FontStudio: Shape-Adaptive Diffusion Model for Coherent and Consistent Font Effect Generation
Xinzhi Mu, Li Chen, Bohan Chen, Shuyang Gu, Jianmin Bao, Dong Chen, Ji Li, Yuhui Yuan
2024-06-13
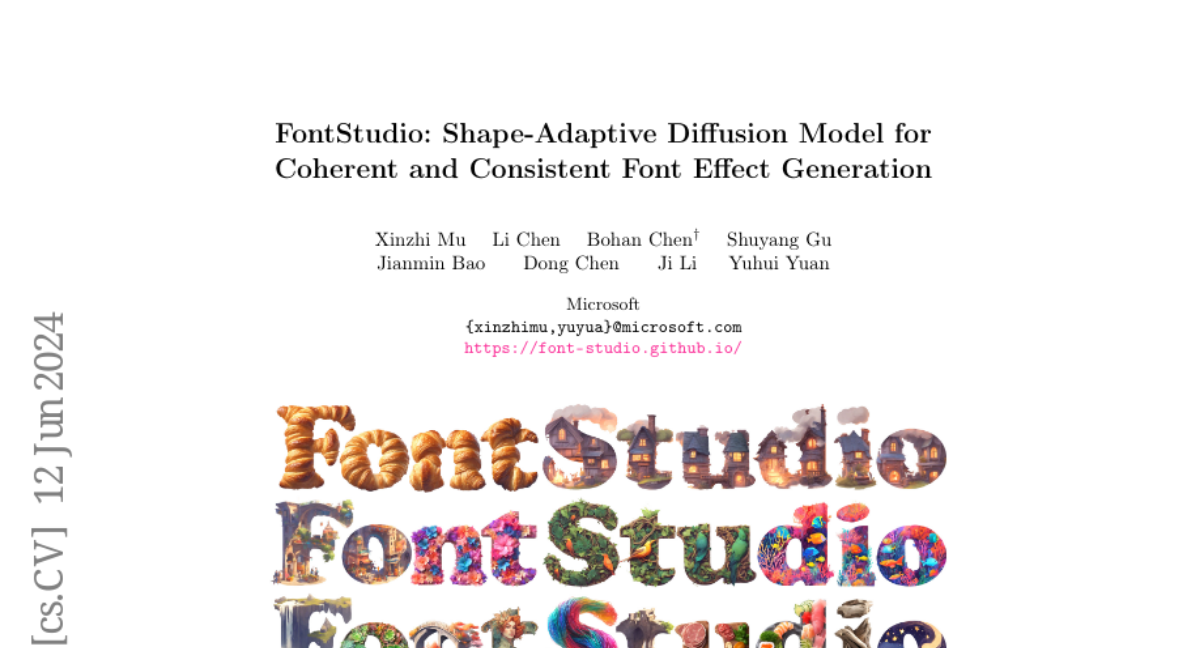
Summary
This paper introduces FontStudio, a new model designed to create artistic font effects that are coherent and consistent, especially for multilingual fonts. It uses advanced techniques to adapt the design process to the unique shapes of different fonts.
What's the problem?
Creating visually appealing font effects has traditionally been the job of professional designers, and existing methods often struggle with consistency and coherence, particularly when dealing with different languages or irregular shapes. Most models focus on generating effects in standard rectangular spaces, which doesn't suit the unique shapes of various fonts.
What's the solution?
FontStudio addresses this issue by using a shape-adaptive diffusion model that can understand and work with the specific shapes of fonts. It generates text effects by planning how to distribute pixels within the irregular canvas created by the font shape. The model also incorporates a high-quality dataset and uses segmentation masks to guide the generation process. Additionally, it features a training-free method for transferring textures from one letter to another, ensuring that all letters maintain a consistent look. User studies showed that FontStudio was preferred over other tools, including Adobe Firefly, for its aesthetic quality.
Why it matters?
This research is significant because it enhances the ability to create high-quality, artistic fonts that can be used in various languages. By improving how font effects are generated, FontStudio opens up new possibilities for designers and artists, making it easier to produce visually stunning text that fits diverse styles and applications.
Abstract
Recently, the application of modern diffusion-based text-to-image generation models for creating artistic fonts, traditionally the domain of professional designers, has garnered significant interest. Diverging from the majority of existing studies that concentrate on generating artistic typography, our research aims to tackle a novel and more demanding challenge: the generation of text effects for multilingual fonts. This task essentially requires generating coherent and consistent visual content within the confines of a font-shaped canvas, as opposed to a traditional rectangular canvas. To address this task, we introduce a novel shape-adaptive diffusion model capable of interpreting the given shape and strategically planning pixel distributions within the irregular canvas. To achieve this, we curate a high-quality shape-adaptive image-text dataset and incorporate the segmentation mask as a visual condition to steer the image generation process within the irregular-canvas. This approach enables the traditionally rectangle canvas-based diffusion model to produce the desired concepts in accordance with the provided geometric shapes. Second, to maintain consistency across multiple letters, we also present a training-free, shape-adaptive effect transfer method for transferring textures from a generated reference letter to others. The key insights are building a font effect noise prior and propagating the font effect information in a concatenated latent space. The efficacy of our FontStudio system is confirmed through user preference studies, which show a marked preference (78% win-rates on aesthetics) for our system even when compared to the latest unrivaled commercial product, Adobe Firefly.