FreeScale: Unleashing the Resolution of Diffusion Models via Tuning-Free Scale Fusion
Haonan Qiu, Shiwei Zhang, Yujie Wei, Ruihang Chu, Hangjie Yuan, Xiang Wang, Yingya Zhang, Ziwei Liu
2024-12-16
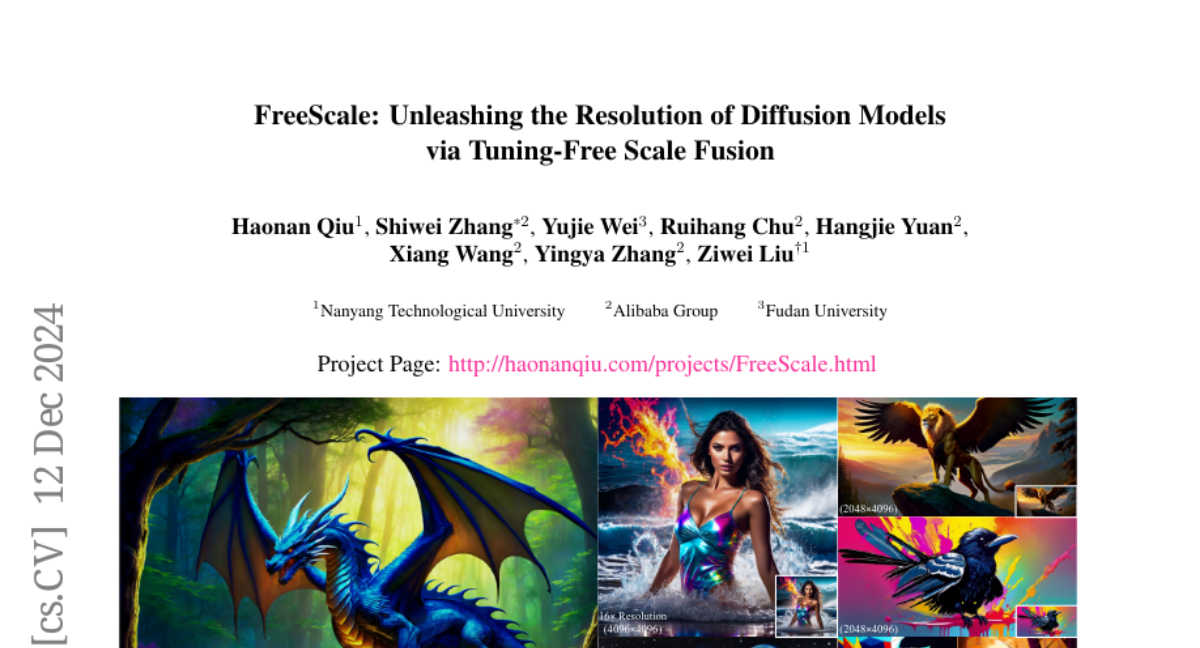
Summary
This paper talks about FreeScale, a new method that improves how AI models generate high-resolution images and videos without needing complex adjustments.
What's the problem?
Many AI models that create images or videos are limited by the resolution they were trained on, which means they can't produce very detailed content. When these models try to generate higher-resolution visuals, they often end up creating low-quality images with repetitive patterns due to errors that build up during the process.
What's the solution?
FreeScale addresses this problem by using a technique called scale fusion, which allows the model to combine information from different levels of detail. Instead of just trying to generate everything at once, FreeScale processes various scales of information and merges them effectively. This method enables the generation of high-quality visuals at resolutions as high as 8k for the first time, while maintaining fast processing speeds and reducing errors.
Why it matters?
This research is important because it enhances the capabilities of AI in creating realistic and detailed images and videos. By unlocking the potential for higher-resolution generation, FreeScale can benefit industries like film, gaming, and virtual reality, where high-quality visuals are essential for engaging experiences.
Abstract
Visual diffusion models achieve remarkable progress, yet they are typically trained at limited resolutions due to the lack of high-resolution data and constrained computation resources, hampering their ability to generate high-fidelity images or videos at higher resolutions. Recent efforts have explored tuning-free strategies to exhibit the untapped potential higher-resolution visual generation of pre-trained models. However, these methods are still prone to producing low-quality visual content with repetitive patterns. The key obstacle lies in the inevitable increase in high-frequency information when the model generates visual content exceeding its training resolution, leading to undesirable repetitive patterns deriving from the accumulated errors. To tackle this challenge, we propose FreeScale, a tuning-free inference paradigm to enable higher-resolution visual generation via scale fusion. Specifically, FreeScale processes information from different receptive scales and then fuses it by extracting desired frequency components. Extensive experiments validate the superiority of our paradigm in extending the capabilities of higher-resolution visual generation for both image and video models. Notably, compared with the previous best-performing method, FreeScale unlocks the generation of 8k-resolution images for the first time.