FreeTraj: Tuning-Free Trajectory Control in Video Diffusion Models
Haonan Qiu, Zhaoxi Chen, Zhouxia Wang, Yingqing He, Menghan Xia, Ziwei Liu
2024-06-26
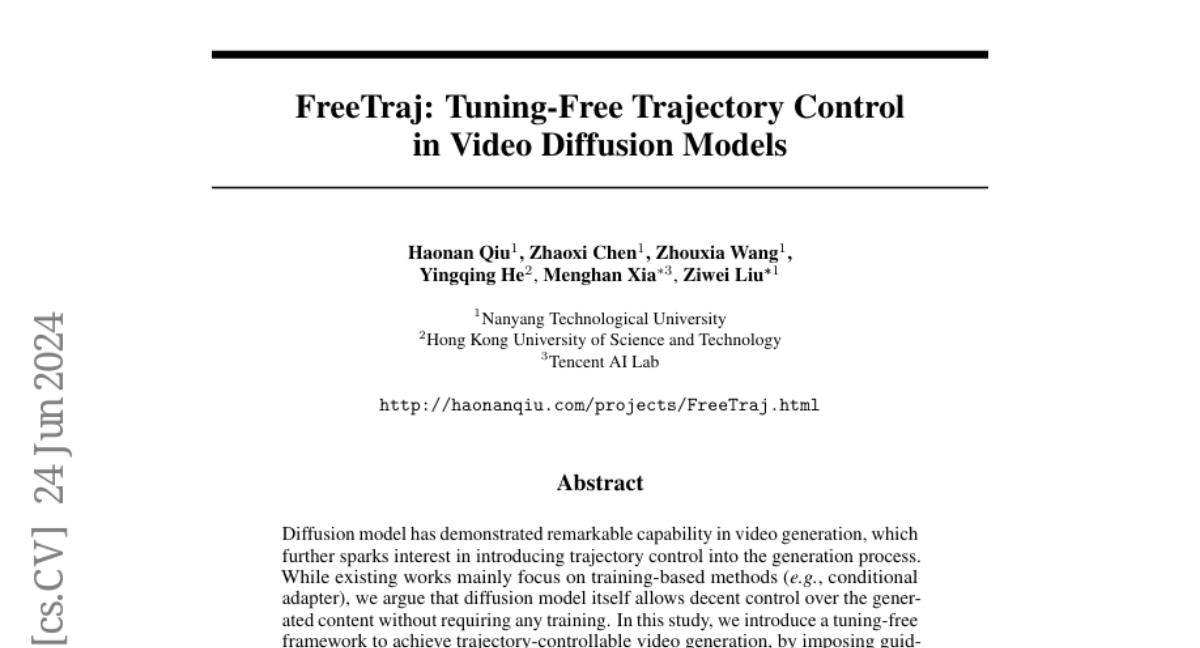
Summary
This paper introduces FreeTraj, a new method for generating videos that allows users to control the movement of objects within those videos without needing to retrain the model. It focuses on how to guide the video creation process using noise and attention mechanisms.
What's the problem?
Many existing methods for generating videos require extensive training or adjustments to control how objects move. This can be time-consuming and complicated, especially when users want specific movements or trajectories in their videos. Additionally, controlling these movements often relies on techniques that may not be flexible or efficient.
What's the solution?
The authors propose FreeTraj, which simplifies the process by allowing users to control the motion of objects in videos without the need for additional training. They explain how initial noise affects the movement of objects and introduce a framework that modifies how noise is sampled and how attention is applied during video generation. This method enables users to either manually set the desired trajectories or use an automatic planner to generate them. FreeTraj also supports creating longer videos with controlled movements.
Why it matters?
This research is important because it makes video generation more accessible and flexible for users who want specific control over how objects move in their videos. By reducing the need for complex training processes, FreeTraj can help creators in fields like animation, gaming, and virtual reality produce high-quality content more efficiently.
Abstract
Diffusion model has demonstrated remarkable capability in video generation, which further sparks interest in introducing trajectory control into the generation process. While existing works mainly focus on training-based methods (e.g., conditional adapter), we argue that diffusion model itself allows decent control over the generated content without requiring any training. In this study, we introduce a tuning-free framework to achieve trajectory-controllable video generation, by imposing guidance on both noise construction and attention computation. Specifically, 1) we first show several instructive phenomenons and analyze how initial noises influence the motion trajectory of generated content. 2) Subsequently, we propose FreeTraj, a tuning-free approach that enables trajectory control by modifying noise sampling and attention mechanisms. 3) Furthermore, we extend FreeTraj to facilitate longer and larger video generation with controllable trajectories. Equipped with these designs, users have the flexibility to provide trajectories manually or opt for trajectories automatically generated by the LLM trajectory planner. Extensive experiments validate the efficacy of our approach in enhancing the trajectory controllability of video diffusion models.