Foundational Autoraters: Taming Large Language Models for Better Automatic Evaluation
Tu Vu, Kalpesh Krishna, Salaheddin Alzubi, Chris Tar, Manaal Faruqui, Yun-Hsuan Sung
2024-07-16
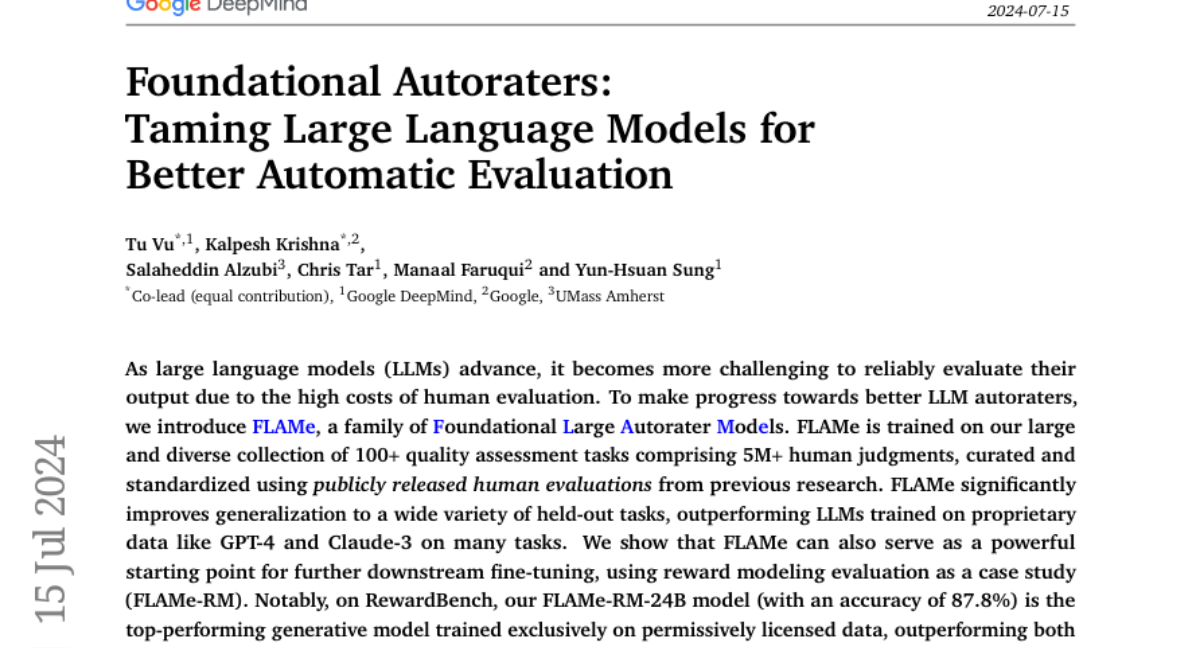
Summary
This paper presents FLAMe, a new type of model designed to automatically evaluate the quality of text generated by large language models (LLMs), improving how we assess their performance.
What's the problem?
Evaluating the outputs of large language models can be very expensive and time-consuming if done by humans. Current methods often rely on proprietary data that may not generalize well across different tasks, leading to inconsistent evaluations and making it hard to determine how well these models truly perform.
What's the solution?
FLAMe is trained on a large and diverse dataset that includes over 5 million human judgments across 100+ quality assessment tasks. This training helps FLAMe to better evaluate LLM outputs compared to previous models. The paper shows that FLAMe outperforms other well-known models like GPT-4 on various evaluation benchmarks, particularly in reward modeling tasks. Additionally, a new technique called tail-patch fine-tuning is introduced, which allows for efficient training with fewer data points while still achieving strong performance.
Why it matters?
This research is important because it provides a more effective and efficient way to evaluate large language models. By using FLAMe, developers can get better insights into how their models perform across different tasks without the high costs of human evaluation. This could lead to improvements in AI systems used for writing, customer service, and many other applications, ultimately enhancing the quality of interactions people have with AI.
Abstract
As large language models (LLMs) advance, it becomes more challenging to reliably evaluate their output due to the high costs of human evaluation. To make progress towards better LLM autoraters, we introduce FLAMe, a family of Foundational Large Autorater Models. FLAMe is trained on our large and diverse collection of 100+ quality assessment tasks comprising 5M+ human judgments, curated and standardized using publicly released human evaluations from previous research. FLAMe significantly improves generalization to a wide variety of held-out tasks, outperforming LLMs trained on proprietary data like GPT-4 and Claude-3 on many tasks. We show that FLAMe can also serve as a powerful starting point for further downstream fine-tuning, using reward modeling evaluation as a case study (FLAMe-RM). Notably, on RewardBench, our FLAMe-RM-24B model (with an accuracy of 87.8%) is the top-performing generative model trained exclusively on permissively licensed data, outperforming both GPT-4-0125 (85.9%) and GPT-4o (84.7%). Additionally, we explore a more computationally efficient approach using a novel tail-patch fine-tuning strategy to optimize our FLAMe multitask mixture for reward modeling evaluation (FLAMe-Opt-RM), offering competitive RewardBench performance while requiring approximately 25x less training datapoints. Overall, our FLAMe variants outperform all popular proprietary LLM-as-a-Judge models we consider across 8 out of 12 autorater evaluation benchmarks, encompassing 53 quality assessment tasks, including RewardBench and LLM-AggreFact. Finally, our analysis reveals that FLAMe is significantly less biased than these LLM-as-a-Judge models on the CoBBLEr autorater bias benchmark, while effectively identifying high-quality responses for code generation.