Flowing from Words to Pixels: A Framework for Cross-Modality Evolution
Qihao Liu, Xi Yin, Alan Yuille, Andrew Brown, Mannat Singh
2024-12-20
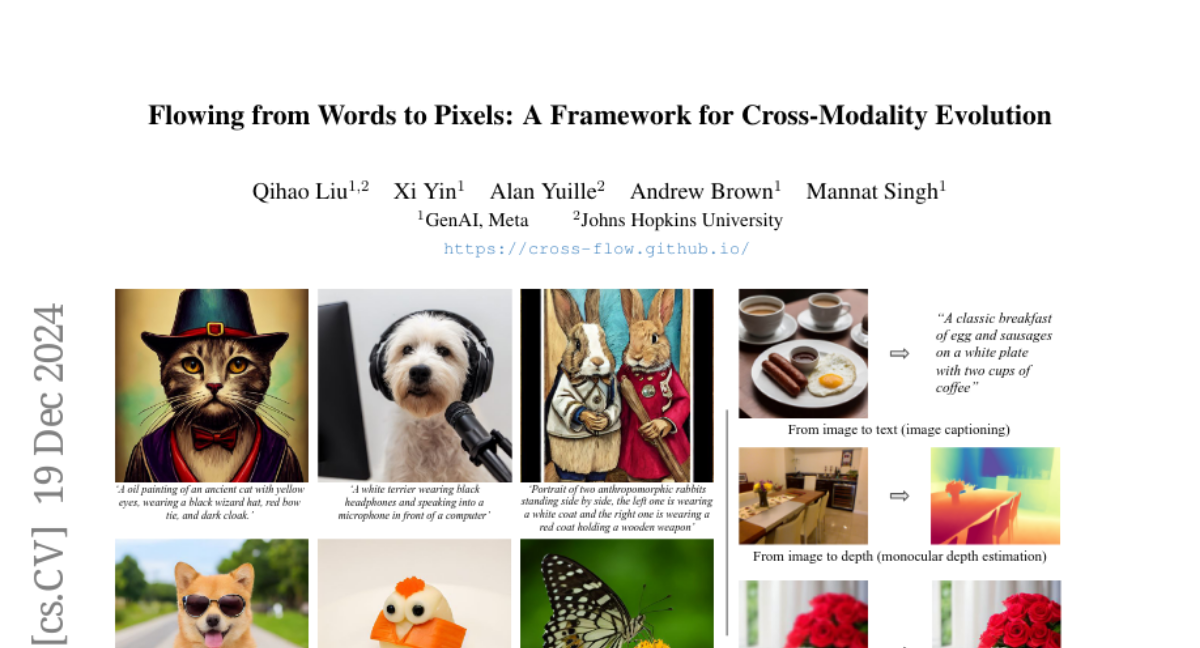
Summary
This paper presents CrossFlow, a new framework that allows models to directly convert text into images without needing to start from random noise. It focuses on improving how AI can understand and generate media across different types, like turning written descriptions into pictures.
What's the problem?
Traditional methods for generating images from text often rely on complex processes that start with random noise and require additional steps to condition the model. This can make the process inefficient and complicated, limiting the model's ability to produce high-quality results.
What's the solution?
CrossFlow simplifies this by training models to learn a direct relationship between text and images. Instead of starting with noise, it uses Variational Encoders to convert text into a format that can be directly transformed into images. The framework also introduces techniques that allow for interesting edits in the generated images, making it easier to create variations based on simple adjustments. The results show that CrossFlow performs as well as or better than existing methods in various tasks, including generating images from text and enhancing image quality.
Why it matters?
This research is significant because it streamlines the process of creating images from text, making it more efficient and effective. By improving cross-modal generation (the ability to connect different types of data), CrossFlow could enhance applications in art, design, and other fields where visual content is created based on written descriptions.
Abstract
Diffusion models, and their generalization, flow matching, have had a remarkable impact on the field of media generation. Here, the conventional approach is to learn the complex mapping from a simple source distribution of Gaussian noise to the target media distribution. For cross-modal tasks such as text-to-image generation, this same mapping from noise to image is learnt whilst including a conditioning mechanism in the model. One key and thus far relatively unexplored feature of flow matching is that, unlike Diffusion models, they are not constrained for the source distribution to be noise. Hence, in this paper, we propose a paradigm shift, and ask the question of whether we can instead train flow matching models to learn a direct mapping from the distribution of one modality to the distribution of another, thus obviating the need for both the noise distribution and conditioning mechanism. We present a general and simple framework, CrossFlow, for cross-modal flow matching. We show the importance of applying Variational Encoders to the input data, and introduce a method to enable Classifier-free guidance. Surprisingly, for text-to-image, CrossFlow with a vanilla transformer without cross attention slightly outperforms standard flow matching, and we show that it scales better with training steps and model size, while also allowing for interesting latent arithmetic which results in semantically meaningful edits in the output space. To demonstrate the generalizability of our approach, we also show that CrossFlow is on par with or outperforms the state-of-the-art for various cross-modal / intra-modal mapping tasks, viz. image captioning, depth estimation, and image super-resolution. We hope this paper contributes to accelerating progress in cross-modal media generation.