FRAP: Faithful and Realistic Text-to-Image Generation with Adaptive Prompt Weighting
Liyao Jiang, Negar Hassanpour, Mohammad Salameh, Mohan Sai Singamsetti, Fengyu Sun, Wei Lu, Di Niu
2024-08-22
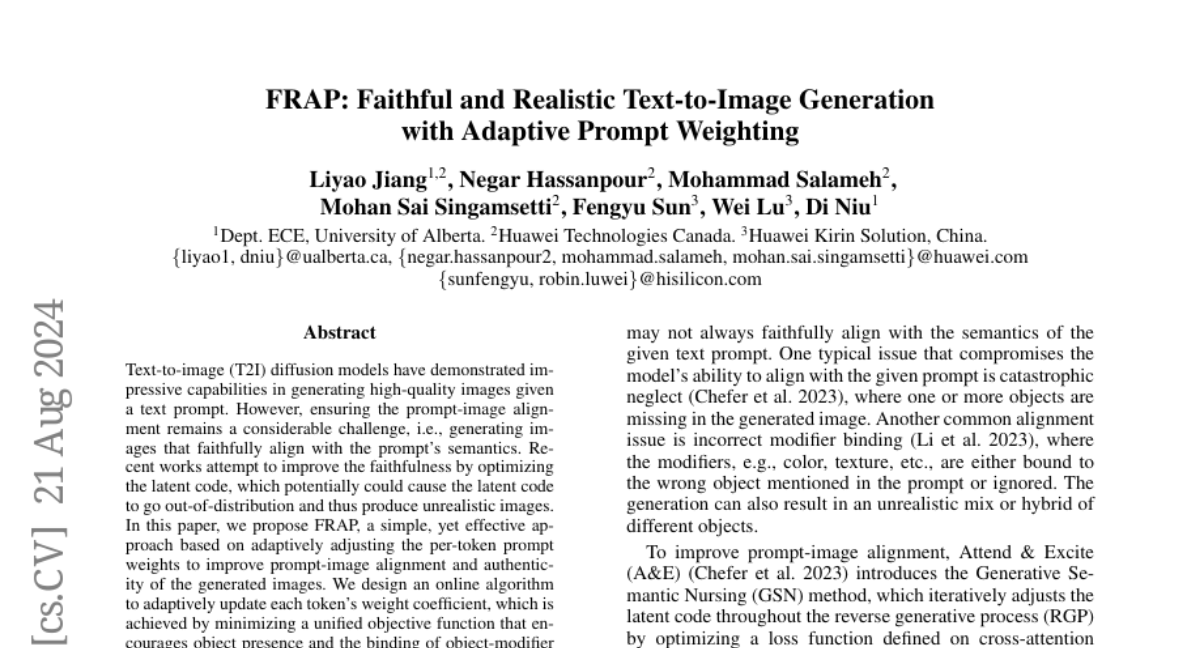
Summary
This paper discusses FRAP, a new method for generating images from text prompts that ensures the images closely match what the prompts describe.
What's the problem?
When creating images from text using AI, it can be difficult to ensure that the images accurately reflect the details and ideas in the text. Some methods that try to improve this can lead to unrealistic images because they change too much about the original data.
What's the solution?
The authors propose a method called FRAP, which adjusts the importance of different parts of the text prompt to improve how well the generated images align with the prompts. They use a smart algorithm that updates these importance weights while focusing on keeping relevant details intact. This helps produce images that are not only more accurate but also realistic-looking, and it does so faster than some previous methods.
Why it matters?
This research is important because it helps improve the quality of AI-generated images, making them more useful for applications like art creation, advertising, and education. By ensuring that generated images are faithful to their prompts, it enhances the overall effectiveness of text-to-image technology.
Abstract
Text-to-image (T2I) diffusion models have demonstrated impressive capabilities in generating high-quality images given a text prompt. However, ensuring the prompt-image alignment remains a considerable challenge, i.e., generating images that faithfully align with the prompt's semantics. Recent works attempt to improve the faithfulness by optimizing the latent code, which potentially could cause the latent code to go out-of-distribution and thus produce unrealistic images. In this paper, we propose FRAP, a simple, yet effective approach based on adaptively adjusting the per-token prompt weights to improve prompt-image alignment and authenticity of the generated images. We design an online algorithm to adaptively update each token's weight coefficient, which is achieved by minimizing a unified objective function that encourages object presence and the binding of object-modifier pairs. Through extensive evaluations, we show FRAP generates images with significantly higher prompt-image alignment to prompts from complex datasets, while having a lower average latency compared to recent latent code optimization methods, e.g., 4 seconds faster than D&B on the COCO-Subject dataset. Furthermore, through visual comparisons and evaluation on the CLIP-IQA-Real metric, we show that FRAP not only improves prompt-image alignment but also generates more authentic images with realistic appearances. We also explore combining FRAP with prompt rewriting LLM to recover their degraded prompt-image alignment, where we observe improvements in both prompt-image alignment and image quality.