Futga: Towards Fine-grained Music Understanding through Temporally-enhanced Generative Augmentation
Junda Wu, Zachary Novack, Amit Namburi, Jiaheng Dai, Hao-Wen Dong, Zhouhang Xie, Carol Chen, Julian McAuley
2024-07-31
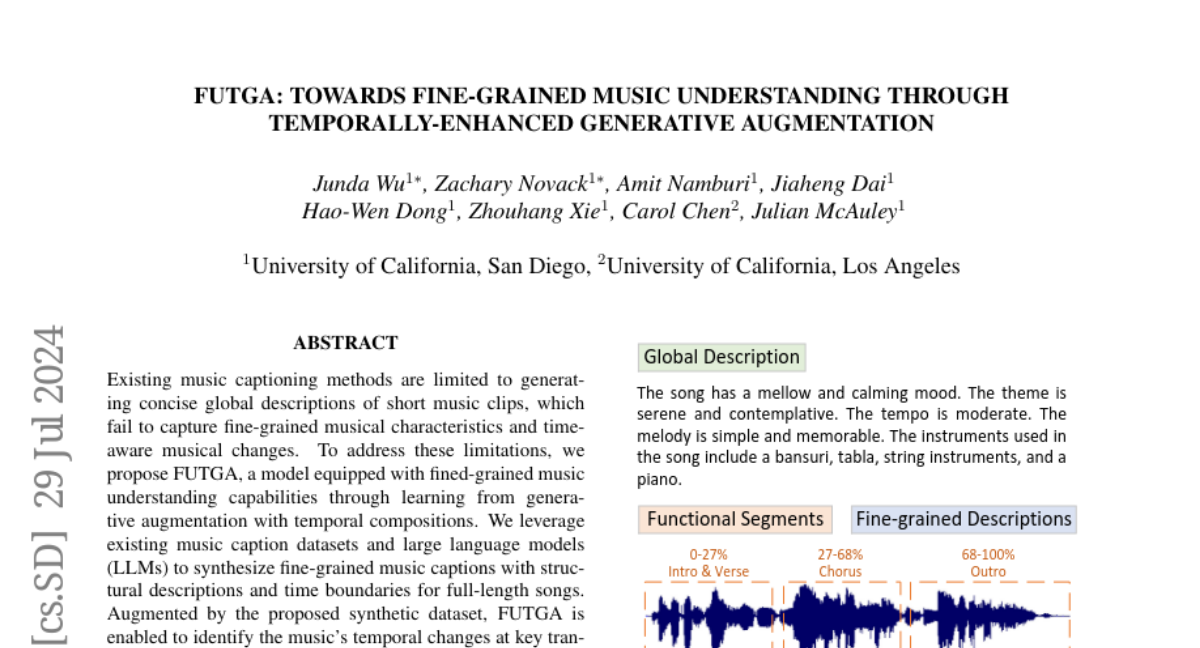
Summary
This paper introduces FUTGA, a new model designed to improve music understanding by generating detailed captions for full-length songs. It focuses on capturing the intricate details and changes in music over time.
What's the problem?
Current methods for music captioning mainly provide simple, general descriptions of short music clips. These methods often miss important details about the music's characteristics and how they change throughout a song, leading to a lack of depth in understanding musical pieces.
What's the solution?
To address these limitations, the authors developed FUTGA, which uses advanced techniques to create fine-grained music captions. By leveraging existing datasets and large language models, FUTGA generates detailed descriptions that include structural information and time markers for different segments of a song. This allows it to identify key transitions and musical functions, providing a richer understanding of the music.
Why it matters?
This research is significant because it enhances how we interact with and understand music. By generating more detailed captions, FUTGA can improve applications like music retrieval and generation, making it easier for listeners to find and appreciate music based on its specific characteristics. This could lead to better tools for musicians, educators, and anyone interested in exploring music in greater detail.
Abstract
Existing music captioning methods are limited to generating concise global descriptions of short music clips, which fail to capture fine-grained musical characteristics and time-aware musical changes. To address these limitations, we propose FUTGA, a model equipped with fined-grained music understanding capabilities through learning from generative augmentation with temporal compositions. We leverage existing music caption datasets and large language models (LLMs) to synthesize fine-grained music captions with structural descriptions and time boundaries for full-length songs. Augmented by the proposed synthetic dataset, FUTGA is enabled to identify the music's temporal changes at key transition points and their musical functions, as well as generate detailed descriptions for each music segment. We further introduce a full-length music caption dataset generated by FUTGA, as the augmentation of the MusicCaps and the Song Describer datasets. We evaluate the automatically generated captions on several downstream tasks, including music generation and retrieval. The experiments demonstrate the quality of the generated captions and the better performance in various downstream tasks achieved by the proposed music captioning approach. Our code and datasets can be found in https://huggingface.co/JoshuaW1997/FUTGA{blue{https://huggingface.co/JoshuaW1997/FUTGA}}.