FIRE: A Dataset for Feedback Integration and Refinement Evaluation of Multimodal Models
Pengxiang Li, Zhi Gao, Bofei Zhang, Tao Yuan, Yuwei Wu, Mehrtash Harandi, Yunde Jia, Song-Chun Zhu, Qing Li
2024-07-17
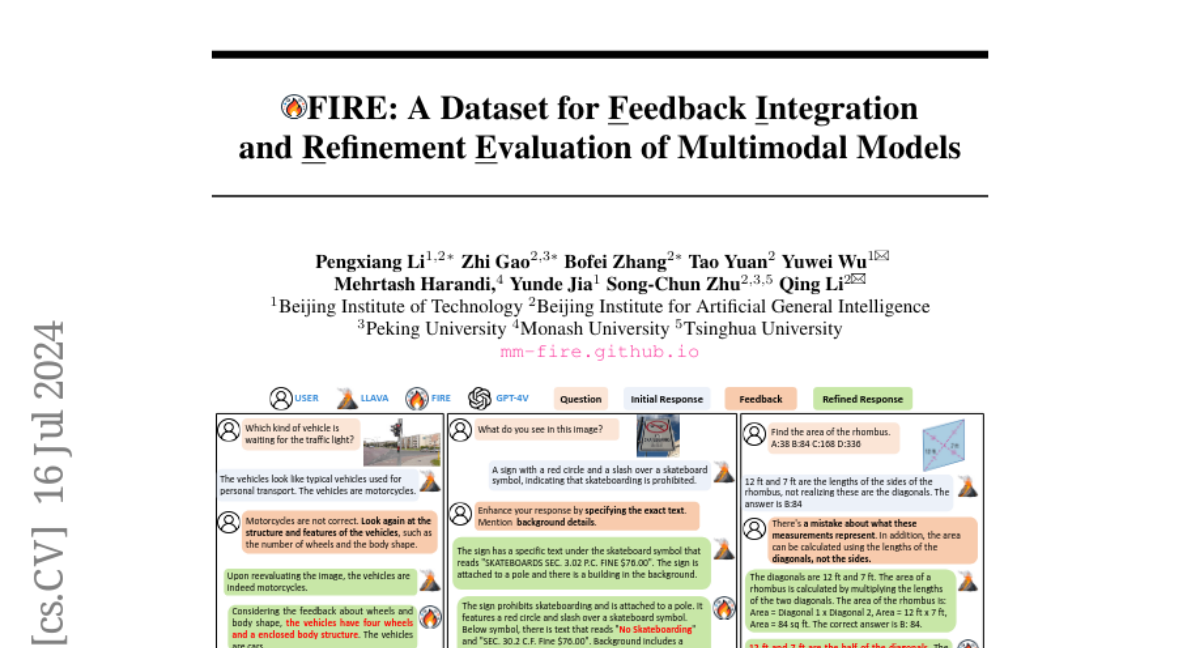
Summary
This paper introduces FIRE, a new dataset designed to help evaluate how well vision language models (VLMs) can improve their responses based on user feedback through multi-turn conversations.
What's the problem?
Existing models that combine text and images often struggle to learn from feedback effectively. They need to be able to refine their answers over time, but there hasn't been a standardized way to test how well they can do this, especially when it comes to understanding complex tasks that involve both visual and textual information.
What's the solution?
To address this issue, the researchers created the FIRE dataset, which includes 1.1 million conversations derived from 27 different sources. This dataset allows VLMs to practice refining their responses based on user feedback. They also developed FIRE-Bench, a benchmark that contains 11,000 conversations specifically designed to evaluate how well VLMs can integrate feedback. Additionally, they fine-tuned a model called FIRE-LLaVA using this dataset, which showed significant improvements in its ability to refine answers compared to untrained models.
Why it matters?
This research is important because it provides a valuable resource for evaluating and improving AI systems that work with both images and text. By focusing on feedback integration, the FIRE dataset can help enhance the performance of VLMs in real-world applications, such as virtual assistants or educational tools, where understanding and responding to user input is crucial.
Abstract
Vision language models (VLMs) have achieved impressive progress in diverse applications, becoming a prevalent research direction. In this paper, we build FIRE, a feedback-refinement dataset, consisting of 1.1M multi-turn conversations that are derived from 27 source datasets, empowering VLMs to spontaneously refine their responses based on user feedback across diverse tasks. To scale up the data collection, FIRE is collected in two components: FIRE-100K and FIRE-1M, where FIRE-100K is generated by GPT-4V, and FIRE-1M is freely generated via models trained on FIRE-100K. Then, we build FIRE-Bench, a benchmark to comprehensively evaluate the feedback-refining capability of VLMs, which contains 11K feedback-refinement conversations as the test data, two evaluation settings, and a model to provide feedback for VLMs. We develop the FIRE-LLaVA model by fine-tuning LLaVA on FIRE-100K and FIRE-1M, which shows remarkable feedback-refining capability on FIRE-Bench and outperforms untrained VLMs by 50%, making more efficient user-agent interactions and underscoring the significance of the FIRE dataset.