Flash-VStream: Memory-Based Real-Time Understanding for Long Video Streams
Haoji Zhang, Yiqin Wang, Yansong Tang, Yong Liu, Jiashi Feng, Jifeng Dai, Xiaojie Jin
2024-07-08
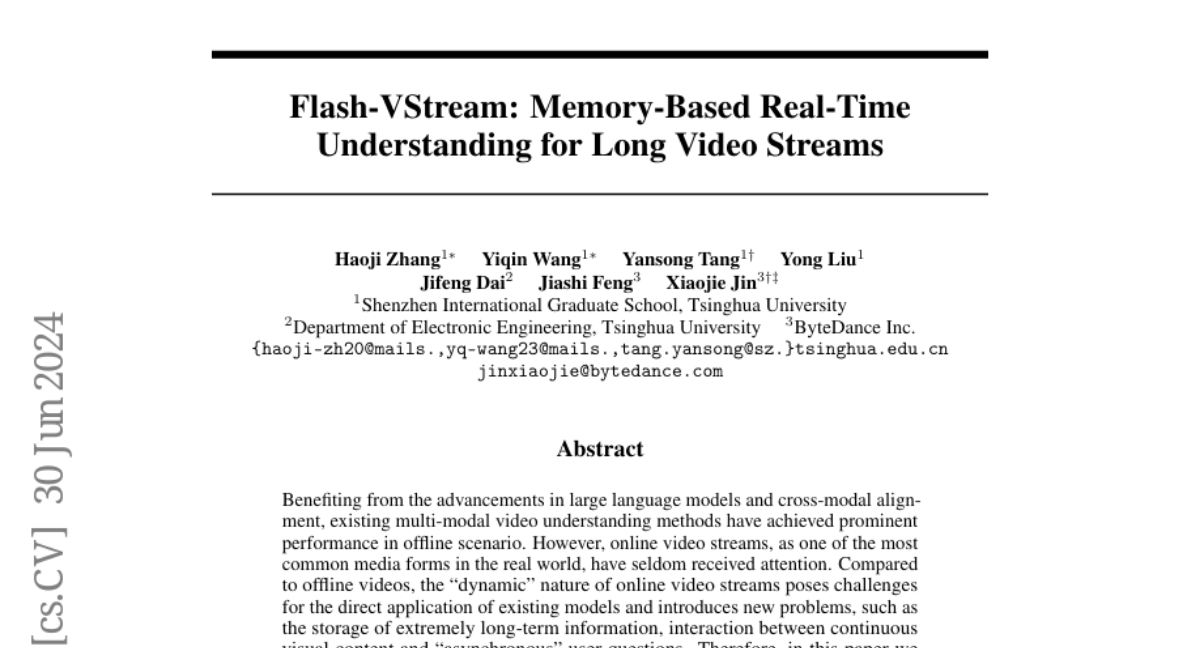
Summary
This paper talks about Flash-VStream, a new video-language model designed to understand and process long video streams in real-time, similar to how humans remember and respond to information while watching videos.
What's the problem?
The main problem is that most existing models for understanding videos work well only with offline videos, where everything is already recorded. However, online video streams are dynamic and constantly changing, making it difficult for these models to keep track of long-term information and respond to user questions in real-time. This leads to challenges in maintaining a coherent understanding of the video content as it unfolds.
What's the solution?
To address these issues, the authors developed Flash-VStream, which simulates human memory to effectively process long video streams while responding to user queries. This model can store important information over time and retrieve it when needed, allowing for better interactions with the content. Additionally, they introduced a new benchmark called VStream-QA specifically for evaluating how well models understand online video streams. Flash-VStream has been shown to perform better than existing methods in both online and offline scenarios.
Why it matters?
This research is important because it enhances the ability of AI systems to understand and interact with real-time video content, which is increasingly common in our daily lives. By improving how models process and remember information from long videos, Flash-VStream can be useful in various applications such as video analysis, live streaming interactions, and educational tools, making technology more responsive and effective.
Abstract
Benefiting from the advancements in large language models and cross-modal alignment, existing multi-modal video understanding methods have achieved prominent performance in offline scenario. However, online video streams, as one of the most common media forms in the real world, have seldom received attention. Compared to offline videos, the 'dynamic' nature of online video streams poses challenges for the direct application of existing models and introduces new problems, such as the storage of extremely long-term information, interaction between continuous visual content and 'asynchronous' user questions. Therefore, in this paper we present Flash-VStream, a video-language model that simulates the memory mechanism of human. Our model is able to process extremely long video streams in real-time and respond to user queries simultaneously. Compared to existing models, Flash-VStream achieves significant reductions in inference latency and VRAM consumption, which is intimately related to performing understanding of online streaming video. In addition, given that existing video understanding benchmarks predominantly concentrate on offline scenario, we propose VStream-QA, a novel question answering benchmark specifically designed for online video streaming understanding. Comparisons with popular existing methods on the proposed benchmark demonstrate the superiority of our method for such challenging setting. To verify the generalizability of our approach, we further evaluate it on existing video understanding benchmarks and achieves state-of-the-art performance in offline scenarios as well. All code, models, and datasets are available at the https://invinciblewyq.github.io/vstream-page/