Frontiers in Intelligent Colonoscopy
Ge-Peng Ji, Jingyi Liu, Peng Xu, Nick Barnes, Fahad Shahbaz Khan, Salman Khan, Deng-Ping Fan
2024-10-23
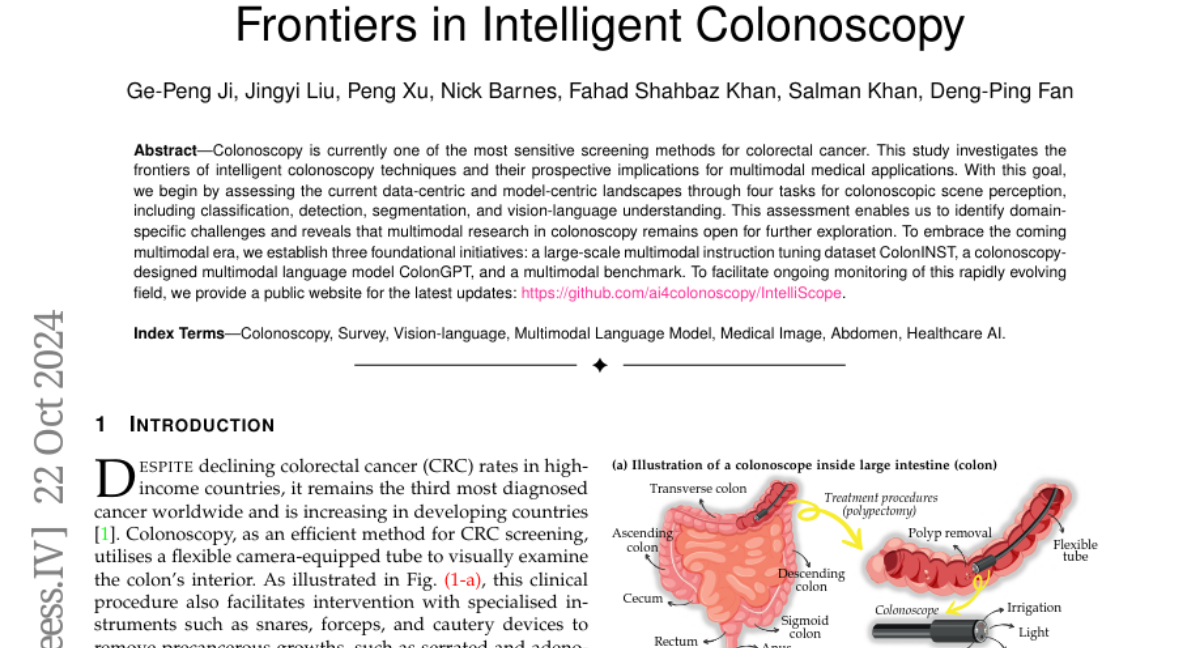
Summary
This paper discusses advancements in intelligent colonoscopy techniques aimed at improving the detection and treatment of colorectal cancer through better data analysis and artificial intelligence.
What's the problem?
Colonoscopy is a critical procedure for screening colorectal cancer, but current methods can be limited in their effectiveness. There are challenges in accurately interpreting the complex images produced during colonoscopy, which can lead to missed diagnoses and ineffective treatments.
What's the solution?
The authors propose several initiatives to enhance colonoscopy techniques, including creating a large dataset called ColonINST for training AI models, developing a multimodal language model named ColonGPT to assist doctors during procedures, and establishing a benchmark for evaluating these technologies. These tools aim to improve how well AI can understand and analyze colonoscopic images.
Why it matters?
This research is important because it could lead to more accurate and efficient colonoscopy procedures. By integrating advanced AI techniques, doctors can better identify precancerous growths, ultimately improving patient outcomes and reducing the incidence of colorectal cancer.
Abstract
Colonoscopy is currently one of the most sensitive screening methods for colorectal cancer. This study investigates the frontiers of intelligent colonoscopy techniques and their prospective implications for multimodal medical applications. With this goal, we begin by assessing the current data-centric and model-centric landscapes through four tasks for colonoscopic scene perception, including classification, detection, segmentation, and vision-language understanding. This assessment enables us to identify domain-specific challenges and reveals that multimodal research in colonoscopy remains open for further exploration. To embrace the coming multimodal era, we establish three foundational initiatives: a large-scale multimodal instruction tuning dataset ColonINST, a colonoscopy-designed multimodal language model ColonGPT, and a multimodal benchmark. To facilitate ongoing monitoring of this rapidly evolving field, we provide a public website for the latest updates: https://github.com/ai4colonoscopy/IntelliScope.