Finch: Prompt-guided Key-Value Cache Compression
Giulio Corallo, Paolo Papotti
2024-08-02
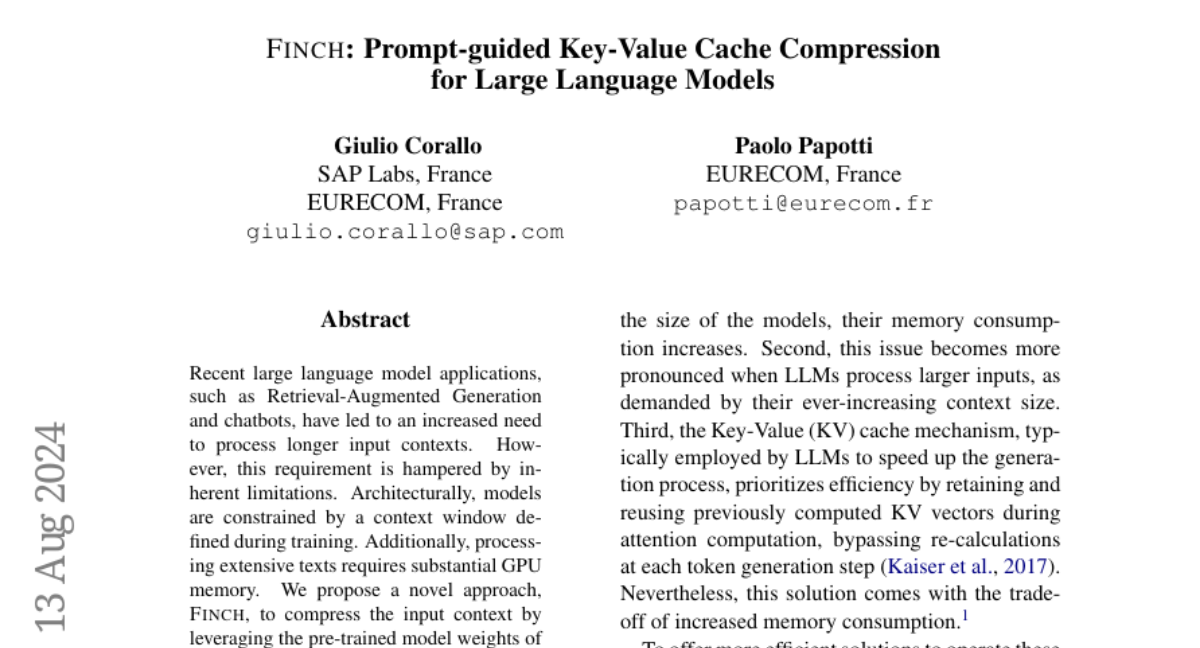
Summary
This paper presents Finch, a new method for compressing the input context used by large language models, allowing them to process longer texts more efficiently without losing important information.
What's the problem?
Large language models often have a limit on how much text they can handle at once, known as the context window. When trying to process long inputs, they can run out of memory and struggle to maintain performance. This makes it difficult to use these models for applications that require understanding extensive text, like chatbots or document analysis.
What's the solution?
To solve this problem, the authors developed Finch, which compresses the input context by focusing on the most relevant information. When given a prompt and a long text, Finch identifies and stores only the important Key (K) and Value (V) pairs from the text in a cache. This allows the model to work with a smaller amount of data while still keeping the essential meaning intact. Finch can compress this information by up to 93 times, making it possible for models to handle larger inputs efficiently without needing extra training.
Why it matters?
This research is significant because it enables large language models to be more practical and accessible for various applications. By reducing memory requirements and improving processing capabilities, Finch allows developers to create more powerful AI systems that can understand and generate text based on longer contexts. This could lead to better chatbots, more effective document summarization tools, and improved AI interactions in general.
Abstract
Recent large language model applications, such as Retrieval-Augmented Generation and chatbots, have led to an increased need to process longer input contexts. However, this requirement is hampered by inherent limitations. Architecturally, models are constrained by a context window defined during training. Additionally, processing extensive texts requires substantial GPU memory. We propose a novel approach, Finch, to compress the input context by leveraging the pre-trained model weights of the self-attention. Given a prompt and a long text, Finch iteratively identifies the most relevant Key (K) and Value (V) pairs over chunks of the text conditioned on the prompt. Only such pairs are stored in the KV cache, which, within the space constrained by the context window, ultimately contains a compressed version of the long text. Our proposal enables models to consume large inputs even with high compression (up to 93x) while preserving semantic integrity without the need for fine-tuning.