FastVoiceGrad: One-step Diffusion-Based Voice Conversion with Adversarial Conditional Diffusion Distillation
Takuhiro Kaneko, Hirokazu Kameoka, Kou Tanaka, Yuto Kondo
2024-09-05
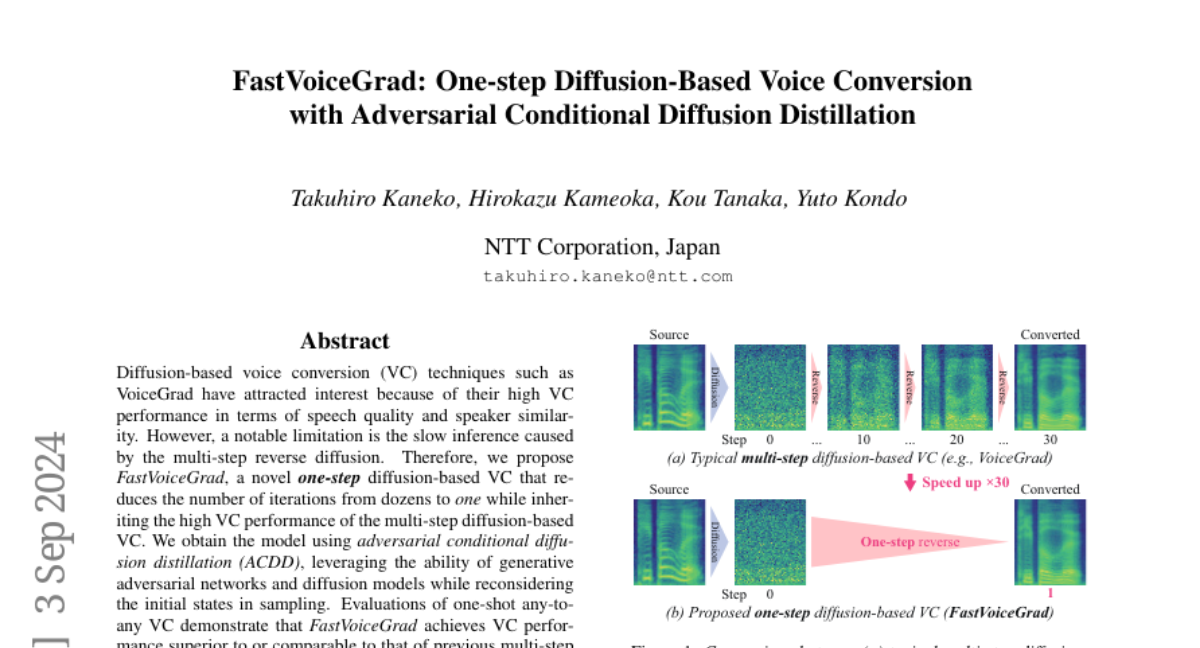
Summary
This paper talks about FastVoiceGrad, a new method for voice conversion that allows for fast and high-quality changes in a person's voice using audio input.
What's the problem?
Traditional voice conversion methods often take a long time because they require many steps to process the audio. This slow inference can be frustrating and limits the practical use of these technologies, especially when quick responses are needed.
What's the solution?
FastVoiceGrad solves this problem by using a one-step diffusion process instead of the usual multi-step approach. This means it can convert voices in just one go while still maintaining the quality and similarity of the original voice. The method combines techniques from generative adversarial networks (which help create realistic outputs) and diffusion models (which help refine those outputs), making it both efficient and effective.
Why it matters?
This research is important because it significantly speeds up voice conversion technology, making it more usable in real-time applications like virtual assistants, video games, or any situation where quick voice changes are needed. By improving the speed without sacrificing quality, FastVoiceGrad opens up new possibilities for how we can use voice technology.
Abstract
Diffusion-based voice conversion (VC) techniques such as VoiceGrad have attracted interest because of their high VC performance in terms of speech quality and speaker similarity. However, a notable limitation is the slow inference caused by the multi-step reverse diffusion. Therefore, we propose FastVoiceGrad, a novel one-step diffusion-based VC that reduces the number of iterations from dozens to one while inheriting the high VC performance of the multi-step diffusion-based VC. We obtain the model using adversarial conditional diffusion distillation (ACDD), leveraging the ability of generative adversarial networks and diffusion models while reconsidering the initial states in sampling. Evaluations of one-shot any-to-any VC demonstrate that FastVoiceGrad achieves VC performance superior to or comparable to that of previous multi-step diffusion-based VC while enhancing the inference speed. Audio samples are available at https://www.kecl.ntt.co.jp/people/kaneko.takuhiro/projects/fastvoicegrad/.