FrugalNeRF: Fast Convergence for Few-shot Novel View Synthesis without Learned Priors
Chin-Yang Lin, Chung-Ho Wu, Chang-Han Yeh, Shih-Han Yen, Cheng Sun, Yu-Lun Liu
2024-10-22
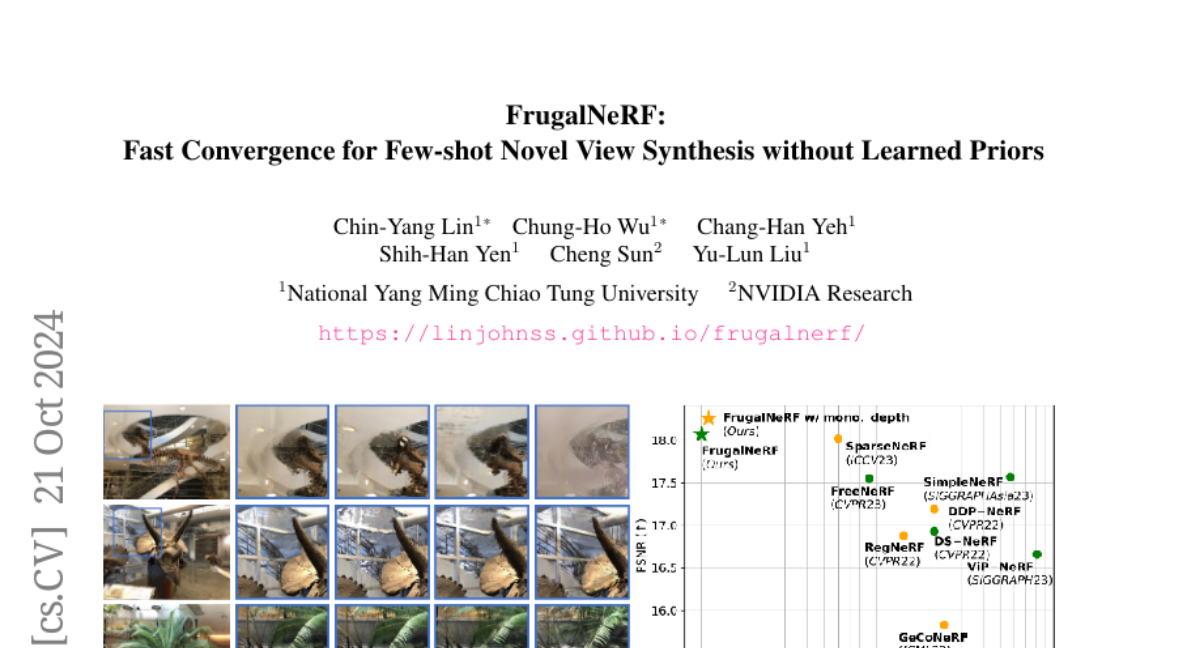
Summary
This paper introduces FrugalNeRF, a new framework designed to quickly and effectively generate high-quality 3D images from just a few input images, overcoming challenges faced by existing methods.
What's the problem?
When trying to create detailed 3D images using Neural Radiance Fields (NeRF) with only a few images, models often struggle due to overfitting and long training times. Current methods can be complex and may rely on pre-trained data, which can limit their effectiveness in generating accurate representations of scenes. This makes it difficult to produce high-quality images quickly in situations where only a few shots are available.
What's the solution?
To address these challenges, the authors developed FrugalNeRF, which uses a method of sharing weights across different scales to better represent scene details. They introduced a technique called cross-scale geometric adaptation that helps the model learn from the depth of images without needing external data. This allows FrugalNeRF to make the most of the limited training data it has. The authors tested FrugalNeRF on various datasets and found that it outperformed existing few-shot NeRF methods while also reducing the time needed for training.
Why it matters?
This research is important because it provides a more efficient way to generate high-quality 3D images from limited input data. By improving how models learn from fewer images, FrugalNeRF can be applied in many areas such as virtual reality, gaming, and architecture, where quick and accurate visualizations are essential.
Abstract
Neural Radiance Fields (NeRF) face significant challenges in few-shot scenarios, primarily due to overfitting and long training times for high-fidelity rendering. Existing methods, such as FreeNeRF and SparseNeRF, use frequency regularization or pre-trained priors but struggle with complex scheduling and bias. We introduce FrugalNeRF, a novel few-shot NeRF framework that leverages weight-sharing voxels across multiple scales to efficiently represent scene details. Our key contribution is a cross-scale geometric adaptation scheme that selects pseudo ground truth depth based on reprojection errors across scales. This guides training without relying on externally learned priors, enabling full utilization of the training data. It can also integrate pre-trained priors, enhancing quality without slowing convergence. Experiments on LLFF, DTU, and RealEstate-10K show that FrugalNeRF outperforms other few-shot NeRF methods while significantly reducing training time, making it a practical solution for efficient and accurate 3D scene reconstruction.