GarVerseLOD: High-Fidelity 3D Garment Reconstruction from a Single In-the-Wild Image using a Dataset with Levels of Details
Zhongjin Luo, Haolin Liu, Chenghong Li, Wanghao Du, Zirong Jin, Wanhu Sun, Yinyu Nie, Weikai Chen, Xiaoguang Han
2024-11-06
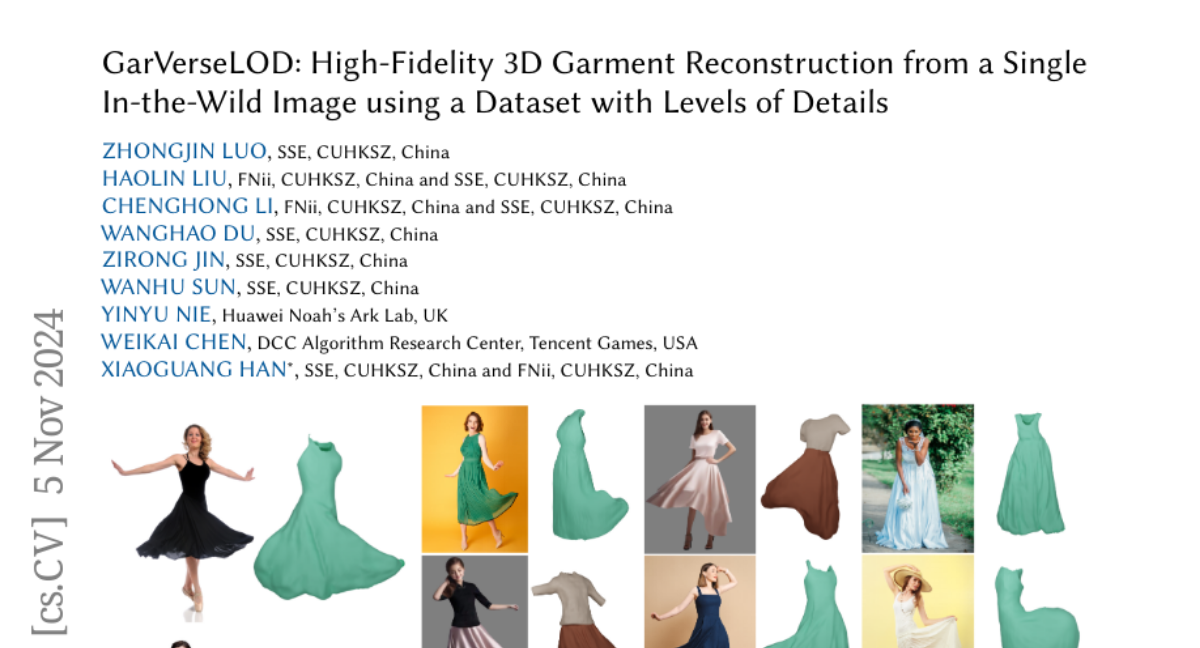
Summary
This paper presents GarVerseLOD, a new dataset and framework for creating high-quality 3D garment models from just one image, even if that image is taken in everyday situations.
What's the problem?
Current methods for creating 3D models of clothing often struggle with complex shapes and poses, especially when using only one image. This makes it difficult to accurately represent garments in 3D, which can lead to poor-quality models that don't look realistic.
What's the solution?
The authors developed GarVerseLOD, which includes a dataset of 6,000 detailed cloth models created by professional artists. They designed the framework to handle different levels of detail in the garment models, allowing the system to focus on simpler tasks first before refining them into more complex shapes. They also used advanced techniques to generate realistic images of garments from their 3D models, ensuring that the system can work well with real-world images.
Why it matters?
This research is important because it improves how we can create realistic 3D models from simple images, which has many applications in fashion design, gaming, and virtual reality. By making it easier to generate high-quality garment representations, it can help designers and developers create better products and experiences.
Abstract
Neural implicit functions have brought impressive advances to the state-of-the-art of clothed human digitization from multiple or even single images. However, despite the progress, current arts still have difficulty generalizing to unseen images with complex cloth deformation and body poses. In this work, we present GarVerseLOD, a new dataset and framework that paves the way to achieving unprecedented robustness in high-fidelity 3D garment reconstruction from a single unconstrained image. Inspired by the recent success of large generative models, we believe that one key to addressing the generalization challenge lies in the quantity and quality of 3D garment data. Towards this end, GarVerseLOD collects 6,000 high-quality cloth models with fine-grained geometry details manually created by professional artists. In addition to the scale of training data, we observe that having disentangled granularities of geometry can play an important role in boosting the generalization capability and inference accuracy of the learned model. We hence craft GarVerseLOD as a hierarchical dataset with levels of details (LOD), spanning from detail-free stylized shape to pose-blended garment with pixel-aligned details. This allows us to make this highly under-constrained problem tractable by factorizing the inference into easier tasks, each narrowed down with smaller searching space. To ensure GarVerseLOD can generalize well to in-the-wild images, we propose a novel labeling paradigm based on conditional diffusion models to generate extensive paired images for each garment model with high photorealism. We evaluate our method on a massive amount of in-the-wild images. Experimental results demonstrate that GarVerseLOD can generate standalone garment pieces with significantly better quality than prior approaches. Project page: https://garverselod.github.io/