GAMA: A Large Audio-Language Model with Advanced Audio Understanding and Complex Reasoning Abilities
Sreyan Ghosh, Sonal Kumar, Ashish Seth, Chandra Kiran Reddy Evuru, Utkarsh Tyagi, S Sakshi, Oriol Nieto, Ramani Duraiswami, Dinesh Manocha
2024-06-18
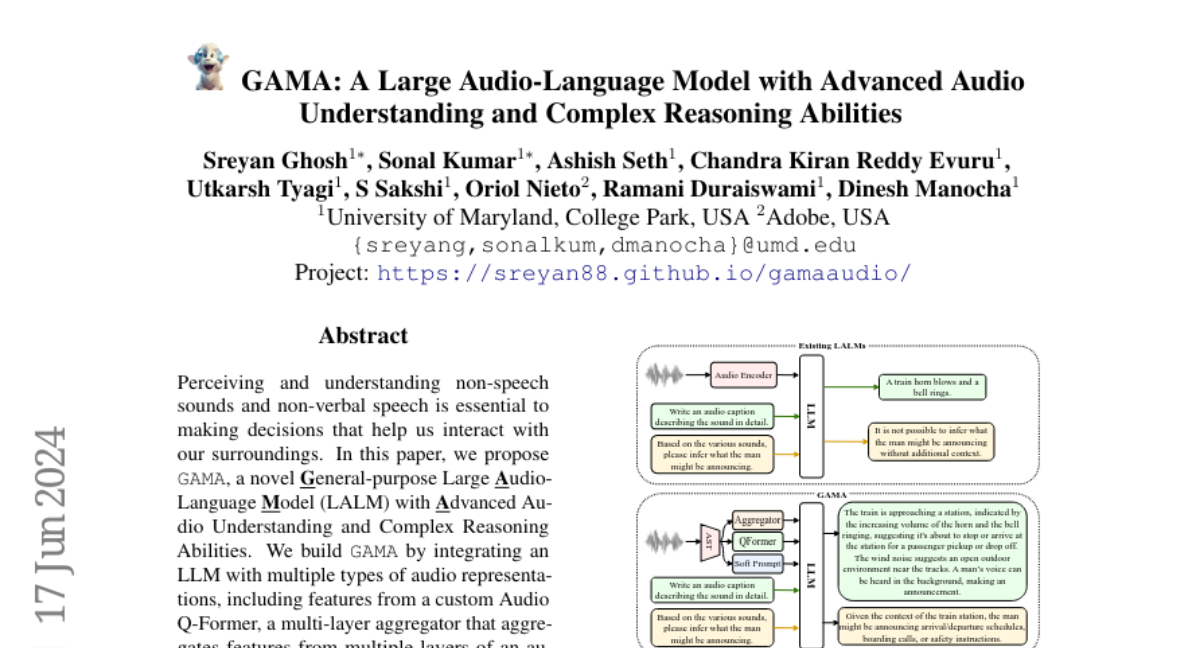
Summary
This paper presents GAMA, a new type of large audio-language model designed to understand and reason about non-speech sounds and non-verbal speech. It combines advanced audio processing with language understanding to interpret various sounds in our environment better.
What's the problem?
Understanding sounds that are not spoken words, like background noises or emotional cues in a baby's cry, is crucial for making decisions in everyday life. However, existing models struggle to interpret these types of audio effectively. They often lack the ability to reason about complex audio inputs, which limits their usefulness in real-world applications where sound plays a significant role.
What's the solution?
To address this issue, the authors developed GAMA by integrating a large language model (LLM) with various audio representations. They created a custom feature extractor called Audio Q-Former to gather detailed information from audio inputs. Additionally, they fine-tuned GAMA on a large dataset specifically designed for audio-language tasks, which included complex reasoning challenges. They also introduced a new dataset called CompA-R for instruction tuning, which helps the model learn how to respond accurately to complex audio scenarios. GAMA was tested against other models and showed significantly better performance in understanding and reasoning about audio.
Why it matters?
This research is important because it enhances how machines can interact with and understand the world through sound. By improving the ability to process non-speech audio, GAMA can be used in various applications, such as assistive technologies for people with hearing impairments, surveillance systems that interpret environmental sounds, and even robots that need to understand their surroundings better. This advancement opens up new possibilities for AI in everyday life.
Abstract
Perceiving and understanding non-speech sounds and non-verbal speech is essential to making decisions that help us interact with our surroundings. In this paper, we propose GAMA, a novel General-purpose Large Audio-Language Model (LALM) with Advanced Audio Understanding and Complex Reasoning Abilities. We build GAMA by integrating an LLM with multiple types of audio representations, including features from a custom Audio Q-Former, a multi-layer aggregator that aggregates features from multiple layers of an audio encoder. We fine-tune GAMA on a large-scale audio-language dataset, which augments it with audio understanding capabilities. Next, we propose CompA-R (Instruction-Tuning for Complex Audio Reasoning), a synthetically generated instruction-tuning (IT) dataset with instructions that require the model to perform complex reasoning on the input audio. We instruction-tune GAMA with CompA-R to endow it with complex reasoning abilities, where we further add a soft prompt as input with high-level semantic evidence by leveraging event tags of the input audio. Finally, we also propose CompA-R-test, a human-labeled evaluation dataset for evaluating the capabilities of LALMs on open-ended audio question-answering that requires complex reasoning. Through automated and expert human evaluations, we show that GAMA outperforms all other LALMs in literature on diverse audio understanding tasks by margins of 1%-84%. Further, GAMA IT-ed on CompA-R proves to be superior in its complex reasoning and instruction following capabilities.