Gaze-LLE: Gaze Target Estimation via Large-Scale Learned Encoders
Fiona Ryan, Ajay Bati, Sangmin Lee, Daniel Bolya, Judy Hoffman, James M. Rehg
2024-12-13
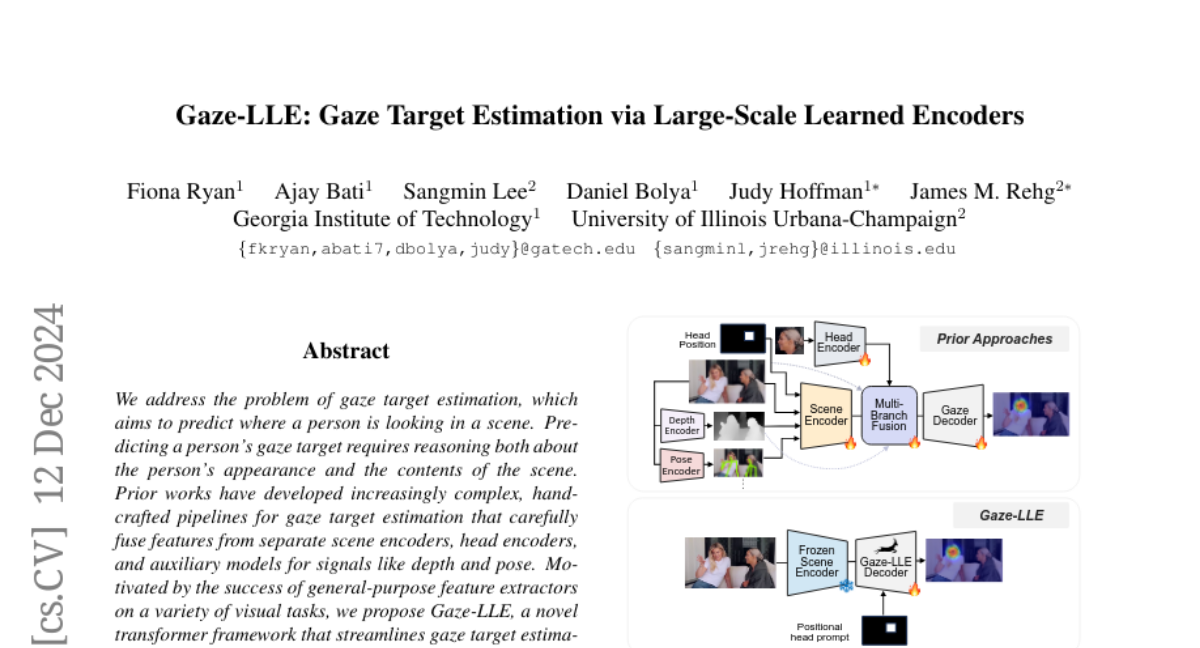
Summary
This paper talks about Gaze-LLE, a new method for predicting where a person is looking in an image using advanced technology that simplifies the process of gaze target estimation.
What's the problem?
Determining where someone is looking in a scene is challenging because it requires understanding both the person's features and the details of the environment. Previous methods used complicated systems that combined information from different sources, which made them hard to manage and less efficient.
What's the solution?
Gaze-LLE introduces a more straightforward approach by using a frozen encoder called DINOv2 to extract visual features from the scene. It then applies a lightweight module to decode these features into a heatmap that shows where the person is likely gazing. This method reduces the complexity of previous models, allowing for faster and more accurate gaze predictions without needing additional inputs like depth or pose information.
Why it matters?
This research is important because it enhances our ability to understand human attention in images, which has applications in areas like virtual reality, robotics, and human-computer interaction. By simplifying the gaze estimation process, Gaze-LLE can lead to better performance in technologies that rely on accurately interpreting where people are looking.
Abstract
We address the problem of gaze target estimation, which aims to predict where a person is looking in a scene. Predicting a person's gaze target requires reasoning both about the person's appearance and the contents of the scene. Prior works have developed increasingly complex, hand-crafted pipelines for gaze target estimation that carefully fuse features from separate scene encoders, head encoders, and auxiliary models for signals like depth and pose. Motivated by the success of general-purpose feature extractors on a variety of visual tasks, we propose Gaze-LLE, a novel transformer framework that streamlines gaze target estimation by leveraging features from a frozen DINOv2 encoder. We extract a single feature representation for the scene, and apply a person-specific positional prompt to decode gaze with a lightweight module. We demonstrate state-of-the-art performance across several gaze benchmarks and provide extensive analysis to validate our design choices. Our code is available at: http://github.com/fkryan/gazelle .