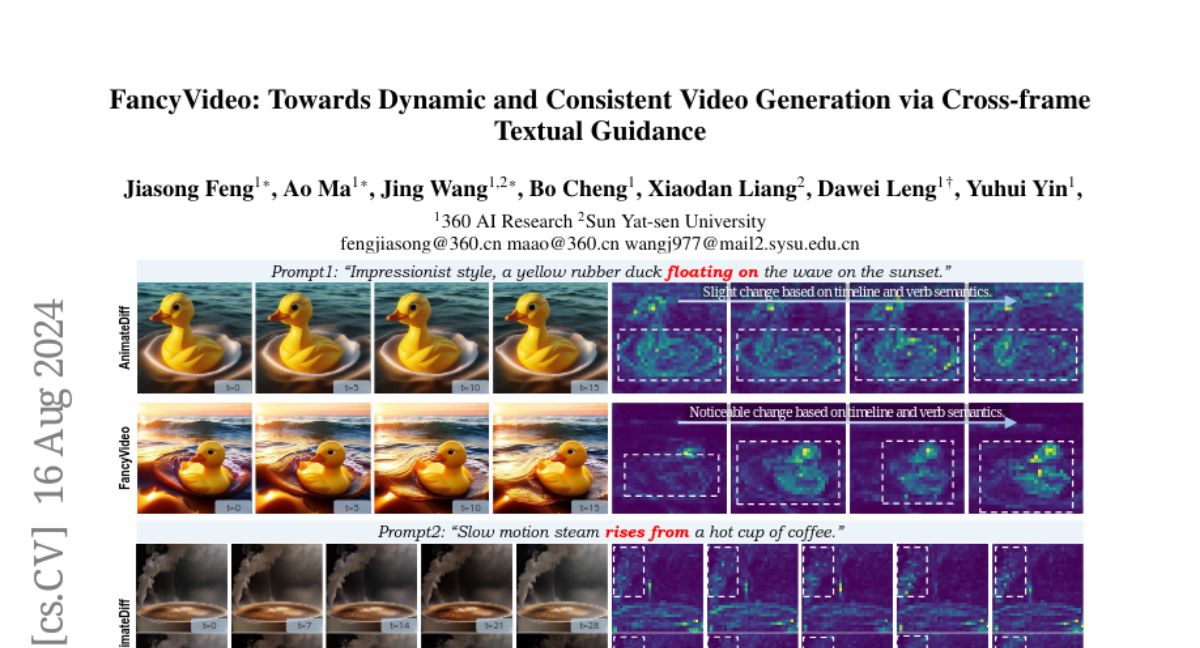
Summary
This paper introduces FancyVideo, a new method for generating videos that are both dynamic and consistent by using advanced techniques to guide the video creation process.
What's the problem?
Creating videos that show smooth and realistic motion over time is difficult for AI, especially when the videos need to be long. Current text-to-video models often struggle because they don’t use specific text instructions for each frame, which limits their ability to understand how actions should flow together.
What's the solution?
The authors developed FancyVideo, which includes a special system called the Cross-frame Textual Guidance Module (CTGM). This system helps the model understand and apply specific text instructions to each frame of the video. It uses several components to inject information about each frame, refine how frames relate to each other over time, and ensure that the video maintains a consistent look throughout. Extensive testing showed that FancyVideo produces high-quality videos that are both dynamic and coherent.
Why it matters?
This research is important because it improves the way AI can generate videos, making them more useful for applications like filmmaking, animation, and virtual reality. By enabling more precise control over how videos are created, it can lead to better storytelling and more engaging visual content.
Abstract
Synthesizing motion-rich and temporally consistent videos remains a challenge in artificial intelligence, especially when dealing with extended durations. Existing text-to-video (T2V) models commonly employ spatial cross-attention for text control, equivalently guiding different frame generations without frame-specific textual guidance. Thus, the model's capacity to comprehend the temporal logic conveyed in prompts and generate videos with coherent motion is restricted. To tackle this limitation, we introduce FancyVideo, an innovative video generator that improves the existing text-control mechanism with the well-designed Cross-frame Textual Guidance Module (CTGM). Specifically, CTGM incorporates the Temporal Information Injector (TII), Temporal Affinity Refiner (TAR), and Temporal Feature Booster (TFB) at the beginning, middle, and end of cross-attention, respectively, to achieve frame-specific textual guidance. Firstly, TII injects frame-specific information from latent features into text conditions, thereby obtaining cross-frame textual conditions. Then, TAR refines the correlation matrix between cross-frame textual conditions and latent features along the time dimension. Lastly, TFB boosts the temporal consistency of latent features. Extensive experiments comprising both quantitative and qualitative evaluations demonstrate the effectiveness of FancyVideo. Our approach achieves state-of-the-art T2V generation results on the EvalCrafter benchmark and facilitates the synthesis of dynamic and consistent videos. The video show results can be available at https://fancyvideo.github.io/, and we will make our code and model weights publicly available.